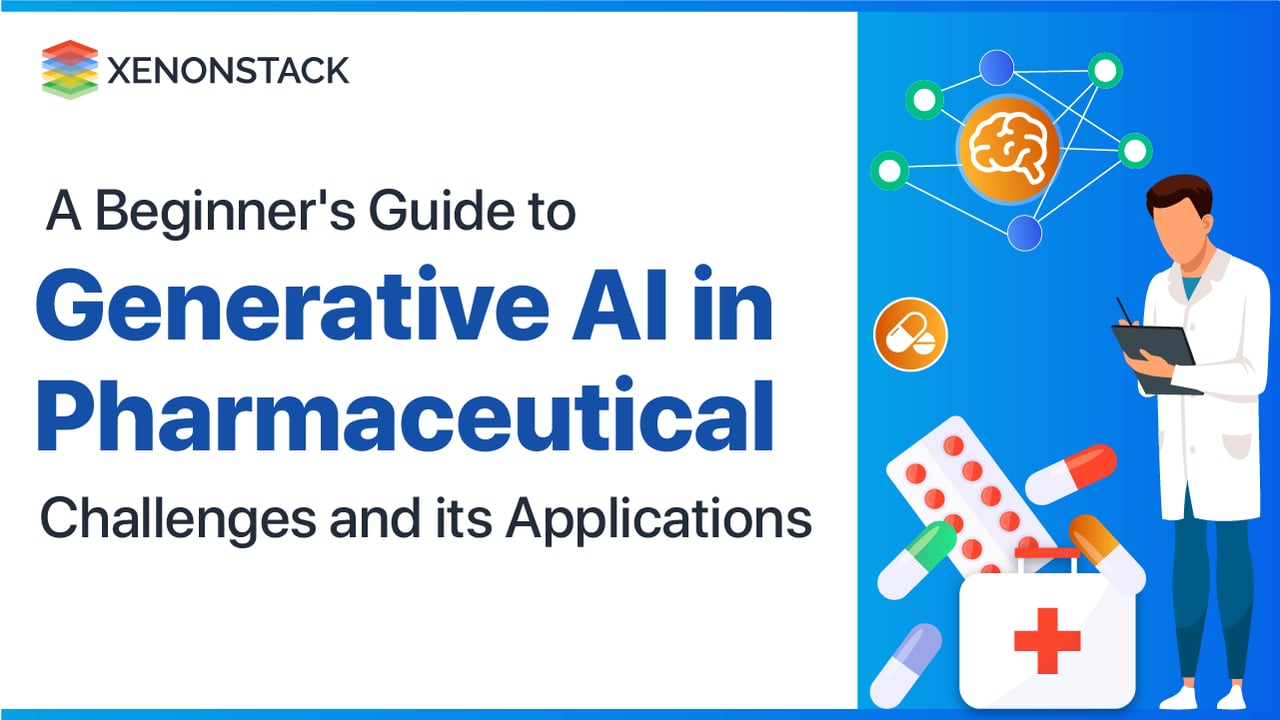
Introduction to Generative AI
Artificial Intelligence (AI) has rapidly transformed diverse industries over the past few years. From healthcare to manufacturing, AI is revolutionizing the way things are done. The pharmaceutical industry has been an early user of AI, especially in drug discovery and development. In this blog, we will focus on one specific area of AI, Generative AI, and its uses in the pharmaceutical industry.
Data Collection and Data Ingestion are the processes of fetching data from any data source. Taken From Article, Artificial Intelligence Adoption Best Practices
What is Generative AI?
Generative AI is a type of AI that involves the use of algorithms to generate new data that is like the input data it has been trained on. The input data can be anything from images, text, or chemical structures. Generative AI is a subset of machine learning, and it uses neural networks to learn the patterns in the input data and generate new data that is like the input data. Generative AI has shown great promise in the pharmaceutical industry, particularly in drug discovery and development. Generative AI can accelerate drug discovery by generating new molecules with specific properties and help pharmaceutical companies develop new drugs faster and more efficiently.
Generative AI is a machine learning algorithm that uses two neural networks – a generator and a discriminator – to create new data. The generator creates new data similar to real-world data, while the discriminator tries to differentiate between the generated and real-world data. Over time, the generator improves output to create data almost indistinguishable from real-world data. Generative AI is a subset of unsupervised learning, meaning it can learn from unlabelled data. This advantage is significant because it allows the algorithm to generate new data without requiring pre-existing datasets.
