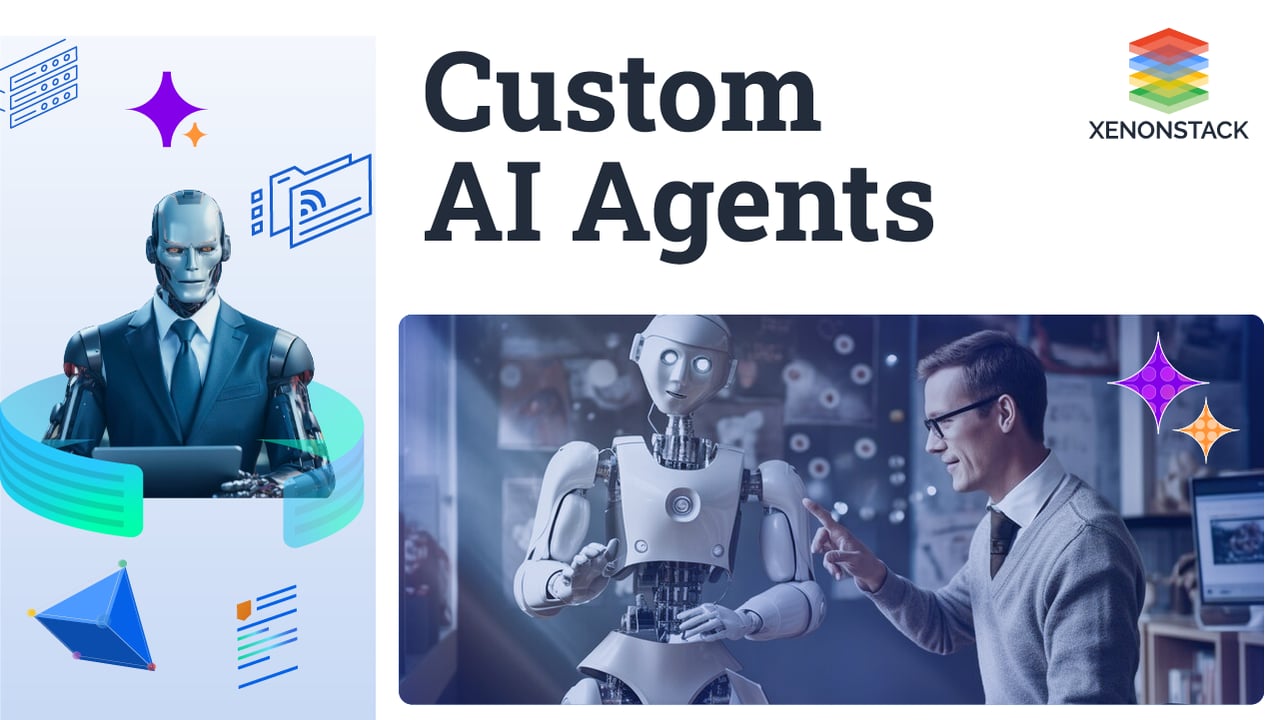
How to Build a Custom AI Agent?
AI agents are transforming industries by automating tasks, analyzing data, and making intelligent decisions. Whether for customer support, data analytics, or real-time decision-making, building a custom AI agent requires a strategic approach. This guide walks you through the essential steps to develop a robust AI agent tailored to your needs.
Step 1 - Define the Objectives
Before development begins, clearly define your AI agent’s purpose and tasks. Will it provide personalized recommendations, automate workflows, or assist users with queries? Setting well-defined objectives helps guide architecture and functionality decisions.
Step 2 - Gather and Prepare Data
Quality data is the foundation of an effective AI agent. Collect relevant datasets such as text, images, audio, or structured information, depending on your agent’s use case. Ensure diversity and accuracy for improved training outcomes.
Step 3 - Choose the Right LLM and AI Technologies
Not all large language models (LLMs) are equal. Selecting the right AI tools ensures optimal performance:
-
Choose an LLM that excels in reasoning benchmarks.
-
Support chain-of-thought (CoT) prompting for better decision-making.
-
Ensure consistent and reliable outputs.
Tip: Experiment with different models and fine-tune prompts to enhance reasoning capabilities.
Step 4 - Design the AI Agent’s Control Logic
Your AI agent must operate based on defined logic:
-
Tool Use: Calls external tools only when necessary.
-
Basic Reflection: Generates, critiques, and refines responses.
-
ReAct (Reasoning + Acting): Plans, executes, observes, and iterates.
-
Plan-then-Execute: Structures all steps before execution.
Choosing the right approach improves the agent’s reasoning and reliability.
Step 5 - Define Core Instructions & Features
Set operational rules to guide the AI agent’s interactions:
-
How should it handle unclear queries? (Ask clarifying questions.)
-
When should it use external tools?
-
What output formats should it follow? (Markdown, JSON, etc.)
