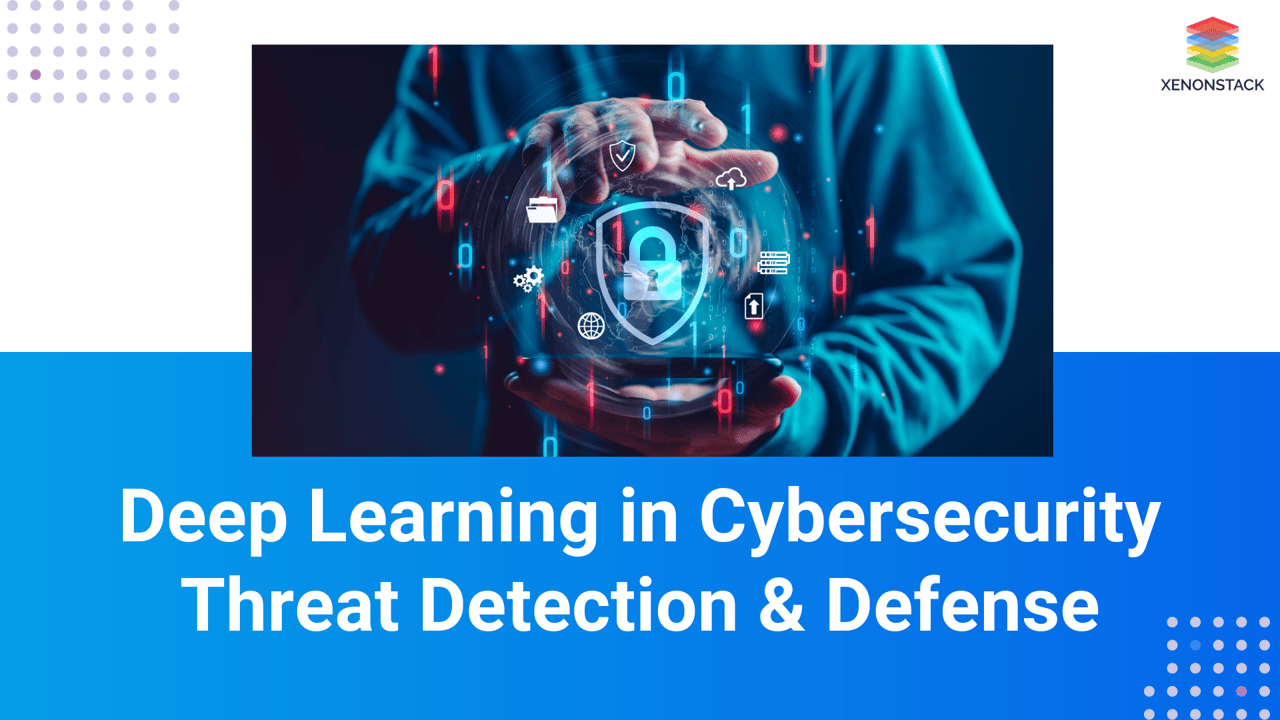
Challenges in Traditional Cybersecurity
The cybersecurity environment has evolved to create a wider attack surface and new, more complex threats. Old-generation security solutions such as firewalls and antivirus programs mostly depend on identified threats and typically represent the signature-based detection system. However, these approaches have limitations:
-
Static Detection: Some traditional methods have inherent drawbacks, especially in poorly defining new or unknown threats that may threaten the networks.
-
High False Positives: Most historical security solutions produce many false alarms that cloud security professionals use to distract from legitimate threats.
-
Manual Intervention: The conventional approaches involve a massive proportion of manual intervention and, therefore, are not adaptable to provide instant countermeasures against a rising threat.
How Deep Learning Enhances Cybersecurity?
