Optimisation of Clinical Trials
Patient Stratification and Recruitment
AI enhances clinical trial feasibility by using EHR and RWD to choose the right patient population. Cohorts are stratified based on clinical information only, with the help of Natural Language Processing (NLP) to minimise undue recruitment time.
Techniques:
-
Predictive Analytics: They also predict patients' eligibility and adherence, making trials more efficient.
-
Simulation Models: Trial predictions are made through simulation via AI to enhance the operational protocol of trials.
Adaptive Clinical Trials
AI makes trial design active by adjusting protocols depending on data received halfway through. The situation changes, and the probabilities of retaining the trials favour using Bayesian models and reinforcement learning.
Case Study: Pfizer used AI in agenda reformulation in oncology studies with mid-trial adaptive adjustments to increase patient benefits and speed drug approval.
AI in Personalised Medicine
Biomarker Discovery
AI learns about biomarkers that can help in treatment and which treatment has a high chance of responding well. ML algorithms evaluate different types of inputs to find the biomarkers of response to therapy and the side effects.
Example: Foundation Medicine employs machine learning techniques to analyze genomic data to identify biomarkers that eventually help administer better-targeted cancer treatments, enhancing cure accuracy.
Predictive Modeling for Treatment Response
AI models anticipate a patient’s reaction to the drugs in their MDA by combining genotypes, phenotypes, and the patient's environment. These models help define the treatment that will be most effective and have fewer side effects.
Techniques:
-
Support Vector Machines (SVMs): SVMs predict patient groups by response tendency.
-
Deep Learning Models: DL architectures, such as autoencoders, incorporate intricate mapping between patient characteristics and treatment results.
AI in Drug Repurposing
-
Knowledge Graphs and Semantic Analysis
AI utilizes knowledge graphs to connect various biomedical data sources, helping to identify existing drugs that could be repurposed for new therapeutic uses. Through semantic analysis and link prediction algorithms, it reveals hidden connections between drugs, targets, and diseases.
-
Similarity Metrics and Pattern Recognition
AI evaluates the chemical and biological similarities between current drugs and new targets. Methods like Tanimoto coefficients and neural similarity metrics assess structural and functional similarities, aiding in discovering candidates for repurposing.