A systematic approach to knowledge integration that ensures consistent use and reuse of knowledge. Click to explore about our, MDM Insurance for Finance Management
What are the types of Generative AI tools used in Pharmaceutical?
In the pharmaceutical industry, various Generative AI tools address different challenges and tasks. Here are some common types of Generative AI tools used in the pharmaceutical sector:
Generative Adversarial Networks (GANs)
GANs are widely used in pharmaceutical research for drug design and molecule generation tasks. GANs consist of a generator network that creates synthetic samples and a discriminator network that evaluates the authenticity of these samples, resulting in the generation of novel molecules with desired properties.
Recurrent Neural Networks (RNNs)
RNNs are employed for sequential data generation, making them helpful in generating novel chemical structures or optimizing drug properties. RNNs can learn patterns from sequential data, allowing them to generate new sequences with desired characteristics.
Variational Autoencoders (VAEs)
VAEs are used for drug discovery and optimization tasks. VAEs learn the underlying distribution of chemical structures and enable the generation of new molecules with specific properties. They are instrumental in exploring chemical space and generating diverse compounds.
Deep Reinforcement Learning
Deep reinforcement learning techniques are applied in drug discovery and optimization processes. These algorithms learn through trial and error and can generate molecules with desired properties by maximizing reward signals based on predefined objectives.
Transformer Models
Transformer models, such as the popular GPT (Generative Pre-trained Transformer) architecture, are utilized in natural language processing tasks, including text generation and drug discovery. These models can generate coherent and contextually relevant text, making them valuable for generating chemical descriptions and drug-related text.
It's important to note that the field of Generative AI is continuously evolving, and new tools and techniques are being developed and adapted to address the specific challenges in the pharmaceutical industry. The choice of a Generative AI tool depends on the specific task and the desired outcome sought by pharmaceutical researchers and professionals.
Explainable Artificial Intelligence in manufacturing improves efficiency, workplace safety, and customer satisfaction by automating tasks. Taken From Article, Explainable AI in Manufacturing Industry
What are the applications of Generative AI in Pharmaceutical?
The applications of Generative AI in Pharmaceutical are described below:
Drug Discovery
Drug discovery is a complex and time-consuming process that involves the screening of millions of compounds to identify potential drug candidates. Generative AI can help accelerate this process by generating new molecules with specific properties. These molecules can be designed to target specific diseases or conditions and can be optimized for efficacy, safety, and pharmacokinetics.
Generative AI can also be used to design new chemical compounds that have never been synthesized. This can lead to the discovery of new drugs with unique properties and potentially be used to treat diseases that currently have no effective treatments.
Lead Optimization
Once a potential drug candidate has been identified, the next step is lead optimization. This involves modifying the structure of the drug molecule to optimize its pharmacological properties, such as efficacy, safety, and pharmacokinetics. Generative AI can help in this process by generating new molecules similar to the lead compound but with improved properties. These new molecules can then be synthesized and tested for efficacy and safety.
Drug Repurposing
Drug resurging/repurposing is identifying new uses for existing drugs. Generative AI can help in this process by generating new molecules similar to existing drugs but with different properties. These new molecules can then be tested for their therapeutic potential for other diseases or conditions.
Personalized Medicine
Personalized medicine is an emerging field that tailors treatments to individual patients based on their genetic makeup and other characteristics. Generative AI can design personalized drugs optimized for specific patient populations. Generative AI can help improve treatment outcomes and reduce side effects by generating new molecules tailored to individual patient characteristics.
Generative AI and the Pharmaceutical Industry
The pharmaceutical industry is one of the most heavily regulated industries in the world. Developing new drugs is a lengthy and expensive process that requires extensive testing and approval from regulatory bodies such as the FDA (Food and Drug Administration). However, generative AI has the potential to revolutionize the drug development process by reducing the time and cost required to bring a new drug to market.
Drug Discovery
One of the best applications of generative AI in the pharmaceutical industry is drug discovery. Drug discovery is identifying and testing potential new drug candidates and testing them to determine their effectiveness and safety.
Traditionally, drug discovery has relied on screening large chemical libraries to identify compounds with potential therapeutic value. However, this process is time-consuming and expensive, with many potential compounds failing to make it past the early stages of development.