Dependent on ML, deep learning involves using artificial neural networks to solve complex problems without exerting any human input by breaking down large datasets to distinguish patterns from them. This technology has emerged as a powerful tool in cybersecurity for several reasons:
Advantages of Deep Learning
Autonomous Learning: Different deep learning structures can analyze large volumes of information, learn from it, and make progress of amity without detailed one-on-one surveillance. Enhanced Pattern Recognition: DL algorithms are good at analyzing data and noting strange and complex patterns within it, which allows them to identify advanced attacks that conventional methods might not notice. Reduced False Positives: Deep learning can detect possible threats much more accurately, thus reducing the number of false positives seen by security personnel.
Key Applications of Deep Learning in Cybersecurity
Deep learning has a range of applications in cybersecurity, including but not limited to:
-
Intrusion Detection Systems (IDS): DL models can inspect traffic and recognize attempted intrusions to clearly distinguish between normal and potential attacks.
- Malware Detection: Malware identification and various types of malware can also be recognized by deep learning algorithms involving file behaviour analysis and their features.
- Phishing Detection: DL can complement conventional approaches of filtering email by evaluating the content within the message and detecting phishing schemes based on minor patterns.
-
Behavioral Analytics: One of the best features of deep learning insight is the ability to detect deep-rooted abnormal activities from end-users who are considered insiders or have had their accounts breached.
Traditional vs. Modern Cybersecurity Framework
Figure 1: Traditional vs. Modern Cybersecurity Framework
Deep Learning Architecture in Cybersecurity
The means of incorporating deep learning in cybersecurity include having a proper architecture that supports the cornerstone of the process, which is data gathering, model learning and identifying threats. Here, we present a very basic flow chart of how a deep learning cybersecurity system might be structured:
Figure 2: Deep Learning Architecture for Cybersecurity
-
Data Collection: Security data is sourced from various areas, such as network traffic, Computers, Devices, and logos.
-
Data Preprocessing: The collected raw data is processed and organized in a manner that is appropriate for implementing machine learning models.
-
Model Training: This work uses labelled datasets to provide deep learning models with features characteristic of cyber threats.
-
Threat Detection: After training, these models scrutinize the data flow and mark any suspicious activity for examination.
Case Studies of Deep Learning in Cybersecurity
Many organizations are already using deep learning to improve their cybersecurity capabilities. Here are a few notable case studies:
IBM Security QRadar
IBM Security QRadar uses deep learning to improve its advanced threat detection system. With the help of network traffic and user activities, QRadar can detect threats and suspicious attempts to violate security. To ensure that the platform Captures any new threat activities, the platform uses machine learning algorithms, which keep.
Darktrace
Darktrace uses deep learning to automate cybersecurity responses. Its Enterprise Immune System is based on the human immune system and measures the level of deviation within an organization. Darktrace's artificial intelligence capability means that without intervention, it can take measures within minutes regarding emerging threats.
Future Trends in Deep Learning
Deep learning has not been fully incorporated into cybersecurity, but the possibilities are enormous. Future developments may focus on:
-
Federated Learning: This approach enables a set of models to be trained jointly by several organizations with access to different data sets to work on without transferring it to other organizations to improve privacy and security.
-
Explainable AI: The creation of explainable deep learning models is growing to enhance the assurance of automated threat detection systems by allowing security specialists to comprehend the reasoning behind the AI's detections.
-
Real-Time Threat Intelligence: A deep learning algorithm integrated in real-time could promptly work with threat intelligence to fight emerging threats.
Final Thoughts
Today, deep learning may be viewed as a breakthrough in cybersecurity as this approach allows organizations to think more proactively about combating constantly escalating cyber threats. With the help of the capabilities discussed, such as monitoring, analysis, and optimization, organizations can improve their defences, minimize false positives, and optimize their security. Therefore, adopting deep learning is expected to be critical as organizations seek to safeguard their web assets due to the changing threat profile.
Read More AI in Cybersecurity Learn More About AI-Driven Threat Hunting: Proactive Cyber Defense