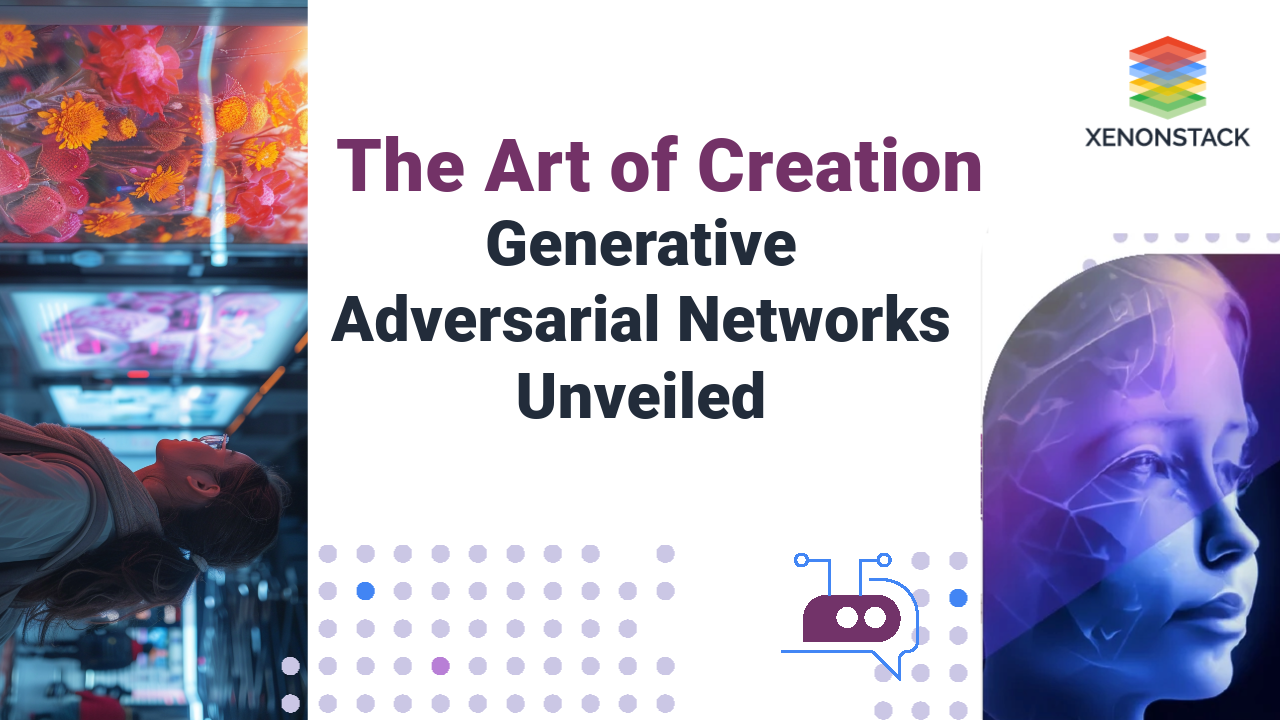
Generative Adversarial Networks rather are the new generation of deep learning models created for efficiently producing non-realistic images. A GAN is constructed from two sub-models: the generator that is used to create images and the discriminator that is used to evaluate them. During the process they are getting better through learning, providing the network with better and better synthetic images which look identical to actual images. Hence, object detection has been boosted a lot by the improvement in GANs’ performance in many applications like deepfake synthesis, style transfer, and image super-resolution.
