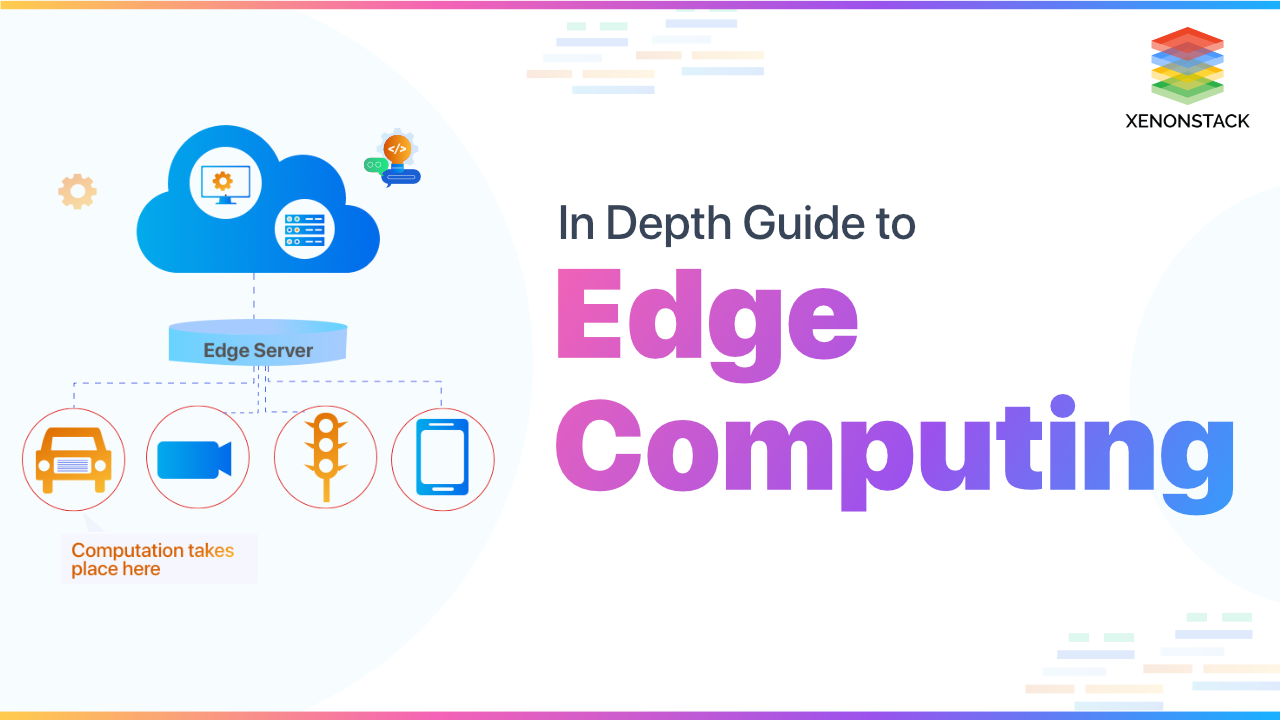
Overview of Edge Computing
It is a distributed computing paradigm in which processing and computation are performed mainly on classified device nodes known as smart devices or edge devices as opposed to processed in a centralized cloud environment or data centers. It helps to provide server resources, data analysis, and artificial intelligence to data collection sources and cyber-physical sources like smart sensors and actuators.Is edge computing seen as necessary? In the realization of physical computing, smart cities, computing, multimedia applications such as augmented reality and cloud gaming, and the Internet of Things (IoT). It is a way to streamline the movement of traffic from IoT devices and implement real-time local data analysis.
Edge AI offers high safety and security level with enhanced security features, and edge AI-powered devices minimize the risk. Source: Bringing Artificial Intelligence
Data produced by the Internet of Things devices is processed where it is created instead of taking away the routes to data centers with the help of it. It also benefits Remote Office/Branch Office (ROBO) environments and organizations that have a dispersed user base geographically. A significant benefit is that it improves time to action and reduces response time to milliseconds while also conserving network resources. “Practice of processing data near the edge of your network locally or on own server produced by IoT instead of centralized data processing warehouse. ”
Benefits of Edge Computing
- Improve Speed/Reduced Latency: As there's almost no distance between the points where data is generated and processed, there is almost negligible latency for Edge. It decreases the time and travel time is known as Latency. Edge computing reduces the latency and increases the network speed. For a company, every millisecond is critical to its success. As a result, latency is measured in microseconds instead of milliseconds.
- Improved Security and Privacy Protection: It improves security and privacy Protection because it keeps data close to the edge. It sends the appropriate amount of data to the cloud. When compared to the cloud, it provides a possibility to reduce risk.
- Cost Saving: The data is now processed and stored in localized servers and devices. There is no need to transfer most data to go to data centers. The ultimate result is that it requires less data center bandwidth. It reduced the cost due to lower bandwidth by storing less data in the cloud.
