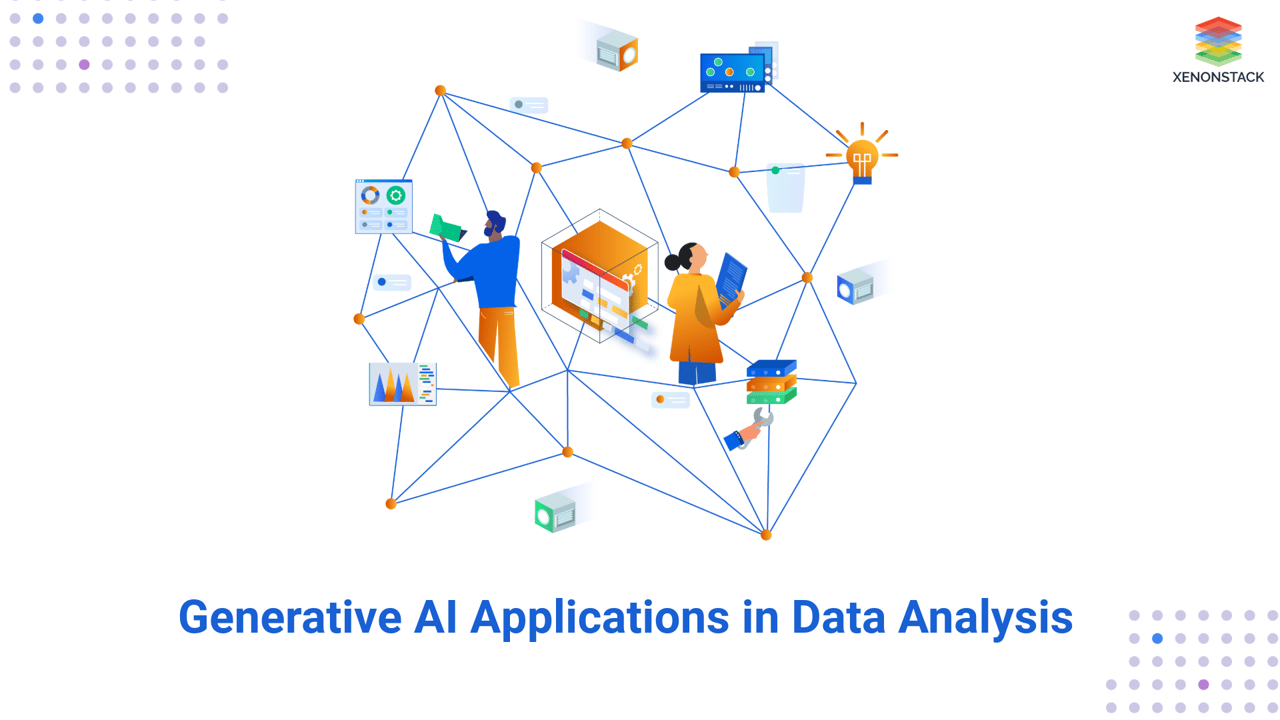
Advantages of Generative AI for Data Analysis
Generative AI in Data Democratization, turning vast amounts of synthetic data into actionable insights. As big data continues to play a critical role in business application strategy, AI becomes embedded in the sense-making process of enterprises. The future is bright, and Generative AI is at the forefront of this transformative journey. Here’s what it offers:
1. Automated Insights: Traditionally, data analysis required skilled analysts to meticulously sift through datasets. Generative AI algorithms automate this process, swiftly identifying crucial indicators and patterns. Decision-makers can now access real-time information without delay.
2. Efficiency Boost: Repetitive tasks like data cleaning and organization are automated by Generative AI. Analysts can redirect their efforts toward building advanced models and scrutinizing results. This efficiency enhancement accelerates the analytical process.
3. Understanding Customer Behavior: Generative AI delves into unstructured data, such as social media posts and online reviews. By analyzing copious amounts of text, it provides a deeper understanding of customer behavior. Companies can leverage these insights to craft targeted marketing strategies and enhance overall customer experiences.