GAN Architecture Overview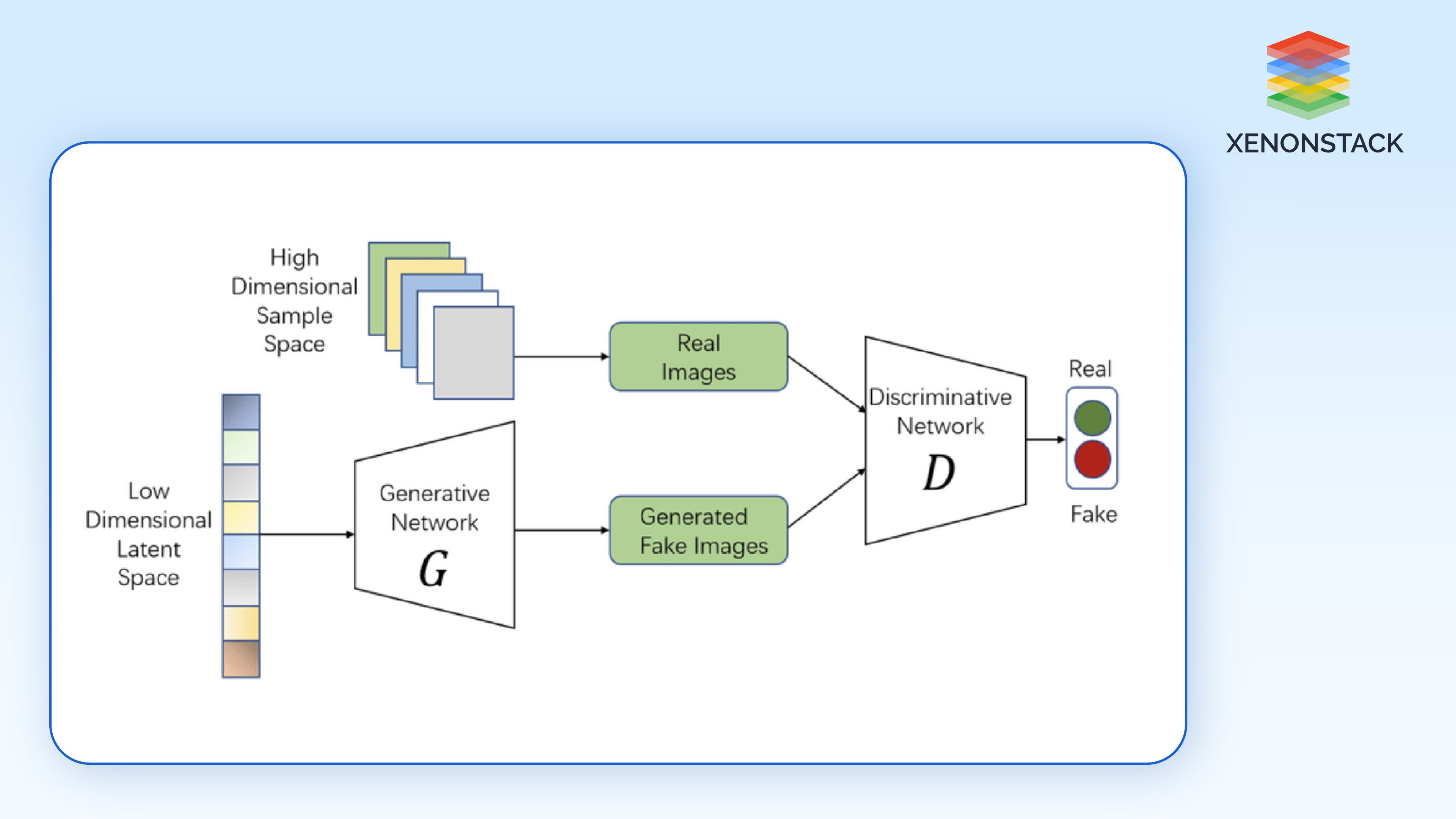
Fig 1.0: Generative Adversarial Network (GAN)
The foundation of GANs is on two competing networks:
-
Generator: This network prescribes real data from random noises. A generator is a neural network that takes some embedding space (for example, a space of low dimensionality) and outputs some target data space, for example, images. The goal of the generator is to create data that could be classified as real data by the discriminator.
-
Discriminator: A binary classifier that separates fake data from actual data. The discriminator should give the highest likelihood to the real data and almost zero likelihood to synthesized data generated by the generator.
Fig 2.0: Generator and Discriminator in GANs
The training of GANs follows a minimax game between the generator and the discriminator:
-
The generator improves by producing more realistic images, attempting to fool the discriminator.
-
The discriminator becomes more adept at distinguishing real from fake, continually challenging the generator.
The objective is to reach an equilibrium where the discriminator cannot tell real images from generated ones, thus producing highly realistic images.