- Enable AI and ML applications: It allows All the applications to be deployed close to where data is collected, so results are often obtained in a real-time environment.
Various types of Edge Computing
There are four types of Edge Computing.
- Cloud: Cloud computing is the first type of it refers to the massive data centers run by cloud companies like AWS and GCP, which can include VMware Cloud on AWS and other clouds.
- Device Edge: The 2nd type of it is the Device edge. It is also known as Nano DC. It has limited processing power.
- Compute Edge: The 3rd type of it is the Compute Edge. It is also called Micro-DC. Compute Edge is a small data center consisting of many servers. In these data centers, you will have a lot of resources. One significant advantage is that the system latency at the edge would be less relative to the cloud. So network bandwidth is higher but more efficient.
- Sensors: The fourth type of it is Sensors. IoT Sensors collect data and monitor things. Examples of sensors are light bulbs, clocks, Surveillance cameras, etc.
Key Features of Edge Computing
- A feature that will play an essential role in the expansion of it.
- It is perfect for low latency, where every millisecond counts.
- It is suitable for fast and real-time analysis.
- It can work without internet connectivity.
- By reducing latency.
- By reducing latency edge, computing improves network performance.
- Fewer latency and bandwidth requirements increased performance, highly scalable, and lower operational expenses.
Internet of Things and Edge Computing
With IoT, and with its help of it, intelligence moves to the edge. If you have massive amounts of data and for this, you have to leverage in such end-to-endways or highly sensor-intensive or data-intensive environments where data is generated at the edge, which is due to IoT as data sensing at the edge. And also, with real-time information, the increasingly unstructured data of which sensor and IoT data are part, traditional approaches don’t meet the requirements which are needed. There are various scenarios where speed and high-speed data are the main components for management, power issues, analytics, real-time need, etc., which helps to process data with it in the Internet of Things.Internet of Things Critical Cases
- Network bandwidth limitations and cut costs.
- Evolution of Cloud Computing.
- Data Center Loads and Complementing.
- Security Concerns.
What are the benefits of Edge Computing in IoT?
- Lesser Network Load
- Zero Latency
- Reduced Data Exposure
- Computational Efficient
- Costs and Autonomous Operation
- Security and Privacy
Future Directions of Computing for the Internet of Things
- Edge-to-Cloud data exchange capabilities
- Common-on-Edge data exchange capabilities
- Streaming Data Analytics and Batch frameworks and APIs
- Controlled rolling and Versioning upgrades of applications
- Status of application monitoring from an Ad-Hoc Cloud Dashboard
- Cloud-Based Deployments of its Applications
Terms and Definitions
The terms related to it are defined:
Edge
It highly depends on the use cases. Like in telecommunication, it may be a cell phone or cell tower. Similarly, in the automotive example, it could be a car. In manufacturing, it could be a machine, and in the Information Technology field, it could be a laptop.Edge Devices
A device that produces data is an edge device like a machine and sensor or any device through which information is collected and delivered.Edge Gateway
It’s a buffer where its processing is done. The gateway is the window into the environment beyond the edge of the network.Fat Client
It’s software that processes data in edge devices, which is opposite to thin client, which hardly transfers data.Equipment
Devices like sensors and machines can be outfitted to work in it. Environments by making the internet accessible.Mobile
It signifies the growth of its systems in telecommunication systems like 5G scenarios.What exactly is it, according to research firms?
- A network of micro data centers that store or process critical data locally and push received data to a centralized data center or repository of cloud storage.
- Typically in the Internet of Things use cases, a massive chunk of data goes through the data center, but it processes the data locally, resulting in reduced traffic in the central repository.
- This is done by devices transferring the data to the local device, which includes storage, computing, and network connectivity.
- After that, data is processed at the edge while another portion is sent to the storage repository or central processing in the data center.
The edge is an endpoint where data is generated through some type of interface, device, or sensor. The edge has become a major growth business. Source: The Edge- What Does It Mean For Artificial Intelligence?
Why is Edge Computing in IoT important?
- New Functionalities are offered.
- Easier configurations.
- Hacking Potential is increased.
- The load on the server is reduced.
- Load on Network is reduced.
- Application Programming Interface.
- Increases Extensibility.
- Centralized Management.
- Costs of Licensing.
- Support and Updates.
Advantages of enabling it
- Increase Speed.
- Rise in Reliability.
- Decrease the random issues.
- The compliance issue is reduced.
- Minimize the Hacking issues.
- Decrease the random issues.
Edge Gateway Server
- Real-Time Analytics
- Transactional analytics
- Business Intelligence
- No Latency Issue
- Medium Latency Requirements
- Low Latency Requirements
Cloud Computing vs. Edge Computing vs. Fog Computing
Fog Computing is the extension of Cloud Networks, which are a collection of servers comprising a distributed network. Such networks allow organizations to exceed the resources that would be otherwise available to them. The main advantage of cloud networks is that they allow data collection from multiple sources. Which is accessible anywhere over the internet. While Fog Computing and it are almost similar, where the talk about intelligence and processing of data at the time of creation.