How can generative AI improve predictive analytics in different industries
Data Synthesis and Augmentation
Generative AI creates synthetic data to enhance limited or sensitive datasets, improving model accuracy, especially in fields like healthcare where it can supplement small patient datasets.
Scenario Simulation
GenAI simulates various future scenarios for "what-if" analyses, aiding industries like finance and automotive in assessing risks and testing systems under rare conditions.
Anomaly Detection
By learning normal data patterns, generative models detect anomalies, helping industries like cybersecurity and fraud prevention identify risks early.
Enhanced Time-Series Forecasting
Generative AI leverages techniques like RNNs and GANs to predict future trends from historical data, improving accuracy in areas like stock prices, energy demand, and weather.
Natural Language Generation (NLG)
NLG enables AI to generate human-like reports and summaries from complex data, simplifying communication of trends and forecasts.
Personalization and Recommendations
Generative AI analyzes user behavior to provide personalized recommendations, boosting engagement in e-commerce and content streaming.
Risk Assessment and Management
Generative models simulate crisis scenarios, helping organizations anticipate and manage potential risks more effectively.
Improved Data Quality and Preparation
GenAI enhances data quality by accurately filling in missing values, ensuring more reliable datasets for decision-making.
Utilizing Generative AI in Data Lifecycle Management
Data lifecycle management involves the process of managing data throughout its entire lifespan, from creation or acquisition to disposal. The data lifecycle typically consists of several phases, and the specific steps may vary depending on your organization and data type. There are various steps in which Generative AI can be applied:
1. Data Extraction
Web Scraping
LLMs excel in web scraping and extracting text, links, and images from web pages. They understand text meaning, identify patterns, and summarize information. Extracted data is then pre-processed for further analysis.
Genetic algorithms optimize web scraping by evolving parameters, handling dynamic content, circumventing anti-scraping measures, optimizing data extraction, and adapting to website changes.
Schema Inference & Data Parsing
Generative AI is used in inferring data schemas and parsing unstructured or semi-structured data. Trained on sample data, models learn patterns and extract structured elements, facilitating the transformation of raw data into a structured format.
Gen AI helps enhance schema inference and data parsing by iteratively optimizing algorithms to infer data structures accurately, handle diverse data formats efficiently, and adapt to changes in schema and data patterns dynamically.
Transactional Data Extraction
LLMs extract data from articles, documents, and data marketplaces, saving it in an appropriate format within the Enterprise Data Platform. For instance, extracting financial data from reports, summarizing it, and generating starter code for export to JSON format. They also extract transactional data from documents like invoices and receipts in various text formats, including PDFs.
This can be optimized by Gen AI with streamlining transactional data extraction by iteratively optimizing extraction algorithms to accurately capture transaction details from various sources, improving efficiency, accuracy, and adaptability to changing data formats and structures.
2. Data Integration
Schema Mapping and Transformation
Generative models, trained on source and target data schemas, create mapping rules and transformations. This simplifies data integration, ensures schematic alignment, and provides audit reference documents.
The data integration with gen AI can refine schema mapping and transformation processes by iteratively optimizing algorithms to accurately map data between different schemas, enhancing efficiency, accuracy, and adaptability to evolving data structures and transformation requirements
Entity Resolution and Matching
Generative AI is used in entity resolution and matching tasks, identifying and linking entities across diverse datasets.
This is improved by entity resolution and matching by iteratively optimizing algorithms to accurately identify and match entities across datasets, enhancing efficiency, accuracy, and adaptability to varying data quality and matching criteria.
Data Unification and Deduplication
Trained on existing data, generative models learn patterns to identify duplicate records, generating rules and algorithms for merging similar records. This streamlines data integration by eliminating duplicates.
3. Data Transformation
Data Cleansing
LLM identifies and corrects anomalies within datasets, assisting in standardizing formats and performing deduplication tasks.