-
What should its interaction style be? (Formal, conversational, structured.)
The clear system prompts help shape predictable and structured behaviour.
Step 6 - Implement a Memory Strategy
LLMs don’t inherently remember past interactions, so implementing a memory strategy is crucial:
-
Sliding Window: Retains recent exchanges while discarding older ones.
-
Summarized Memory: Condenses key takeaways from conversations.
-
Long-Term Memory: Stores user preferences for personalized responses.
Example: A financial AI agent recalls a user’s risk tolerance from previous interactions.
Step 7 - Equip the AI Agent with Tools & APIs
To expand its capabilities, integrate external tools:
-
Name: Clearly defined and intuitive (e.g., “StockPriceRetriever”).
-
Description: Explains functionality and expected behavior.
-
Schemas: Defines structured input/output formats.
-
Error Handling: Manages tool failures effectively.
Example: A customer support AI retrieves order details from a CRM API.
Step 8 - Define the AI Agent’s Role & Key Tasks
A well-defined role enhances efficiency. Clearly state:
-
Mission: (e.g., “I analyze financial data to generate insights.”)
-
Key Tasks: (Summarizing, visualizing, analyzing.)
-
Limitations: (e.g., “I do not provide legal advice.”)
Example: A finance-focused AI sticks to financial insights and avoids unrelated topics.
Step 9 - Handling Raw LLM Outputs
Post-processing ensures structured, accurate responses:
-
Convert AI output into structured formats (JSON, tables, charts).
-
Validate correctness before delivering responses.
-
Ensure proper execution of external tools.
Example: A financial AI extracts data and formats it into a structured JSON file.
Step 10 - Scaling to Multi-Agent Systems (Advanced)
For complex workflows, multiple AI agents can collaborate. Consider:
-
Information Sharing: Define how agents communicate and share context.
-
Error Handling: Plan for failures and fallback strategies.
-
State Management: Enable task pausing and resumption.
Example: One agent fetches raw data, Another agent summarizes it, A third generates a final report
Integrate, Deploy, and Continuously Improve
Once built, integrate your AI agent into its target environment, ensuring compatibility with existing systems and user interfaces. Monitor its performance, gather user feedback, and refine it through iterative improvements to enhance efficiency and reliability.
By following these steps, you can build AI agents that are intelligent, efficient, and adaptable for various business applications.
Best Practices for Building Custom AI Agents
-
User-Centric Design: Emphasize user experience and usability at every stage of development, ensuring the AI agent fulfills the needs and expectations of its target audience.
-
Ethical Considerations: Embed ethical principles such as fairness, transparency, and accountability into the design and operation of the AI agent, mitigating risks associated with bias, discrimination, and privacy infringement.
-
Collaboration and Interdisciplinary Expertise: Foster collaboration between domain experts, data scientists, engineers, and other stakeholders to leverage diverse perspectives and expertise in building custom AI agents that deliver value and impact.
-
Scalability and Flexibility: Design AI architectures and systems that can scale gracefully to accommodate growing data volumes, user demands, and evolving use cases, future-proofing your investments and efforts.
-
Security and Compliance: Implement robust security measures and adhere to regulatory requirements to protect sensitive data, mitigate cybersecurity risks, and build trust with users and stakeholders.
Use cases of Custom AI Agents
Custom AI agents offer lots of applications across different business sectors, promising to revolutionize operations and workflow. Let's explore some of the key areas where Custom AI agents can make a significant impact.
-
HR Automation: In human resources, AI agents streamline recruitment and onboarding processes, handling tasks like application sorting and interview scheduling. They also serve as knowledge hubs for new employees, providing instant answers to common queries.
-
Project Management: AI agents streamline task allocation and progress tracking in project management. They distribute tasks based on team members' skills and availability, providing real-time updates and predictive analytics for proactive adjustments.
-
Business Management Automation: AI agents act as decision support systems in business management, offering insights derived from data analysis. They aid in strategic decision-making by analyzing market trends, operational data, and customer insights.
-
Customer Support Automation: AI agents enhance customer support by addressing inquiries, solving technical issues, and offering personalized recommendations. They analyze customer interactions to personalize communication and build trust and loyalty.
-
Finance Automation: AI agents automate back-office tasks in finance, including organizing financial records, processing invoices, and predicting future budget needs. They also validate financial transactions to ensure security and reliability.
-
Document Management: AI agents automate document sorting and archiving, improving organizational efficiency and data retrieval. They can also generate various business documents based on provided data inputs.
-
Order Management: AI agents automate the handling of customer orders, from data extraction to order processing. They provide automated updates to customers about their orders, enhancing the customer experience and reducing the workload on customer service teams.
-
Supply Chain: AI agents integrate and analyze data across the supply chain, allowing for more accurate forecasting of supply chain needs. They also enhance logistics operations by automating shipment tracking and suggesting alternative routes or methods.