However, there is a crucial difference between these two in terms of intelligence and computing power; where Fog Computing focus more on intelligence at the local area network, and this architecture transmits data from endpoints to a gateway where it is sent to sources for processing and return to the transmission while it focuses more on computing power and processing of data locally at the edge of a network. It performs processing on embedded computing platforms interfacing with sensors and controllers.
Connect to deal with persistent data near the data source, which is considered the 'edge' of the association. Click to explore about, Difference Between Cloud, Edge, and Fog Computing?
Cloud Layer
- Big Data Processing
- Data Warehousing
- Business Logic
Fog Layer
- Local Network
- Data Analysis and Reduction
- Standardization
Edge Layer
- Large Volume Real-Time Data Processing
- On-premises Data Visualization
- Embedded Systems
- Gateways
- Micro Data Storage
Laws Calling
- The first one is the law of physics, where you require latency sometimes, which is short, like triggering an alert and needing to react instantly, having no time to get back to the cloud, and for that, you need to able to act on data locally.
- The second one is the law of economics, where you want to process data without much cost and traffic, so it helps to process data locally at the edge of the network.
- The last one is the law of the land, where a business might have particular requirements where certain data needs to stay local, like regulation.
Security
There are two sides to security in it -- One of them is that the security in it is better than any other part of the data storage application because data is not traveling over the network.
- The flip side of it is that security in it is less secure because the edge devices in themselves can be more vulnerable.
- In conclusion, data encryption, access control, and the use of virtual private networks are crucial elements to protect the system.
Use Cases of Edge Computing
- Having low latency, e.g., Closed-loop interaction between machine insights.
- For real-time analytics, access to temporal data.
- Low connectivity, e.g., Remote Location.
- The high cost of transferring data to the cloud.
- Bandwidth.
- Cybersecurity constraints.
- Compliance and Regulation.
- The immediacy of Analysis, e.g., To check machine performance.
- Predictive Maintenance.
- Energy Efficiency Management.
- Flexible Device Replacement.
Why it matters?
- When IoT devices have poor connectivity.
- Not efficient for Internet of Things devices to be in constant touch with the central cloud.
- The latency factor reduces latency because data doesn’t have to traverse over a network to a central cloud for processing.
- Where latencies are untenable, like manufacturing or financial services.
- As soon as data is produced, it doesn’t need to send over a network; instead, it compiles the data and sends daily reports to the cloud for long-term storage, i.e., reduces the data traversing.
- The buildout of the next-generation 5G cellular networks by telecommunication companies.
- Direct access to the gateway into the telecom provider’s network, which connects to a public IaaS cloud provider.
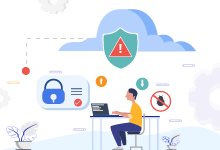
Uses in Industries
- Smart applications and devices respond to data, instantly eliminating lag time.
- Real-time data process with any latency where even milliseconds in latency make a difference in the processing of data.
- Acceleration in the data stream.
- Efficient data processing in massive data.
- Effective use of the application in a remote location.
- Security for sensitive data even without putting it in the public cloud.
Role of it in Healthcare
As we know, edge allows us to manage your connectivity and disperse processing closer to where data is, the advantage is a natural evolution when you optimize some part of your stack in the network by giving more localized services for your application. Moving the analysis of clinical information to it is crucial for healthcare organizations that want to benefit from going digital and the key to digital healthcare Analytics. For example, in the hospital, we collect data from IoT devices, which monitor patients and transfer it to the trust’s electronic health record (EHR) from the bedside, with the authentication of staff to the Internet of Things devices through proximity cards.Role of it in Social Good
Environmental factors like road traffic density, air quality, weather, school holidays, and other open data sets give better results by the processing of data with the help of it and machine learning. The computing power will apply these factors to the data collected from healthcare at the point of admission, where data is to be set where the patient is expected to be discharged. There is also a movement from businesses in all sectors to use it.
What is the future of edge computing?
Its future of it will improve networks like 5G. It provides more capacity and Power. It is taking the future by increasing the speed. Whether it's the need for short response time and low latency to make computing power portable enough to place it at the edge of the network where data is generated, with lowering manufacturing costs and availability of better network and bandwidth, it is rapidly reaching far and wide. The future of it is quickly increasing day by day. As processors become more powerful, storage becomes cheaper, and network access improves in it, there are a number of opportunities to change the world.
A Holistic Strategy
Learning more about edge computing has created an opportunity to help enterprises turn massive amounts of machine-based data into actionable insights with Edgeline IoT Systems, Mobility, and Workplace Services, and Internet of Things Solutions. We recommend talking to our expert.
- Discover more about Artificial Intelligence in Edge Computing
- Discover more about EdgeOps Platform Benefits and Working