Why Generative Adversarial Networks are the Go-To Choice for Image Creation?
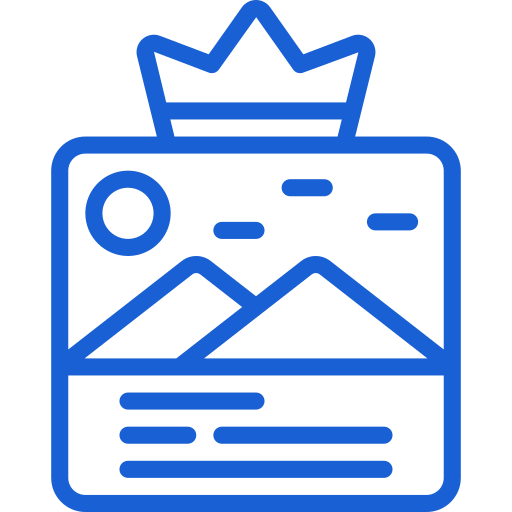
Exceptional Image Quality
GANs are renowned for generating images indistinguishable from real photographs, making them ideal for applications requiring high visual fidelity
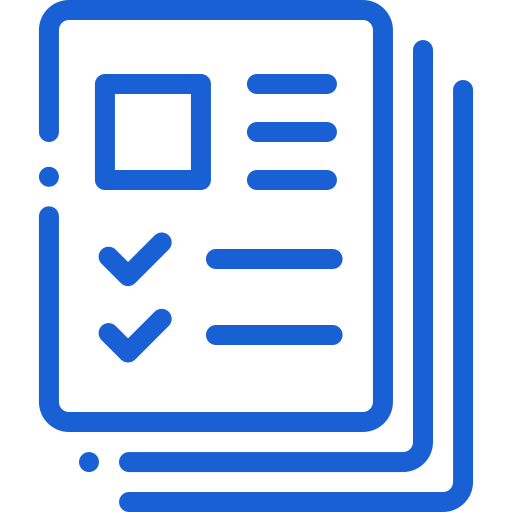
Wide Range of Applications
These networks can be utilized in various domains, including art generation, video game design, and medical imaging, showcasing their versatility
Deepfake Technology
Deep fakes are fake images or videos generated using GANs to generate near-real-life images, videos, or even audio. They generally involve swapping one person’s face with that of another in a video or convincingly changing the tone, often through the use of deep learning. As a result of training GAN-based models on the video and audio databases, the output fakes the authentic content due to realism.
How GANs Create Deepfakes:
GANs play a central role in the generation of deepfakes, with the generator responsible for synthesizing realistic images and the discriminator ensuring these images are indistinguishable from real data.
-
Face Swapping and Face Reenactment: With modifications such as StarGAN or CycleGAN, GANs help transfer specific facial features of one person onto the face of another in a technique called face swap. The generator synthesizes photorealistic face images, and the discriminator aids in guiding the generator to produce a perfect face with suitable locality and motion of muscles.
-
Audio and Speech Generation: GAN brings not only deep fake video but also deep fake audio. Considering the architecture of GANs, these are used for copying voice data, mimicking its speech patterns, tones, voices, and more. Examples of such models include WaveGAN and Tacotron-GAN, which produce realistic human speech, which, when overlaid with realistic facial movements, results in a very believable video.
-
Latent Space Manipulation with Autoencoders: It has been discussed that the autoencoder can be used with GANs to approximate map the real image in a latent space. It enables deep fake models to intake and understand the primary attributes of a person’s face, including their expressions and head movements, which becomes useful to the generator as it can easily perform the transfer on new Video frames.
-
Temporal Consistency and Video Generation: Timing remains a problem in generating deepfakes when dealing with video frames since all the frames must exhibit equal motion. To handle this issue, TGANs have evolved to learn spatiotemporal features to ensure that the synthesized face is moving uniformly and expressing itself uniformly in frames of the video domain.