By using Gen AI for data analysis enhances data cleansing by iteratively optimizing algorithms to automatically detect and correct errors, remove duplicates, and standardize data formats, improving data quality, accuracy, and efficiency in data processing pipelines.
Data Mapping and Transformation
Generative AI, trained on source and target data schemas, creates mappings and transformation rules. LLMs generate code for tasks like merging, formatting or filtering data.
For example, LLMs can transform data across the medallion data flow pattern (Bronze, Silver, Gold), refining and aggregating to generate reports on Sales, Marketing, and Supply Chain/Logistics. LLMs also aid data analysts by quickly validating hypotheses and generating framework code for data transformation rules when generating reports.
4. Data Discovery and Exploration
Data Profiling
Generative AI analyzes dataset content, structure, and metadata, generating descriptive summaries, statistics, and visual representations like distribution charts.
Data profiling with Gen AI can be done via iteratively optimizing algorithms to accurately analyze and summarize data characteristics, identifying patterns, anomalies, and relationships within datasets, enhancing insights, efficiency, and adaptability to diverse data structures and domains.
Data Clustering and Classification
Generative models scrutinize features and relationships to identify groups or categories and help segment datasets.
It can be done from GenAI by iteratively optimizing algorithms to accurately group similar data points and assign them to relevant categories or classes, enhancing efficiency, accuracy, and adaptability to varying data distributions and complexities.
Exploratory Data Visualization
Generative AI supports exploratory data visualization by generating diverse visual formats, helping users interactively explore patterns, trends, and relationships. It creates representations like network graphs or relationship maps for uncovering data dependencies.
Anomaly/Outlier Detection
Generative AI models assist in detecting anomalies or outliers in datasets, flagging potential issues for further investigation during the data discovery process.
Gen AI enhances anomaly/outlier detection by iteratively optimizing algorithms to accurately identify deviations from normal patterns in data, improving detection sensitivity, accuracy, and adaptability to diverse data distributions and anomaly types.
Conversational, natural language interfaces leverage Generative AI to create user-friendly interfaces for data discovery. They interpret user queries, retrieve relevant data, and provide insights in a conversational manner.
5. Data Quality
Data Quality Assessment
Generative AI analyzes data patterns and distributions and identifies anomalies, outliers, and potential quality issues. It flags erroneous, incomplete, and missing data for data cleaning.
Data Preprocessing
Generative AI automates preprocessing tasks like missing value imputation and feature scaling. It predicts missing values and applies standardization techniques for data consistency and quality.
Data Synthesis and Augmentation
Generative AI aids in generating synthetic data points mirroring the patterns of the original dataset. This enhances data for further exploration and hypothesis validation.
6. Data Orchestration: Workflow Automation and DataOps
Generative AI is revolutionizing data orchestration by automating critical tasks throughout the data lifecycle and DataOps. Let's explore how it enhances workflow automation:
Workflow Generation and Documentation
Generative models, trained on historical data and workflow patterns, can automatically generate workflow templates. These templates capture data dependencies, task sequences, and operational procedures. By documenting these details, organizations ensure efficient and auditable workflows.
Task Scheduling Optimization
Generative AI assists in optimal task scheduling within data orchestration workflows. By analyzing dependencies, resource constraints, and historical performance data, models recommend efficient task execution sequences. This optimization minimizes resource bottlenecks and ensures timely data processing.
Debugging and Error Handling
Generative models analyze error logs and historical data to identify common errors. Recommendations for handling and recovering from failures are generated. For instance, Large-Scale Language Models (LLMs) can inspect and debug pipelines, ensuring smooth data flow.
Data Quality Validation and Anomaly Detection:
Generative AI learns patterns and identifies potential data quality issues. Missing values, inconsistencies, and outliers are flagged during data pipeline monitoring. Anomalies are isolated, redacted, and archived, maintaining data integrity.
Automated Data Governance
Generative models assist in metadata capture, data lineage, and business rules. They recommend data classification, access controls, and privacy compliance measures. Organizations can ensure regulatory adherence and enforce organizational policies.