Within each domain, Custom AI agents provide efficiency, precision, and automation, empowering businesses to optimize operations and adeptly respond to evolving demands.
Explore how Akira AI Agents enable autonomous operations
Benefits of Building Your Own AI Agent
Building your own AI agent comes with a multitude of benefits that cater to both practical and personal aspects
-
Customization: One notable benefit is the capability to tailor the AI agent to align with your precise requirements and preferences. You have full control over its features, functionalities, and interactions, ensuring it aligns perfectly with your requirements. Whether it's for automating tasks, providing personalized recommendations, or engaging in conversations, the AI agent can be tailored precisely to your liking.
-
Efficiency and Effectiveness: By building your own AI agent, you can design it to be highly efficient and effective in performing tasks. Since it's tailored to your needs, it can streamline processes, automate repetitive tasks, and provide targeted assistance. As a result, productivity is heightened, and performance across different facets of life or work is enhanced.
-
Understanding and Skill Development: The process of building an AI agent provides invaluable learning opportunities. You gain hands-on experience in AI development, learning about different algorithms, technologies, and methodologies along the way. This enriches your comprehension of artificial intelligence and provides you with invaluable skills that are highly sought-after in today's technology-driven world.
-
Independence and Control: Unlike relying on pre-built AI solutions, building your own AI agent gives you complete independence and control over its operation. You're not bound by the limitations or constraints of third-party platforms, allowing you to customize and adapt the agent according to changing requirements or preferences. This independence ensures flexibility and autonomy in how you utilize the AI agent.
-
Innovation and Creativity: Building your own AI agent encourages innovation and creativity. You are empowered to delve into innovative concepts, explore diverse methodologies, and push the limits of what AI technology can achieve. This fosters a culture of innovation, empowering you to develop unique solutions and applications that cater to specific challenges or opportunities.
-
Personalized Interaction: Your AI agent learns from you over time, adapting to your preferences and becoming more helpful and relatable as you interact with them.
-
Empowerment Through Learning: Building your AI agent is not just about the result; it's also a learning experience that can empower you to understand and engage with AI technology on a deeper level.
Explore more about Automate Complex Tasks with Autonomous Agents Learn more about Autonomous Customer Agents
Drawbacks of Building Your AI Agent
-
Time and Effort: Building a custom AI agent requires time, effort, and sometimes technical knowledge, which may not be feasible for everyone.
-
Complexity: Depending on the complexity of your agent's tasks and interactions, the development process can be challenging and may require troubleshooting along the way.
-
Limitations in Functionality: While you can mostly customize your AI agent, its capabilities may still be limited compared to more advanced commercial solutions.
Custom AI Agents vs. Autonomous Agents
Custom AI Agents
Custom AI agents are software programs specifically tailored to carry out predefined tasks. These agents are designed around a business’s unique requirements, workflows, and existing systems. They can handle various applications, from automating customer interactions in a chatbot to handling internal workflows like processing tickets or scheduling resources.
Key characteristics:
-
Task-specific behaviour
-
Rule-based or ML model-driven logic
-
Deterministic outcomes, depending on the design
-
Aligned tightly with organizational workflows
-
Designed to function within known boundaries and pre-configured datasets
-
Ideal for operational teams with rigid workflows and predictable patterns
These agents are often deployed in customer service, HR onboarding processes, or IT helpdesk workflows, where consistency, accuracy, and transparency are critical.
Autonomous AI Agents
Autonomous agents go a step further. They are designed to perceive their environment, reason over inputs, make independent decisions and take actions—without constant human intervention. These agents are ideal for dynamic conditions like supply chain management, IT operations, and adaptive customer service.
Key characteristics:
-
Goal-oriented and self-directed
-
Operate in real-time, adapting to environmental changes
-
Use feedback to improve over time
-
Often built using advanced AI techniques like reinforcement learning, planning algorithms, and LLMs
-
Capable of complex decision-making involving uncertainty, trade-offs, and exploration
-
Ideal for rapidly changing, data-rich environments requiring continuous decision optimization
In practice, custom agents provide fine-tuned, controlled behaviour for specific problems, while autonomous agents offer broader, adaptive solutions for environments requiring flexibility and decision-making.
The Future of Agentic Operations
-
AgentOps: The emerging field of agent operations focuses on the deployment, monitoring, and lifecycle management of AI agents in production.
-
Autonomous Business Units: Envision AI-driven teams managing logistics, marketing, or IT independently with minimal human supervision.
-
Collaborative AI Swarms: Inspired by nature, this approach allows thousands of simple agents to solve complex problems together.
-
Continuous Self-Learning: Agents will be able to learn not just from data but from simulated environments and operational outcomes.
-
Digital Twins and Simulation Agents: Use AI to simulate real-world operations and predict outcomes across supply chains or infrastructure.
-
Hyper-Personalized Agents: Agents will increasingly understand individual user behaviour, preferences, and emotions for tailored interactions.