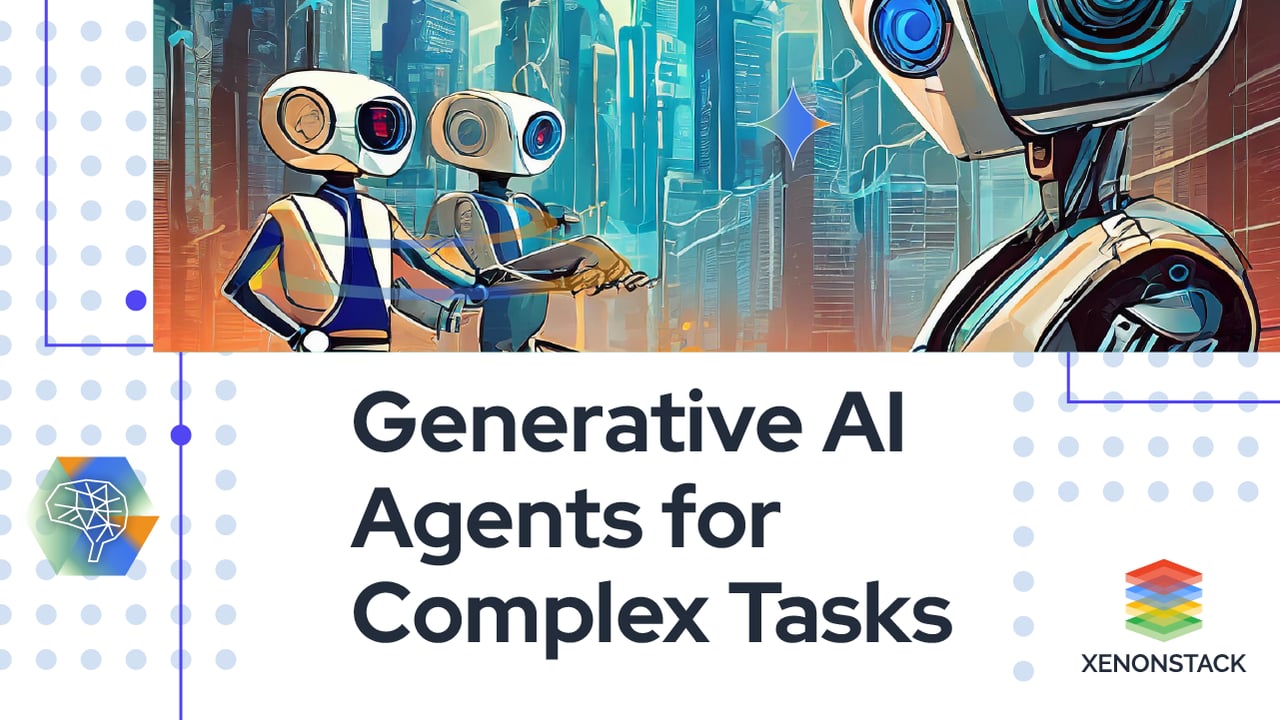
Intelligent Process Automation
Generative AI has moved from a mere concept to a mainstream technology, receiving significant attention and investment. The arrival of AI Agents capable of collaborating towards specific goals marks a significant turning point in the contemporary technological landscape.
Autonomous AI agents are gaining popularity for automating complex tasks, interacting with humans, and augmenting products. They have several use cases, such as finding support docs, writing rules, and solving complex problems. Start-ups are making new use cases with custom AI agents. However, building an agent that meets customer expectations every time is challenging due to the non-deterministic nature of raw LLM tech.
Applications of Autonomous AI Agents
AI agents can create a variety of outputs, including text, images, videos, and music. These outputs are based on the user's inputs and the agent's application area. Each generative AI agent can be customized to meet the user's specific needs and preferences by adjusting its parameters, features, and functionalities. Depending on their design and particular applications, these agents can be classified into various types.
The most common types include natural language processing (NLP) agents, image and video generation agents, music generation agents, and content recommendation agents.
-
Text generation agents can create natural language responses to text-based queries, mimicking human-like conversations or writing styles.
