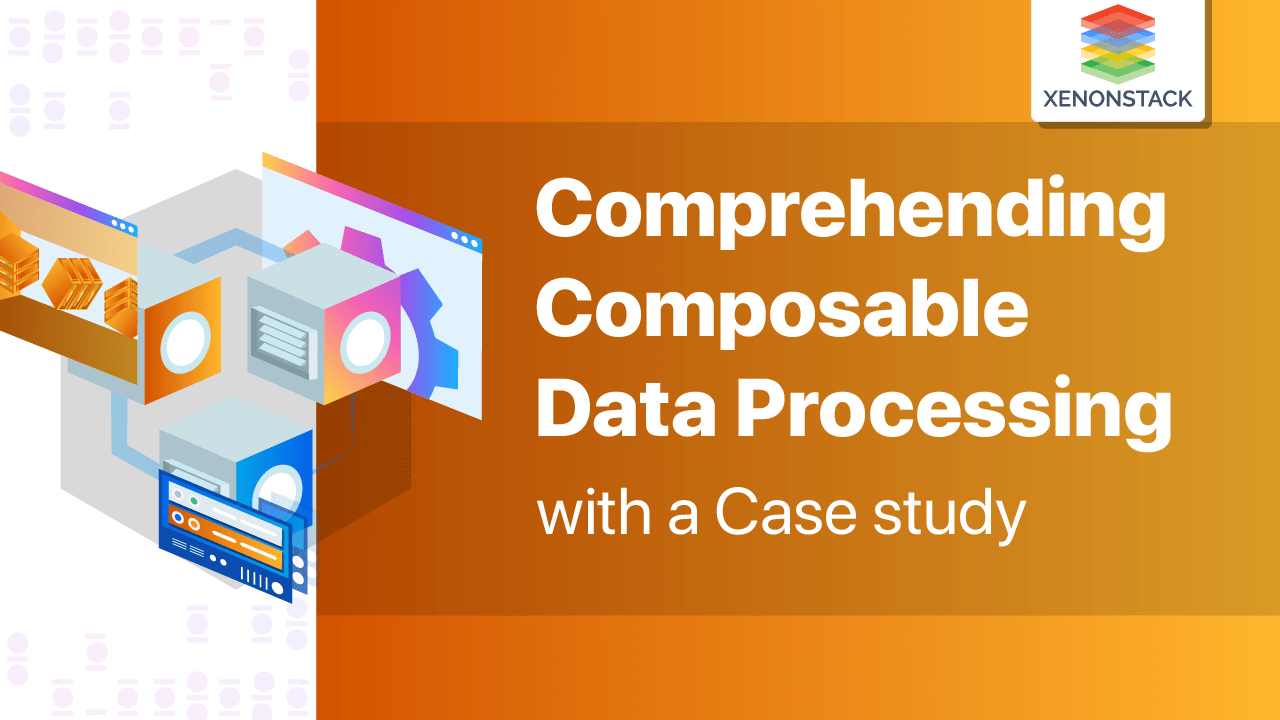
What is Composable Data Processing?
This is a framework that enables different teams to extend data ingestion of Siphon, as shown in Figure. It is:
-
Efficient
-
Modular
-
Plugable
-
Composable
-
Supportable
By having a single stage, a single pass through the data, and a single output, it ends up with the least amount of complexity, latency, and cost, as shown in Figure 1.7.
Composable Data Processing allows scale engineering effort, modularizes architecture and code, and clearly distinguishes roles. Make it easy to test and maintain the system and maximize reuse.
Why do we need Composable Data Processing?
The organization's decision to become data-driven has resulted in a change in the technologies and approaches used to work with data. When data becomes more complicated, the need to process it before using it in analytics solutions becomes more difficult. In a single day, they must upload and use terabytes of data. As a result, collecting and transferring data becomes time-consuming, causing solutions or applications to accumulate backlogs.
Composable Data Processing is an approach that can be used to overcome this solution. To better understand why we need composable data processing, let's discuss a case study of AEP (Adobe Experience Platform) that includes an introduction to composable data processing, the need for it, and the benefits it offers.
Case Study to Understand Composable Data Processing
Adobe Experience Platform (AEP) uses different types of data such as personal, geographical, events, etc., as shown in Figure 1.1. After that, the data governance to manage and use the data followed ML to enrich the data and get insights. Finally, AEP provides an application experience for the customer actions, such as providing targeted ad campaigns.
They use certain applications, services, and a platform to accomplish this mission, as shown.
Adobe team is working in both parts of the data ingest team. As shown in Figure 1.3, ingesting data to data platforms (Siphon). And then distribute chunks of data (data batches) to the consumers.
It's not only about transferring data; several other tasks must be completed as well. Data transformations, planning, and formatting are also essential.
With all of the issues discussed in Figure 1.3, they have to pass 1 million batches every day, which equates to approximately 13 Terabytes, or 34 billion events.
They focus on cross-cutting features of big data applications for workload management, scaling, monitoring, and resilience. Such as queuing and throttling to tackle spikes in workload and scaling based on backlogs.
However, with the change in requirements, new challenges are emerging. Such as demands to have more features as;
-
Parse: The ability to support additional logs and more types of formats rather than JSAL, Mackay, and CSV
-
Convert: To convert raw data into XTL(file format), they start buying additional ETL (Extract Transform Load) transformation capabilities.
-
Validate: Validation tasks such as format check, context check, and check range according to the data.
-
Report: Demands for the additional reporting and diagnostic check tools.
-
Write Data: To use new and advanced technologies for writing and storing data.
-
Write Errors: Monitoring, merging, and mining capabilities, as well as auto-correcting functionality for incorrect or poor data in the system.
With all of these various challenges, they might have different groups of individuals, or different teams, working on each of them. Unfortunately, these data processing features are only being worked on by one team, Siphon.
They will have to take away the challenging work they are doing there if they transfer the team to data processing tasks. Unfortunately, demands are from both ends to scale. The problem is figuring out how to expand the number of data processing features without jeopardizing the system's stability.
A data catalog is a list of all the data that an entity has. It is a library where data is indexed, organized, and stored for an entity. Read more about Data Catalog Architecture for Enterprise Data Assets
Benefits of Composable Data Processing
It provides various benefits to the Siphon, Platform, and customers. As shown in Figure 1.9.
Siphon
-
Scalable Engineering
-
Separation of Responsibilities
-
More reliable and Testable code
-
Easy to maintain
Platform
-
50% or more storage-saving
-
50% or more compute saving
-
Reuse
Customers
-
Minimize Latency
-
More error reporting features
-
More validation features
-
More ETL features
Data Observability abolishes the need for debugging in a respective deployment environment by monitoring the performance of applications. Click to explore What is Data Observability?
Conclusion
The need for composable data processing is already discussed with the case study. The volume of data and heterogeneity of data sources make the data processing operations complex and time-consuming as it requires a lot of tasks for transformation, validation, and process.
Composable Data processing provides a more generic infrastructure or way to integrate different processing steps from other teams, systems, or organizations. That reduces the complexity of the data processing team's task and allows them to work on terabytes of data daily.
- Read more about Top 9 Challenges of Big Data Architecture
- Discover more about Data Catalog with Data Discovery