Harness the power of Generative Adversarial Networks (GANs) to revolutionize your digital marketing strategies with personalized content and stunning visuals. Explore how GANs can elevate your marketing game
Limitations and Technical Challenges:
While GANs can create highly realistic deepfake, there are still technical challenges involved in improving the quality of the output:
-
Facial Expression and Feature Alignment: Because deepfake uses GANs, one major constrain is synchronizing the intricate details of facial expressions or head movements or even illuminance with the target person’s face Higher quality 3D reconstruction models like the 3D Morphable Models (3DMM) are being adopted with the aim of increasing realism in the face replacement by mapping faces from 2D to 3D in space to be manipulated in t the GANs
-
Artifacts and Blurriness: Deepfakes, for example, usually exhibit small artifacts or kernel blurring around the face, which does not allow them to remain unnoticed, even in refined examples or experiments involving rotational motion or varying illumination. There are efforts being made to build up super-resolution GANs that are used to enhance the fine details of artifacts present in the image.
Advanced Techniques in Deepfake Generation:
Several cutting-edge techniques are being integrated with GANs to make deepfakes more sophisticated:
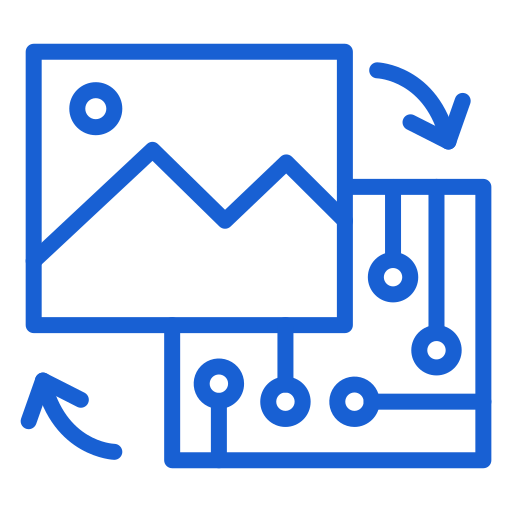
StyleGAN for Precise Manipulation
StyleGAN enables targeted manipulation of generated images, allowing deepfake creators to adjust features such as hairstyle, skin tone, or facial characteristics without altering the identity of the individual being depicted
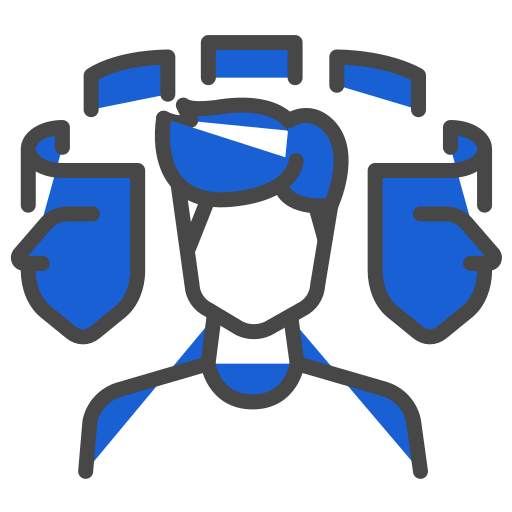
Learning for Deepfake Creation
A major hurdle in deepfake creation is obtaining numerous images or videos of the target. New GAN models employing few-shot learning can generate deepfakes using only a few images, reducing data requirements
Applications
The applications of deepfakes go beyond entertainment and fun. Deepfakes can be used in:
-
Virtual Actors in Films: With deepfakes, filmmakers can reanimate characters or just swap out the actors in your movie with other people, even if they are dead or have never signed up for that film. Sometimes, they can simulate realistic images of past leaders/appendages to an extent that can hardly be compared with other representations.
-
Virtual Avatars for Social Media and Games: New deep fake technologies are making digital avatars of users mimic their facial and even physical gestures in real-time, especially in video games and virtual meetings.
-
Medical Applications: Deepfake models are further being considered for training models in surgical procedures to replicate a patient’s facial movements during surgery to make training more genuine for such professionals.
Ethical challenges
While deepfakes offer innovative potential, there are serious ethical challenges that must be addressed:
-
Misinformation and Fake News: Unfortunately, deepfakes could very much be incriminated in the creation of fake news and defamation material. You have propaganda that is almost impossible to argue against because, using deepfakes, someone can get real videos of some public » shining personality or take some historical footage and insert a totally fake narrative into it.