-
Image and video generation agents can synthesize realistic images or videos from user inputs, such as sketches, captions, or keywords.
-
Music generation agents can compose original pieces of music in various genres, styles, and moods based on user inputs or preferences.
-
Content recommendation agents can use machine learning algorithms to suggest relevant content to users, such as articles, products, or movies, based on their previous interactions or feedback.
Analysing Autonomous AI Agents
Autonomous AI agents are systems that can produce novel and diverse outputs based on some input or goal. AI agents have been utilized for a myriad of intricate tasks, including natural language generation, image synthesis, music composition, and more.
However, using generative AI agents for complex tasks also poses some benefits and drawbacks that must be carefully considered.
Some of the potential benefits of using generative AI agents for complex tasks are:
-
Enhanced Human Creativity and Productivity: They can enhance creativity and productivity by providing new ideas, solutions, or perspectives humans may not have considered.
-
Cost and Time Efficiency in Complex Tasks: Automating or augmenting some aspects of the process can reduce the cost and time of performing complex tasks.
-
Improved Quality and Diversity of Outputs: They can improve the quality and diversity of the outputs by generating multiple options or variations that can be evaluated or refined by humans or other agents.
-
Creation of Novel or Personalized Content: By enabling new forms of expression, communication, or interaction, they can create novel or personalized content appealing to different audiences or contexts.
Some of the potential drawbacks of using generative AI agents for complex tasks are:
-
Ethical, Social, or Legal Challenges They can pose ethical, social, or legal challenges by generating outputs that may be harmful, misleading, offensive, or infringing on the rights or privacy of others.
-
Technical or Operational Risks: They can introduce technical or operational risks by generating outputs that may be unreliable, inaccurate, inconsistent, or incompatible with the intended purpose or environment.
-
Reduced Human Agency or Responsibility: They can minimize human agency or responsibility by generating outputs that may influence or replace human decisions or actions without proper oversight or accountability.
-
Psychological or Emotional Issues: They can create psychological or emotional issues by generating outputs that may positively or negatively affect human emotions, expectations, or trust.
AI Agents: Implications Across Industries
AI agents are software systems that can perform tasks requiring human intelligence. They have been used in various industries, but they raise ethical, social, and legal implications that must be addressed.
Some of the implications of using AI agents are:
Accountability: Who is responsible for the actions and outcomes of AI agents? How can AI agents be transparent, explainable, and auditable?
Privacy: How can we protect the personal data and information that AI agents collect, process, and share? How can we ensure that AI agents respect users' and stakeholders' privacy rights and preferences?
Bias: How can we prevent or mitigate the potential biases and discrimination that AI agents may exhibit or amplify? How can AI agents be fair, inclusive, and diverse?
Safety: How can we ensure that AI agents are reliable, robust, and secure? How can we prevent or handle the possible errors, failures, or attacks that may affect AI agents?
Human-AI interaction: How can we design AI agents that are user-friendly, trustworthy, and respectful? How can we foster a positive and productive relationship between humans and AI agents?
Social impact: How can we assess and monitor the social and environmental effects of AI agents? How do we strike a balance between the advantages and potential risks of AI agents for diverse groups and stakeholders?
Autonomous AI Agents: Jobs & Labor Market Impact
Generative AI agents create diverse content and have various applications across industries. However, they may also displace human workers who perform similar tasks. The impact of productive AI agents on job displacement and the labor market needs to be clarified. It depends on factors like the quality, cost, and ethics of the content produced, the demand and supply of human skills and creativity, and the governance of AI systems. It's essential to consider different scenarios before implementing generative AI agents.
-
Complementing Human Workers: Generative AI agents complement human workers by enhancing their productivity, creativity, and innovation. They provide new opportunities for learning, collaboration, and value creation. Human workers can dedicate their attention to tasks of greater complexity that demand emotional intelligence, critical thinking, and social skills. As new roles and sectors emerge, the labor market becomes more dynamic and diverse.
-
Replacing Human Workers: Generative AI agents replace human workers by outperforming them in quality, speed, and cost. They produce content that is indistinguishable from or superior to human-made content. Human workers lose their jobs or face lower wages and benefits. The labor market becomes more polarized and unequal, as some workers benefit from AI while others are left behind.