Overcoming Challenges with AI Integration
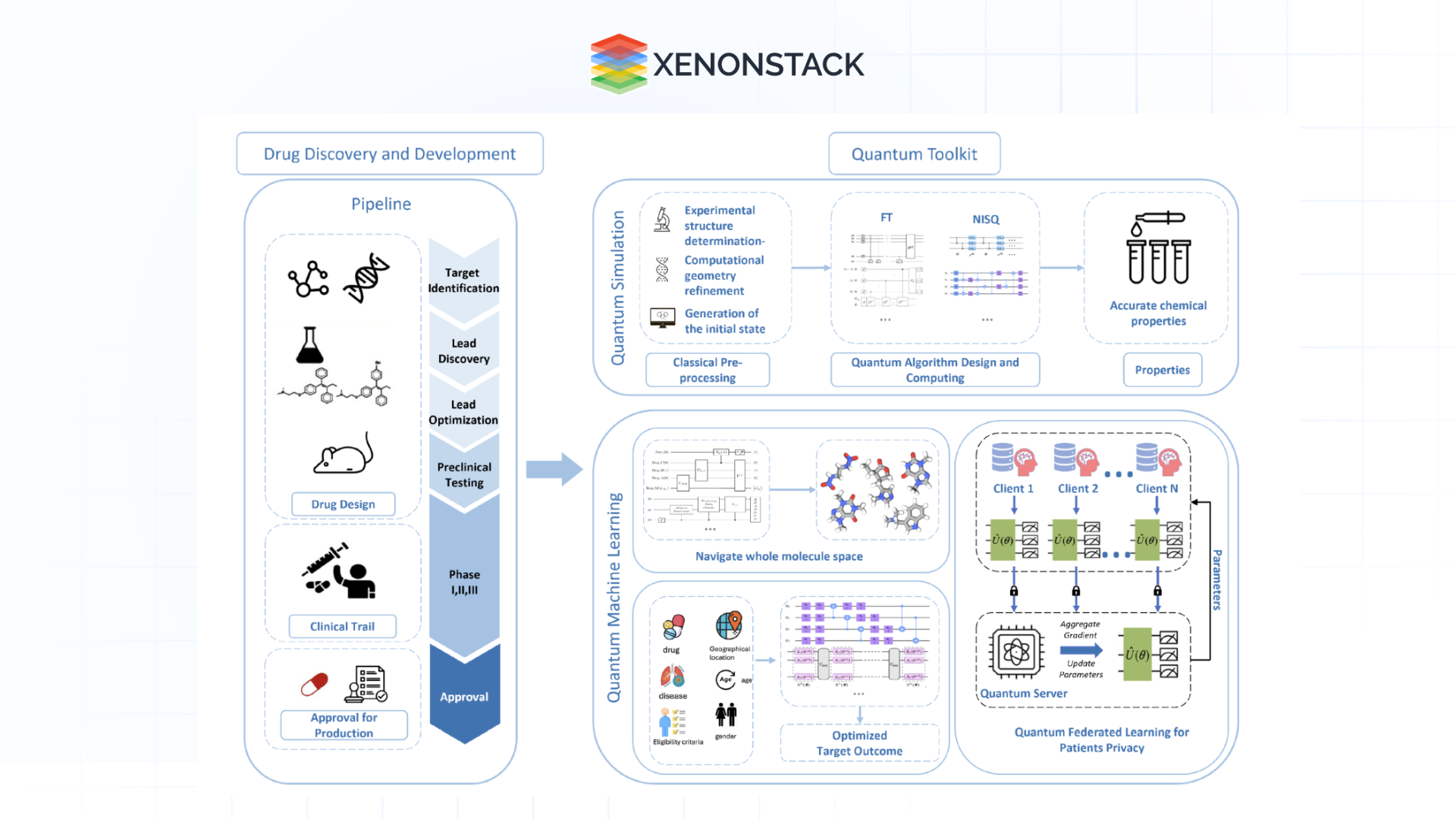
-
Data Quality and Integration
The effectiveness of AI models hinges on the quality of the data on which they are trained. Ensuring that datasets are high-quality, standardised, and well-integrated is crucial. Data normalisation, imputation, and integration pipelines are vital for preparing data for AI applications.
-
Interpretability and Explainability
The “black box” characteristic of some AI models can create challenges in regulatory settings. Creating interpretable models and using explainability methods, like SHAP (Shapley Additive explanations) and LIME (Local Interpretable Model-agnostic Explanations), can build trust and support regulatory approval of Transparent AI.
-
Regulatory and Ethical Considerations
AI-driven drug discovery must comply with strict regulatory standards. Adhering to guidelines from organisations like the FDA and EMA while addressing ethical issues related to data privacy and algorithmic bias is essential for successfully integrating AI.
Future Directions and Innovations
-
Integration of Multi-Modal Data
In the coming years, AI models can expect to incorporate multimodal data, such as imaging, omics, and electronic health records (EHRs), to gain a comprehensive understanding of disease mechanisms and treatment responses.
-
Quantum Computing and AI
The merging of quantum computing and AI presents exciting opportunities for tackling complex molecular simulations and optimisation challenges at remarkable speeds, further expediting drug discovery.
-
AI-Augmented Robotics in High-Throughput Screening
Integrating AI with robotics for automated high-throughput screening can significantly improve precision and scalability, allowing for the rapid evaluation of extensive compound libraries with minimal human involvement.
AI transforms drug discovery and development by improving target identification, refining molecular design, predicting ADMET properties, streamlining clinical trials, enabling personalised medicine, and facilitating drug repurposing. While there are challenges related to data quality, model interpretability, and regulatory compliance, the advantages of incorporating AI are substantial.
As AI technologies advance, their combined use with traditional pharmaceutical practices will likely lead to a new era of quicker, more efficient, and more effective drug development processes, ultimately enhancing patient outcomes and addressing unmet medical needs.