Generative AI can accelerate drug discovery by generating new molecules with specific properties. For example, a generative AI algorithm could be trained to generate molecules with specific binding properties to a particular protein. This exponentially reduces the time and cost required to identify potential drug candidates.
Drug Design
Once a potential drug candidate has been identified, the next step is to design a drug that can effectively target the desired protein. This complex process requires the synthesis and testing of multiple drug variants.
Generative AI can be used to design new drugs by generating molecular structures that are optimized for specific properties. For example, a generative AI algorithm could be trained to generate molecules optimized for potency, selectivity, and bioavailability.
Clinical Trials
Clinical trials are an essential component of the drug development process. They are used to determine the safety and efficacy of new drugs before they can be approved for use by regulatory bodies.
Generative AI can optimize clinical trials by identifying patient subgroups likely to respond to a particular treatment. For example, a generative AI algorithm could be trained to identify genetic markers that predict a patient's response to a particular drug.
Generative AI has been a massive advancement in Manufacturing and Production. Taken From Article, Generative AI in Manufacturing Industry
What are the challenges of Generative AI in Pharmaceuticals?
- Data Quality: One of the prominent challenges is the quality of data used to train the Generative AI models. Poor quality data can lead to incorrect models and inaccurate predictions.
- Regulatory Compliance: Pharmaceuticals are subject to strict regulations, and any new technology must comply. The use of Generative AI in pharmaceuticals may require regulatory approval, which can be a time-consuming and costly process.
- Ethical Concerns: As with any technology, Generative AI raises ethical concerns. One concern is the potential misuse of Generative AI to create new drugs without proper testing and evaluation. Another drawback is the use of Generative AI to create drugs that are only effective for specific demographics, which can lead to inequality in access to healthcare.
- Interpretability and Explainability: Generative AI models often operate as black boxes, making it hard to interpret and explain the underlying decision-making process. In the pharmaceutical industry, where transparency and interpretability are crucial for regulatory approvals and ethical considerations, ensuring transparency can be challenging.
- Ethical Considerations: Generative AI in the pharmaceutical industry raises ethical concerns about patient privacy, data protection, and potential biases in training data. Addressing these ethical considerations and ensuring responsible AI implementation is of paramount importance.
- Validation and Accuracy: Generative AI models' accuracy and reliability must be rigorously validated before deploying them for critical pharmaceutical tasks. Establishing robust validation methodologies specific to the pharmaceutical domain can be complex and time-consuming.
- Collaboration and Integration: Implementing Generative AI in the pharmaceutical industry requires collaboration between AI experts, pharmaceutical researchers, and domain specialists. Bridging the gap between AI expertise and pharmaceutical domain knowledge can be challenging during integration.
- Intellectual Property Rights: Generative AI in pharmaceuticals raises questions about who owns the intellectual property of drugs created through this technology.
- Integration with Existing Systems: Generative AI must be integrated with existing systems in pharmaceuticals, which can be a challenge due to the complexity of these systems. Additionally, pharmaceutical companies may need to invest in new hardware and software to support the use of Generative AI.
- Technical Expertise: The development and implementation of Generative AI models require a high level of technical expertise. Companies may need to hire additional personnel with machine learning, deep learning, and data science expertise to implement this technology successfully.
- Cost: Generative AI can be expensive, especially in the initial stages of development and implementation. Companies must carefully weigh the costs and benefits of using Generative AI before investing in this technology.
While Generative AI has enormous potential in pharmaceuticals, it has challenges. It is essential for pharmaceutical companies to carefully evaluate these challenges and ensure that they have the necessary resources and expertise to implement this technology successfully. Addressing these challenges necessitates a multidisciplinary approach involving experts in AI, pharmaceutical research, regulatory affairs, and data privacy. Overcoming these hurdles will unlock the potential of Generative AI, enabling advancements in drug discovery, personalized medicine, and improved patient outcomes in the pharmaceutical industry.
Conclusion
Generative AI has the potential to revolutionize the pharmaceutical industry by reducing the time and cost required to bring a new drug to market. It can be used to accelerate the drug discovery process, design new drugs, and optimize clinical trials. However, many challenges must be overcome before generative AI can be widely adopted in the pharmaceutical industry. These challenges include the need for more extensive and diverse datasets and the development of robust validation and testing procedures. Despite these challenges, the potential benefits of generative AI are significant, and we are likely to see more and more.
- Read about Data Analytics in Healthcare Industry
- Explore about Internet of Things Solutions for Smart Manufacturing