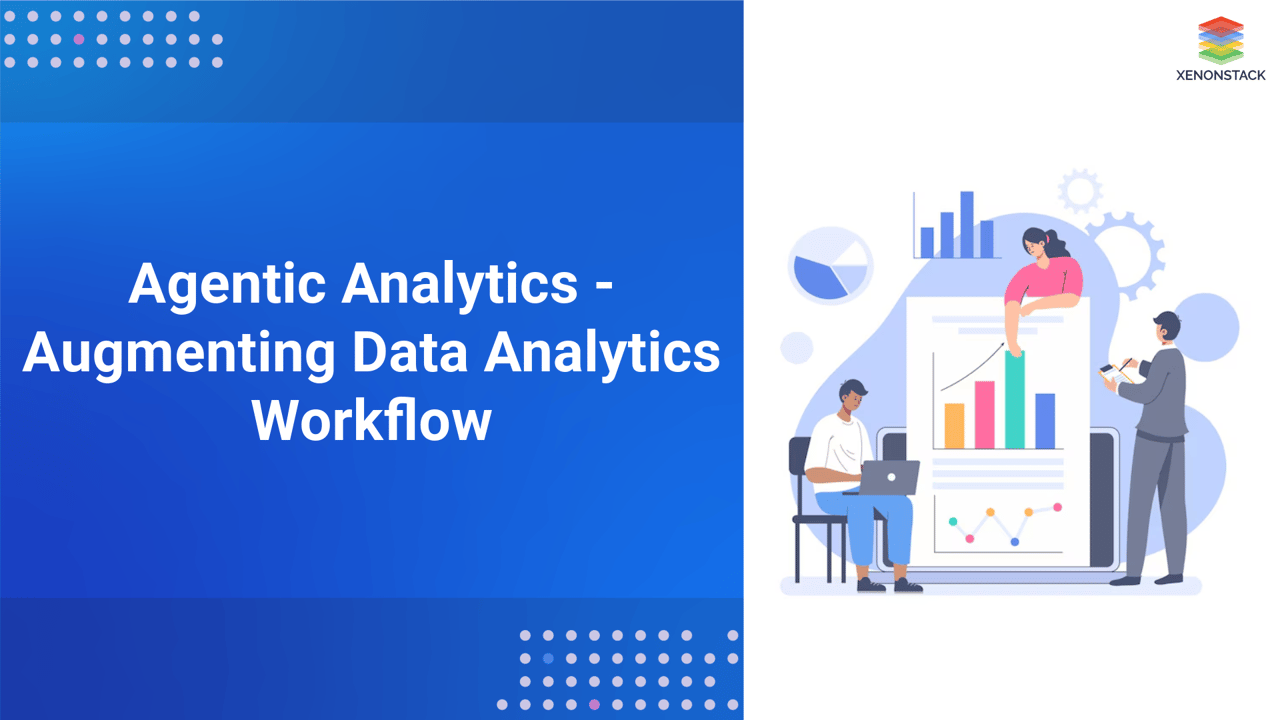
Analytical Processing and Interpretation
Organizations must establish a structured and optimized data workflow to harness Agentic Analytics effectively. The primary stages include:
1. Data Collection and Integration
-
Aggregation of structured (SQL databases) and unstructured (IoT sensor data, social media feeds, video streams) sources.
-
Real-time data ingestion pipelines to support continuous analytics and minimize latency.
-
Cross-platform interoperability between cloud-based, edge, and on-premise infrastructures for seamless data accessibility.
-
Automated data cataloging and metadata management to enhance data discoverability and lineage tracking.
2. Automated Data Cleaning and Preprocessing
-
Implementation of anomaly detection algorithms to enhance data accuracy and integrity.
-
Natural Language Processing (NLP) models for automatic standardization and contextual understanding of textual datasets.
-
Clustering techniques and probabilistic models for outlier detection in numerical and categorical data streams.
-
Automated feature engineering to optimize dataset quality for downstream machine learning applications.
3. Advanced Analytical Processing and Interpretation
-
Deployment of statistical and ML-driven models for multi-dimensional insight extraction.
-
Predictive and prescriptive analytics for scenario modeling, risk mitigation, and business strategy optimization.
-
Edge computing integration for real-time, decentralized data processing and reduced network dependencies.
-
Generative AI models for hypothesis generation and exploratory data analysis.
4. Automated Insight Generation and Reporting
-
Implementing Natural Language Generation (NLG) systems to convert complex analytical findings into human-readable reports.
-
AI explainability frameworks to enhance transparency and trust in decision-making.
-
Real-time alerting systems for dynamic, contextualized recommendations.
