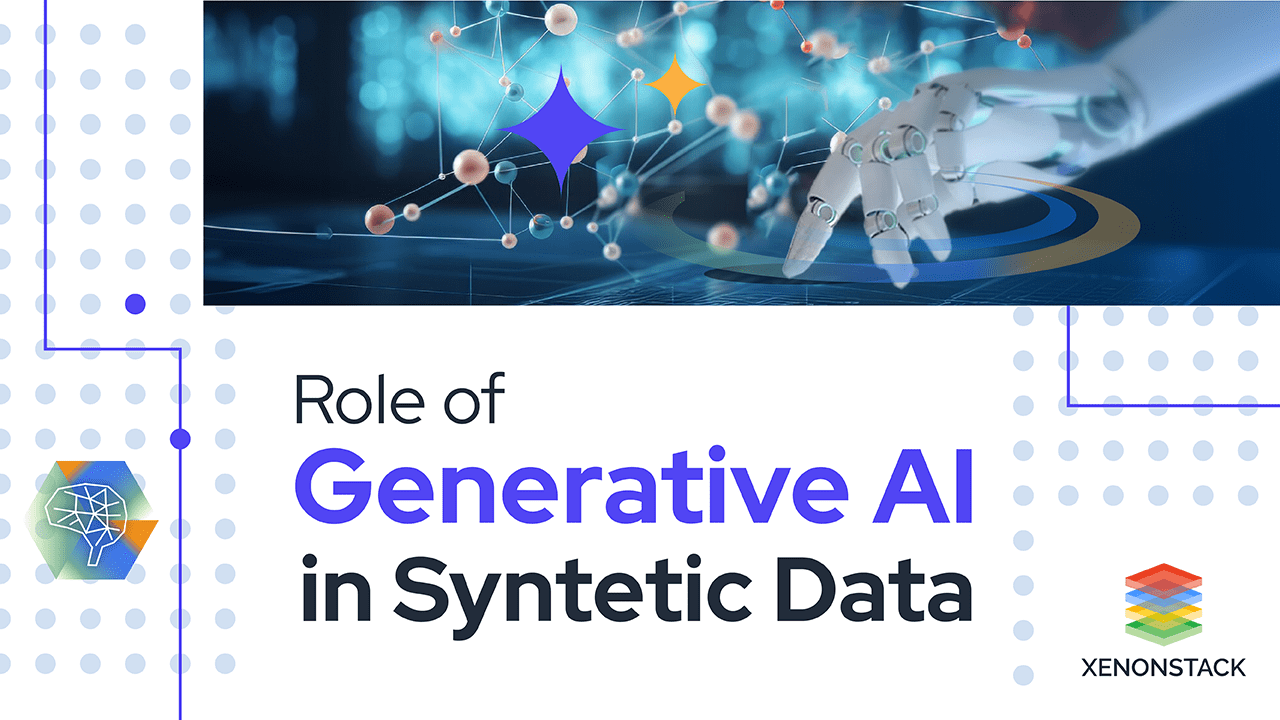
Introduction of GenAI in Synthetic Data
Synthetic data generation creates new datasets that imitate real-world or real customer data but are artificially generated. This technique involves producing tabular data points with similar statistical characteristics and patterns as the original data while ensuring no sensitive or confidential information is disclosed.
The emergence of accurate synthetic data generation is revolutionizing multiple domains, ranging from machine learning and data analysis to privacy-focused research. Its applications are extensive and diverse, making it a crucial tool for companies seeking innovative solutions. However, many organizations encounter difficulties harnessing generative artificial intelligence (AI) solutions due to data-related issues.
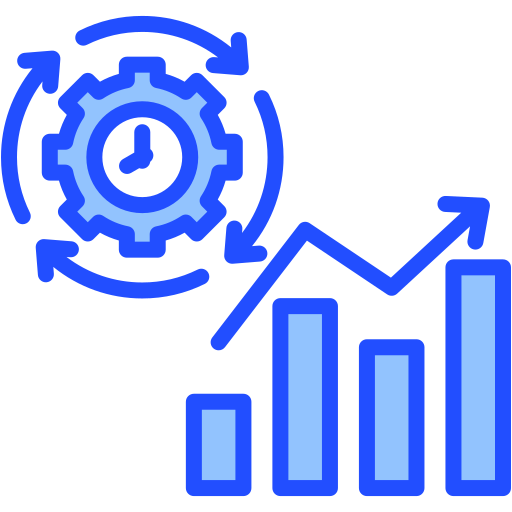
Market Growth Rate
The Synthetic Data Generation Market is expected to experience a substantial 43.13% growth from 2023 to 2027
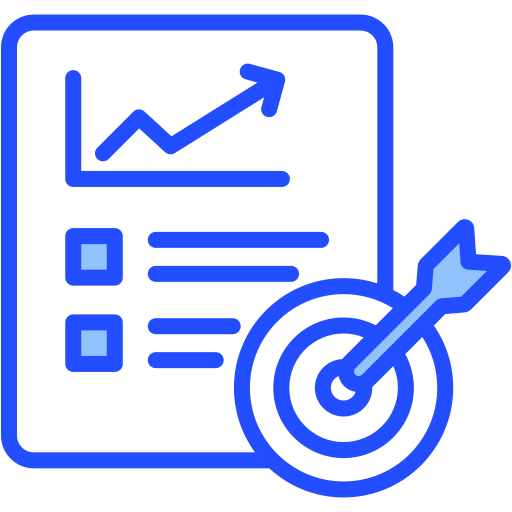
Projected Market Size
The market size is projected to increase by USD 1,072 million during the same perio
Synthetic data is a form of data that mimics the real-world patterns generated through machine learning algorithms.