-
Consent and Privacy Issues: This technology can, therefore, be exploited to produce fake consent pornography or other interpersonal interactions that infringe upon privacy and cause extreme discomfort and harassment.
-
Detection and Prevention: In order to reduce the abuse of deepfake technology, there are various deepfake detection solutions under construction. These systems, frequently of the neural network kind, scrutinize paradoxes comprising incapable blinking, tiny differences at the pixel level, or distortions in the original media. GANs are themselves used in detection where adversarial training is used in order to develop stronger detection methods that are resistant to both primitive deepfakes and the more advanced ones.
Discover how Xenonstack harnesses the power of Generative Adversarial Networks (GANs) to elevate image synthesis capabilities and create stunning visuals tailored to your business needs. Explore our Expertise
Neural Style Transfer
It refers to the process of mapping the style of one image to another, generally without regard to a change in content. The GANs, particularly CycleGAN and Pix2Pix, are key models for these tasks.
Fig 3.0 Cycle GANs
How Style Transfer Works
In CycleGAN, one generator is trained to generate images from one domain to another, that is, from real-world photographs to paintings. It should be noted that the main facility of this network is that it is not necessary to combine two sets of data; thereby it can be used in switching between two absolutely different sets of data without tying two images. In other words, we can transfer any photo to the picture in the style of Van Gogh, which will have the content structure of the initial picture with the help of CycleGAN in artistic style transfer.
Techniques
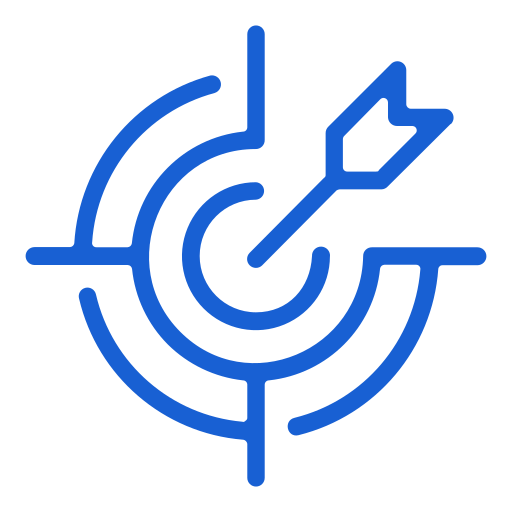
Perceptual Loss
GANs use perceptual loss functions to measure differences in high-level activations from networks like VGG. This ensures that the generated image matches the original's style while retaining the input image's content
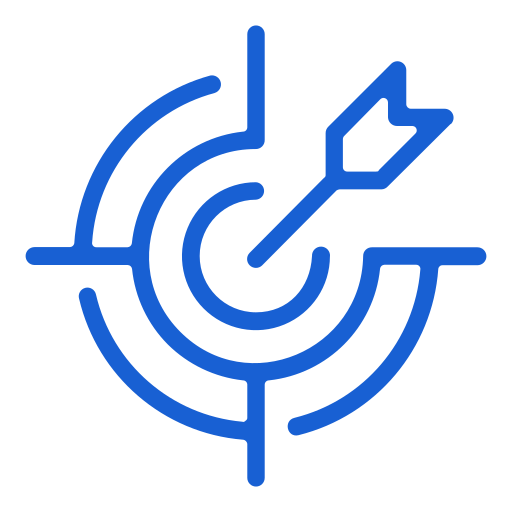
Disentangled Representation
Certain GAN variants focus on decoupling content and style representations, allowing the model to adjust only the style (coloring and texturing) while keeping the content (shapes and objects) intact
Applications
-
Style transfer is used in digital art, creating social media filters, and video games. Artists use GAN-based models to transform photographs or sketches into paintings, effortlessly achieving different stylistic variations.
Image Super-Resolution
Image super-resolution is a process of recovering a low-resolution image in its high-resolution form. GANs, and more importantly, SRGAN, performed very well in restoring fine details and generating high-fidelity images.
How Image Super-Resolution Works:
-
First, SRGAN learns the generator of SISR models to generate high-resolution images from low-resolution inputs; second, the discriminator of SISR models is learned according to the quality of images generated by the generator in view of real high-resolution images.