Data Pipeline Optimization
By analyzing historical data, resource constraints, and pipeline performance, generative models suggest optimizations. Reordering steps, parallelization, and alternative processing techniques improve efficiency and scalability.
7. Data Migration: Enhancing Efficiency and Accuracy
Data migration is a critical process that involves moving data from one system or platform to another. Whether it's transitioning to the cloud, upgrading legacy systems, or consolidating databases, data migration requires careful planning and execution. Generative AI plays a pivotal role in streamlining this complex task.
Data Domain Documentation
Generative AI assists in documenting data domains. By analyzing different datasets, it discovers data mappings, relationships, and semantics. This documentation is crucial, especially for legacy systems where tribal knowledge may be sparse. Understanding the source and target data schemas ensures a smooth migration process.
Migration Rationalization
Generative models perform log analysis and identify usage patterns. They generate reports comparing active and obsolete datasets. This rationalization helps organizations optimize data migration strategies—whether it's re-platforming or refactoring. By focusing efforts on relevant data, businesses achieve efficiency gains.
Data Quality and Error Handling
Generative AI automates data quality assessment during cloud data migration. By analyzing large volumes of error logs, it identifies anomalies and inconsistencies. These models also recommend error-handling strategies, ensuring data integrity throughout the migration process.
Post-Migration Validation
After migration, LLMs (Large-Scale Language Models) and Generative AI validate data consistency. They summarize and compare datasets between the legacy platform and the newly migrated data platform. This validation step ensures that data remains accurate and usable.
Performance Optimization
Generative models analyze historical performance data and resource utilization patterns. Based on this analysis, they recommend optimal configurations and strategies. Whether it's adjusting parallelism, fine-tuning resource allocation, or optimizing data pipelines, Generative AI enhances performance during cloud data migration.
Explore how Generative AI can help in Data Democratization
Available Technologies for Implementing Generative AI in Data Analytics
In the realm of Generative AI for data analytics and management, various cutting-edge technologies empower developers and data scientists to harness the potential of machine learning for diverse applications. Here's a list of leading platforms and tools in this domain:
1. Microsoft Azure
-
Azure Machine Learning: A comprehensive suite of cloud-based tools facilitating the creation, training, and deployment of machine learning models. Employing Gen AI within Azure Machine Learning facilitates the creation and deployment of AI-driven data analysis models. Gen AI can optimize model parameters and improve accuracy.
-
For example, Gen AI optimizes machine learning algorithms for predictive maintenance tasks, improving accuracy and efficiency in identifying equipment failures before they occur.
-
Azure Databricks: Integrating Gen AI with Azure Databricks enhances big data processing capabilities. Gen AI can assist in optimizing data workflows, improving efficiency in data analysis tasks.
-
Azure OpenAI Service: Offering large-scale generative AI models with flexible token and image-based pricing models. By utilizing Gen AI in conjunction with Azure OpenAI Service, businesses can harness large-scale generative models for advanced data analysis tasks such as text generation and image synthesis.
-
Copilot: Generates visualizations, insights, DAX expressions, and narrative summaries within Power BI. Incorporating Gen AI with Copilot in Power BI enables automated insights generation and data visualization, empowering users to derive actionable insights from their data effortlessly.
2. Google Cloud Platform (GCP)
-
Google Cloud AutoML: Empowers developers with limited ML expertise to train high-quality custom models. Integrating Gen AI with AutoML streamlines the development of custom data analysis models. Gen AI can automate the model training process, improving model performance.
-
BigQuery ML: Enables data analysts and scientists to build ML models directly on Google's scalable data warehouse. Leveraging Gen AI with BigQuery ML enables the development of machine learning models directly within Google's data warehouse. Gen AI can enhance model accuracy and efficiency.
-
Vertex AI: Customizable models embeddable in applications, with tuning capabilities using Generative AI Studio. Utilizing Gen AI with Vertex AI facilitates the creation of customizable AI models for data analysis tasks. Gen AI can optimize model parameters and improve model interpretability.