Current Challenges with Real Data
Companies often struggle to implement AI due to data-related challenges. These challenges can be attributed to data regulations, sensitivity, financial implications, and scarcity.
1. Data Regulations
Data regulations are in place to safeguard individual privacy, but they can limit the types and amounts of data available for developing AI systems.
2. Sensitive Data
Since many AI applications involve sensitive customer data, protecting privacy becomes crucial, which requires careful anonymization, a complex and costly process.
3. Financial Implications
Violating regulations can have severe financial consequences, further complicating matters.
4. Data Availability
The difficulty hinders the advancement in crafting potent Agentic AI models in obtaining significant quantities of high-quality historical data for training. This creates a challenge that can be overcome using Synthetic Data. This is where synthetic data comes into play, offering a valuable solution.
Synthetic data is a tool for creating complex and varied datasets that are like real-world data but without any personal information, reducing the risk of violating compliance regulations. Moreover, synthetic data can be produced whenever required, addressing the problem of data scarcity and enabling more effective AI model training.
Benefits of synthetic data across various domains
Here is a table summarising the uses and benefits of synthetic data across various domains:
Here is the data rephrased and presented in table form:
Use Case | Application of Synthetic Data | Advantage |
---|---|---|
1. PII Data Protection | Sharing datasets without revealing PII. | Ensures compliance with privacy regulations like GDPR or HIPAA. Conducts analyses, model development, and hypothesis validation. Reduces the risk of exposing sensitive individual information. |
2. Machine Learning and AI | Enhancing datasets in limited or non-diverse scenarios. | Improves training of machine learning models by introducing diverse data points. Augments training datasets for model robustness. Enhances model performance, especially in data-restricted situations. |
3. Testing | Testing data-centric applications (data pipelines, algorithms, software). | Validates the performance and resilience of various system components. Creates specific test cases and scenarios. Assists in assessing system performance and resilience in edge cases and outliers. |
4. Data Augmentation | Expanding training datasets in fields like computer vision. | Increases dataset size and diversity, aiding in model training. Generates additional data points. It improves model generalization, reduces overfitting, and enhances performance on new data. |
5. Anonymisation and Data Sharing | Replacing original datasets for external sharing. | Allows data sharing while preserving individual privacy. Maintains statistical properties in shared data. Enables external analyses without accessing sensitive information. |
6. Algorithm Development | Developing and benchmarking algorithms with known characteristics and labels. | Facilitates algorithm development and refinement. Compares algorithmic performance and establishes benchmarks—aids in identifying algorithm strengths and weaknesses and standardizing benchmarks for specific tasks. |
This table provides an overview of how synthetic data is used in various sectors, highlighting its importance in maintaining privacy, enhancing machine learning models, testing, data augmentation, and algorithm development.
1. Data Protection and Privacy: By generating synthetic datasets that exclude personally identifiable information and sensitive data, user privacy is effectively safeguarded. These datasets can be used for research and development purposes.Use of Gen AI Models in Generating Synthetic Data
2. Data Augmentation: Moreover, generative models provide the advantage of generating novel training data that can significantly improve real-world datasets. This method is especially beneficial when obtaining more real data is expensive or time-consuming.
3. Generative Models: Generative models can also help address the problem of imbalanced datasets. By providing synthetic examples of underrepresented classes, they can boost the performance and fairness of models.
4. Imbalanced Data: In cases where anonymization is required, generative models can replace sensitive information with synthetic but statistically equivalent values. This allows for data exchange for research or compliance purposes without disclosing confidential information.
5. Testing and Debugging: Generative models can also assist in testing and troubleshooting software systems. Synthetic data can be generated for this purpose without exposing actual data to any potential dangers or vulnerabilities.
6. Data Availability and Accessibility: In situations where access to authentic data is constrained or inadequate, generative models offer a viable solution, enabling researchers and developers to manipulate data representations for their research or applications.
How Does Generative AI Produce Synthetic Data?
Generating synthetic data is possible with the help of Deep Machine Learning (ML) generative models, such as Generative Pre-trained Transformer (GPT) methodology, Generative Adversarial Networks (GANs), and Variational Auto-Encoders (VAEs). These algorithms are designed to learn from existing data and produce new synthetic instances that closely resemble the original dataset.