-
Integrating AI-generated narratives with business intelligence (BI) dashboards for streamlined decision-making.
5. Continuous Learning and Adaptive Intelligence
-
Iterative model refinement using reinforcement learning to enhance accuracy over time.
-
Real-time adaptation to evolving market trends, consumer behavior patterns, and competitive landscapes.
-
Autonomous self-correction mechanisms that adjust models based on continuous feedback loops and performance monitoring.
-
Multi-agent collaboration where AI agents dynamically communicate and optimize workflows across interconnected systems.
Emerging Trends Shaping Agentic Analytics
Several key technological advancements are propelling the evolution of Agentic Analytics:
-
Augmented Analytics: Democratising data-driven decision-making through AI-assisted, low-code/no-code analytical tools.
-
Real-Time Computational Processing: The advancement of high-speed ML algorithms enabling instantaneous and scalable insight generation.
-
Ethical AI and Data Governance: Ensuring compliance with global data protection regulations (e.g., GDPR, CCPA) while minimizing algorithmic bias.
-
Integration with Next-Gen Technologies: AI-enabled analytics synergizing with blockchain for enhanced data security and IoT for real-time environmental intelligence.
-
Quantum Computing in Analytics: Leveraging quantum algorithms to optimize complex analytical computations beyond classical computing capabilities.
Cross-Industry Applications of Agentic Analytics
Agentic Analytics is revolutionizing multiple industries by enabling autonomous, data-driven decision-making:
-
Financial Services: AI-driven fraud detection, algorithmic trading, automated risk modeling, and credit scoring.
-
Technology and Data Infrastructure: Optimization of data lakes, predictive maintenance for IT assets, and AI-driven network orchestration.
-
Consumer Insights and Marketing: Real-time customer segmentation, hyper-personalized marketing campaigns, sentiment analysis, and customer journey prediction.
-
Healthcare and Life Sciences: AI-powered diagnostics, predictive modeling for disease progression, patient-centric treatment optimization, and medical research acceleration.
-
Manufacturing and Supply Chain: Smart factories leveraging AI for predictive maintenance, supply chain resilience modeling, and automated logistics optimization.
-
Energy and Sustainability: AI-driven energy demand forecasting, climate risk assessment, and carbon footprint reduction strategies.
Challenges and Prospective Developments
Despite its transformative capabilities, the widespread adoption of Agentic Analytics presents significant challenges:
-
Integration Barriers: Compatibility issues with legacy data systems, proprietary infrastructure silos, and outdated data architectures.
-
Ethical and Security Considerations: Addressing AI biases, ensuring data privacy, safeguarding against cyber threats, and adhering to evolving regulatory frameworks.
-
Human-AI Synergy: Balancing autonomous AI execution with human expertise to ensure ethical, strategic, and contextually aware decision-making.
-
Scalability and Computational Demands: Managing large-scale AI-driven analytics' high computational costs and infrastructure demands.
-
Trust and Explainability: Ensuring AI-generated insights remain interpretable, transparent, and aligned with business objectives.
How is Agentic process automation changing data analytics?
Agentic process automation revolutionises data analytics by introducing autonomous, context-aware intelligence into the data lifecycle. Unlike traditional rule-based automation, agentic systems leverage intelligent agents that continuously observe, learn and act without human intervention. These agents streamline data preparation by automatically detecting anomalies, handling missing values, and structuring raw data for analysis. They also enable self-optimizing data pipelines that adapt to real-time volume, schema, or latency changes, ensuring resilience and scalability.
Beyond automation, these systems provide contextual analysis by understanding business goals and correlating internal and external data sources to generate deeper insights. Moreover, agentic AI monitors model performance, detects drift and triggers retraining to maintain accuracy and relevance. Integrating natural language interfaces democratizes access to data, allowing non-technical users to interact with complex datasets effortlessly. This shift accelerates decision-making and enhances precision, productivity, and agility across the analytics ecosystem.
Future Trends in Research and Innovation
Agentic Analytics signifies a groundbreaking shift in data science, fundamentally transforming how AI is leveraged in strategic decision-making. By automating the entire analytics lifecycle—from data acquisition and preprocessing to advanced interpretation and autonomous insight generation—AI agents significantly enhance analytical efficiency, accuracy, and adaptability.
However, successful implementation necessitates robust AI governance frameworks, continuous learning mechanisms, and seamless integration with complementary emerging technologies. As organizations navigate the complexities of an AI-driven era, the strategic adoption of Agentic Analytics will be instrumental in unlocking unprecedented advancements in data intelligence, automation, and innovation.