-
A perceptual loss function is employed, which guarantees the desired pixel-level detail in the synthesized high-resolution images and ensures that, compared with ground-truth high-resolution images, they appear pleasing to the eye.
Advanced Techniques:
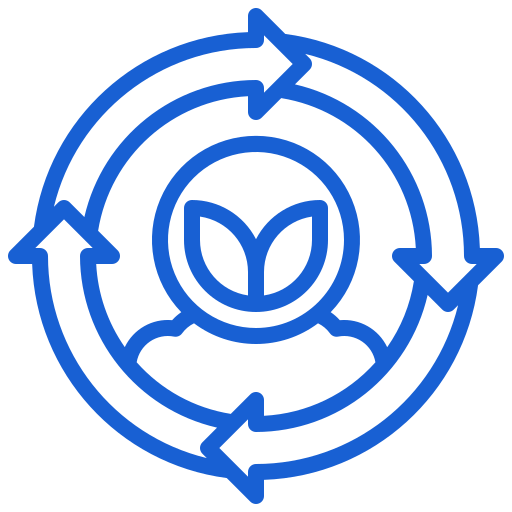
Residual Blocks
In CBAM, the authors again utilize several procedures for training the SRGAN, including residual blocks, to prevent the loss of details during the upsampling process
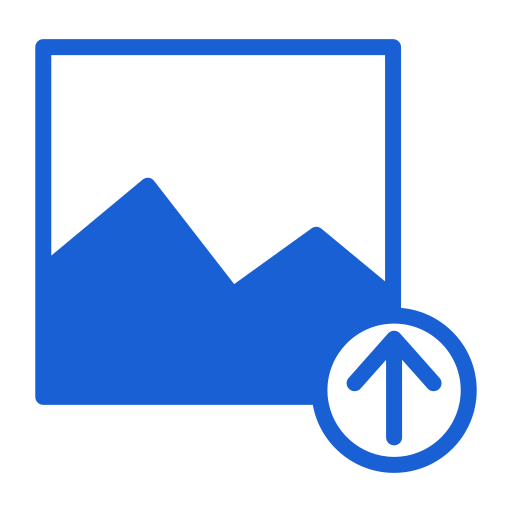
Pixel Shuffle
This layer is applied to raise the degree of the resolution without introducing a jagged appearance, which degrades the continuity of edges and fine details on the prints
Applications
Super-resolution GANs have a variety of applications:-
Medical Imaging: Enhancing low-quality scans to ensure doctors can make more accurate diagnoses.
-
Satellite Imaging: Improving the quality of satellite images for environmental monitoring and geographical mapping.
-
Video Enhancement: Restoring or upscaling old videos to HD or 4K resolutions.
Challenges with GANs
Although GANs seem to be effective image-generation models for a variety of applications, there are some drawbacks as well:
-
Training Instability: There are a few problems with GAN-based systems, one of which is that training can be unstable. For instance, mode collapse occurs when the generator repeatedly creates a few images instead of a variety. It becomes challenging to train a generator and a discriminator simultaneously.
-
High Computational Costs: Aspiring paternal reserves, in this case aspiring fathers, when locating migraines, seek pregnant dark women wherever they are.
-
Ethical Issues: GANs have also been employed to create fake media sources. Some discussions exist, and some works are being planned to limit the application of GANs to socially appropriate activities only.
Final Thoughts
Since its introduction in the computer science community, GAN has set new benchmarks for image synthesis, with applications in deep fake, style transfer, super-resolution of images, and other areas. Due to this constant interaction between the generator and the discriminator, the outcomes obtained in cases where GAN is used are usually more appealing and reasonable.
Nevertheless, there are challenges, including instability of the training process and ethical issues; the possibility of GANs is immense in media and art, in medicine, and much more. Nevertheless, as with any form of research, as time goes on, the present generative adversarial network models will distort the current perception of the image regeneration process, with the view to coming up with more diverse, creative, scientific, and technological solutions.
Learn more about Augmented Reality (AR) and Virtual Reality (VR) Explore more about Vision Transformers (ViTs)