-
GPT is a language model extensively trained on tabular data. It can generate synthetic tabular data that is very similar to real-life data. Tools that use GPT for synthetic data generation rely on the model's ability to understand and replicate patterns from the training data. This makes it useful for augmenting tabular datasets and generating realistic data for ML tasks.
-
GANs, conversely, are a type of neural network that includes both a "generator" and a "discriminator." The generator produces synthetic data that resembles real data, while the discriminator effectively differentiates between genuine and synthetic data. Synthetic data provides a secure avenue for researchers and organizations to share datasets without compromising the privacy of personally identifiable information (PII).
In the training process, the generator and discriminator compete to produce synthetic data that convincingly mimics real data, fooling even the most sophisticated models. Over time, this process creates a high-quality synthetic dataset that closely resembles real data.
-
Finally, VAEs use an "encoder" and a "decoder" to generate synthetic data. The encoder summarizes the characteristics and patterns of real-world data, while the decoder tries to convert that summary into a synthetic dataset that is very similar to real data. VAEs can generate fictitious rows of tabular data that follow the same rules as real data.
Click here to know about the how future of generative AI made significant strides in various domains, including image generation, video game development, education, and machine learning.
Strategy to Protect Businesses from Ethical Implications
Businesses interested in generative AI must navigate several ethical implications, but these risks can be minimized with careful planning and mitigation strategies. In this regard, let us explore potential pitfalls and how to avoid them.
1. Misinformation and Deepfakes
Generative AI's ability to produce content that blurs the lines between reality and fabrication is alarming. From synthetic news to manipulated videos, these creations can distort public perception, fuel propaganda, and damage the reputation of both individuals and organizations. The risks of fake content can be mitigated by investing in developing and deploying tools to identify and remove it.
2. Bias and Discrimination
Generative models can perpetuate societal biases if trained on biased datasets. This can draw legal repercussions and cause brand damage. Organizations must prioritize diversity in training datasets to avoid these issues and commit to periodic audits to check for unintended biases.
3. Copyright and Intellectual Property
The ability of generative AI to craft content that mirrors existing copyrighted materials poses significant legal concerns. Intellectual property infringements can result in costly legal battles and reputational damage. To avoid such risks, businesses must ensure that training content is licensed and transparently outlines how generated content was produced.
4. Privacy and Data Security
Generative models, particularly those trained on personal data, pose privacy risks. The unauthorized use of this data or the generation of eerily accurate synthetic profiles is a significant concern. Companies can lean towards anonymizing data when training models.
Generative AI helps reduce the risk of errors as it detects inconsistencies associated with bank and finances. Read about Generative AI in Banking and Finance Industry.
Applications of Using Synthetic Data
Synthetic data generation has several applications across different domains, including
1. PII Data protection
-
Synthetic data allows researchers and organizations to effortlessly exchange datasets while preserving the confidentiality of personally identifiable information (PII).
-
Researchers are free to conduct in-depth analyses, develop cutting-edge models, and validate hypotheses without jeopardizing the privacy and security of sensitive individual information.
2. Machine Learning and AI
-
Synthetic data is precious in scenarios where the original dataset is limited or lacks diversity. It helps in training machine learning models more effectively by introducing additional data points that cover a broader range of scenarios.
-
The augmentation of training datasets with synthetic data enhances model robustness and performance, especially when collecting large, diverse, or real-world data is challenging.
3. Testing
-
Synthetic data is used to test and validate various components of Data-Centric Applications, such as data processing pipelines, algorithms, and software applications.
-
Developers can create specific test cases, edge scenarios, or outliers to assess the performance and resilience of their systems.
4. Data Augmentation
-
In fields like computer vision, synthetic data is employed to expand the size and diversity of training datasets.
-
Synthetic data, which generates supplementary data points, is vital in enhancing model generalization, preventing overfitting, and boosting performance on uncharted data.
5. Anonymization and Data Sharing
-
Synthetic data is a secure and confidential alternative to original datasets, empowering organizations to confidently share information externally while safeguarding individual privacy.
-
Synthetic data maintains statistical properties and relationships, allowing external parties to analyze without accessing sensitive information.
6. Algorithm Development
-
Synthetic datasets with known characteristics and ground truth labels are used to develop and benchmark new algorithms.
-
Researchers can compare algorithmic performance, identify strengths and weaknesses, and establish benchmark datasets for specific tasks, fostering advancements in the field.
Conclusion
Synthetic data can come close to resembling accurate data, but it may only capture some of the intricate details and complexities found in the original dataset. Therefore, thorough evaluation and testing are necessary to ensure the synthetic data accurately represents the real-world scenarios it aims to mimic. Additionally, the specific implementation details and choice of generative AI model may vary depending on the task, dataset, and application requirements. Different variations of GANs, VAEs, or other generative models may be more appropriate for different scenarios.
- Explore the Log Analytics with Generative AI
- Click here to learn about our ITOps Observability with Generative AI