-
Generative AI App Builder: Entry-level tool for creating chatbots and search applications. Incorporating Gen AI with the App Builder simplifies the development of chatbots and search applications for data analysis purposes, enhancing user engagement and interaction.
3. Amazon Web Services (AWS)
-
Amazon SageMaker & AWS Bedrock:
By combining Gen AI with SageMaker and Bedrock, businesses can develop and deploy advanced generative AI models for data analysis tasks. Gen AI can optimize model performance and scalability. -
For an example, by leveraging Amazon SageMaker & AWS Bedrock to train deploy a recommendation model. It processes user data, trains the model, and deploys it securely. The model provides real-time personalized content recommendations, continuously improving through user feedback.
-
Amazon Forecast: Gen AI improves the accuracy of sales forecasting models by optimizing parameters and adapting to changing data patterns, enabling businesses to make more informed decisions about inventory and resource allocation.
4. Tableau
-
Tableau Pulse: Powered by Tableau GPT, offering automated analytics and surfacing insights through natural language. This automatically generates insights and visualizations from data, helping analysts identify trends and opportunities more efficiently.
5. Sigma
-
Sigma AI: Integrates AI-powered features including Input Tables AI, Natural Language Workbooks, and Helpbot.
For Example, in finance, this assists in automating financial reporting tasks within Sigma, generating insights and recommendations to improve data accuracy and decision-making.
6. Qlik
-
OpenAI Analytics Connector: Incorporates generative content within Qlik Sense apps.
For an example in Supply Chain, Gen AI integrated with Qlik's Analytics Connector enhances supply chain optimization efforts by generating insights and recommendations based on real-time data analysis.
7. LangChain
-
LangChain: Open-source framework connecting large language models to external components for LLM-based applications.
So, in case of someone facing a language barrier, Gen AI within the LangChain framework can assist with improved language translation accuracy and efficiency, enabling seamless communication across diverse language barriers.
8. IBM Cloud
-
IBM Watson Studio: Empowers businesses to collaboratively develop AI-driven applications through a combination of data analysis, visualization, and machine learning techniques.
In healthcare, this technology assists in analyzing patient data within Watson Studio, helping healthcare providers identify trends and patterns for better diagnosis and treatment planning.
Additionally, open-source tools like Python libraries (e.g., pandas, Scikit-learn, TensorFlow, PyTorch), R programming language, and Jupyter Notebook continue to play crucial roles in data analysis, machine learning, and visualization.
Also, in specialized sectors:
Healthcare: DeepMind by Google aids in early disease diagnosis.
Finance: Kensho offers real-time event recognition for macroeconomic impact analysis.
With diverse generative AI capabilities available, organizations can tailor solutions to meet their specific application needs, whether it's analytics, Natural Language Processing (NLP), or chatbot development. These advancements underscore the ongoing evolution and democratization of AI in data analytics and management.
Final Thoughts on the Impact of Generative AI in Data Analytics
In a data-driven world, Generative AI is reshaping how organizations extract insights from vast datasets, becoming a pivotal tool in data analytics and management.
-
Democratizing Insights: Generative AI broadens access to advanced analytics, empowering users beyond experts to uncover hidden patterns and drive informed decisions, fostering a data-driven culture.
-
Enterprise Usability: Enterprise-ready generative models, leveraging large-scale language models (LLMs), automate tasks like text generation and image synthesis, boosting productivity and efficiency across various domains.
-
Industry-Specific Solutions: Startups focusing on generative AI offer tailored solutions across industries, optimizing processes from supply chain logistics to marketing personalization, and reshaping business operations.
-
Growth Trajectory: Rapid adoption of Generative AI by businesses underscores its growing relevance, though careful consideration of ethical guidelines is essential to mitigate unintended consequences.
-
Ethical Considerations: Upholding security, privacy, and ethical standards is imperative in Generative AI adoption, necessitating transparency, fairness, and accountability in its use.