-
Coexisting with Human Workers: Generative AI agents coexist with human workers by offering different content and services. They produce novel and diverse content, but only sometimes realistic or accurate. Human workers make reliable and trustworthy content, but only occasionally original or varied. The labor market becomes more segmented and competitive as workers and consumers have more choices and preferences.
AI Agent Safety: Development & Deployment
AI agents can benefit various domains, such as health, education, transportation, and entertainment. However, AI agents also pose potential safety risks that must be carefully considered and mitigated in their design and deployment. Some of these risks include:
-
Unintended or harmful consequences: AI agents may behave in ways not aligned with the goals or values of their users, stakeholders, or society. For example, an AI agent may optimize for a narrow objective without considering broader implications or trade-offs or exploit loopholes or vulnerabilities in its environment or reward function.
-
Lack of transparency or explainability: AI agents may operate in opaque or complex ways that humans find difficult to understand, interpret, or verify. For example, an AI agent may use a black-box model or algorithm that produces outputs without revealing the underlying logic or rationale or may generate results inconsistent or contradictory with human expectations or intuition.
-
Loss of human control or autonomy: AI agents may act independently or unpredictably without human oversight, intervention, or consent. For example, an AI agent may take irreversible or irreversible actions without human approval or override or manipulate human decisions or preferences.
Several approaches and principles have been proposed and applied to address these potential safety concerns in the development and deployment of AI agents. Some of these include:
-
Value alignment: AI agents should be designed and trained to align with the goals and values of their users, stakeholders, and society. This may involve eliciting and incorporating human feedback, preferences, and norms into the AI agent's objectives, constraints, and incentives and ensuring that the AI agent respects human dignity, rights, and autonomy.
-
Transparency and explainability: AI agents should be designed and deployed to provide clear and understandable information about their behavior, outcomes, and impacts on humans. This may involve using interpretable or explainable models or algorithms, providing meaningful and relevant explanations or justifications for the AI agent's actions or decisions, and enabling human access and auditability of the AI agent's data and processes.
-
Human oversight and control: AI agents should be designed and deployed to allow for human oversight, intervention, and consent. This may involve establishing appropriate levels of human involvement and authority over the AI agent's actions or decisions, providing mechanisms for human monitoring and feedback on the AI agent's performance and behavior, and ensuring that humans can stop or correct the AI agent if needed.
Advances in Autonomous AI for Complex Tasks
The future developments and advancements in the field of generative AI agents for complex tasks are promising and involve addressing various challenges. Some of the potential future developments and advancements in the field of Generative AI agents for complex tasks on which Xenonstack is focussing:
-
Improving Quality, Diversity, and Coherence: We are improving the quality, diversity, and coherence of the generated outputs by using better models, data, and evaluation metrics.
-
Control over Style, Content, and Structure: We are developing methods to control the generated outputs' style, content, and structure by incorporating user feedback, preferences, and constraints.
-
Enhancing Creativity, Originality, and Novelty We are enhancing the generated outputs' creativity, originality, and novelty by exploring different modalities, domains, and genres.
-
Increasing Scalability, Efficiency, and Robustness We are increasing the scalability, efficiency, and robustness of generative AI agents by leveraging distributed computing, parallel processing, and adversarial learning.
-
Addressing Ethical, Social, and Legal Implications: We are Addressing the ethical, social, and legal implications of generative AI agents by ensuring fairness, accountability, transparency, and safety.
Future of Multi Systems or Compound AI
Autonomous AI agents can automate tasks, interact with humans, and enhance products. Generating diverse content types, including text, images, videos, and music, is feasible, yet maintaining customer satisfaction remains a challenge due to the unpredictable nature of raw LLM technology. The effects on job displacement and the labor market hinge on factors like content quality, cost, ethical considerations, and AI system governance. Advances in generative AI agents aim to improve the quality and diversity of outputs, enhance control, and address ethical, social, and legal implications.
-
Click to explore Autonomous Agents for Software Testing