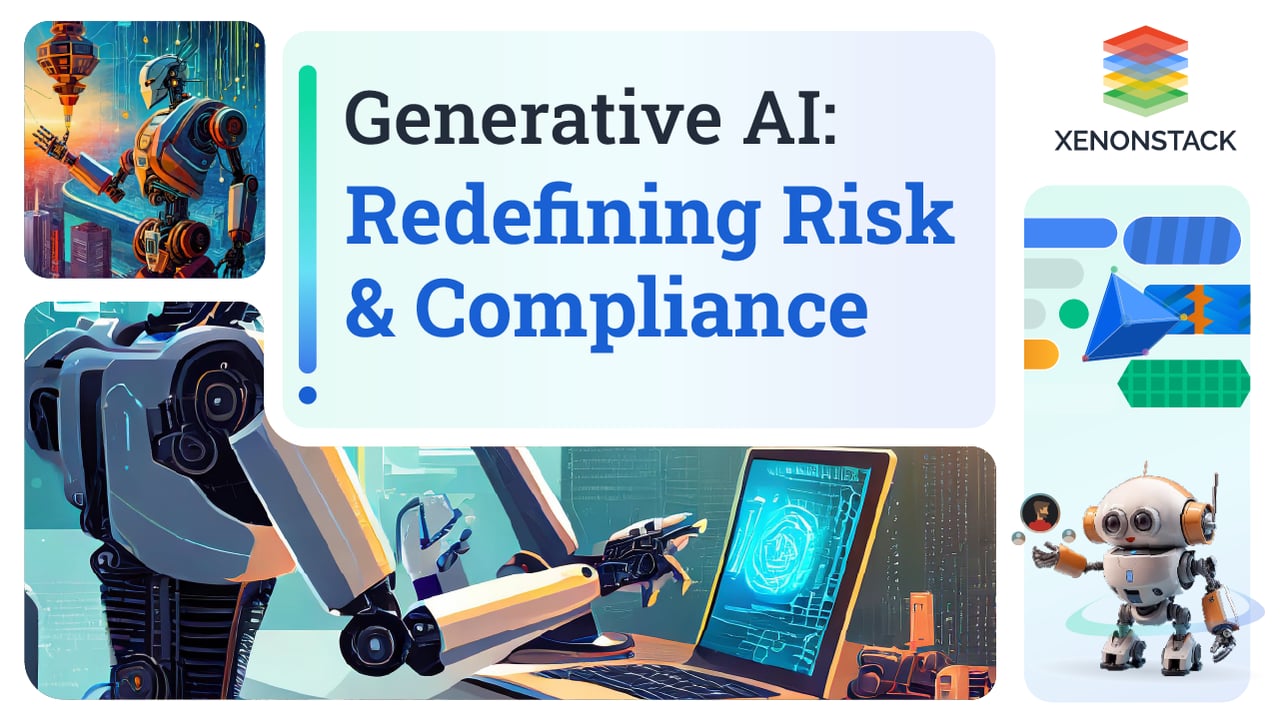
Compliance for Credit & Security Risks with Generative AI
Generative AI can revolutionize compliance assessments by significantly enhancing efficiency and accuracy in multiple facets of compliance management. Through advanced Natural Language Processing (NLP) techniques, generative AI technologies utilize extensive training on compliance-related language and legal texts, offering a sophisticated understanding of regulatory requirements and standards. This enables the swift generation of crucial compliance documents, such as policy manuals, compliance reports, and risk assessments, tailored to meet specific regulatory guidelines.
In analyzing regulatory documents, generative AI models are trained with domain-specific data to navigate the complexities of regulatory texts. They identify essential compliance requirements and legislative changes, providing concise summaries and actionable insights. This deep analysis helps organizations stay on top of regulatory updates and understand the impact of policy-making changes on their operations.
-
Answering compliance-related queries: Generative AI-powered chatbots can provide immediate assistance. By integrating with regulatory databases and organizational policies, these chatbots can offer real-time answers to questions about compliance procedures and regulatory requirements, streamlining the compliance process.
-
Contract review and analysis: Generative AI, fine-tuned on legal and compliance terminology, can scrutinize legal contracts for compliance with regulations and organizational standards. It highlights discrepancies and potential risks, ensuring that contracts adhere to predefined compliance criteria.
-
Customer communications: Generative AI ensures that interactions follow industry-specific regulations and privacy laws, automating the creation of compliant and personalized communications. This not only mitigates the risk of regulatory violations but also enhances customer trust.
-
In the critical areas: In the area of Anti-Money Laundering (AML) and Know Your Customer (KYC) compliance, generative AI facilitates the automation of report generation and risk identification by analyzing transaction data and customer information. This enhances the detection of suspicious activities and ensures regulatory compliance.
-
Privacy: generative AI can automate the creation of privacy notices, consent forms, and responses to Data Subject Access Requests (DSARs), ensuring adherence to data protection regulations like GDPR and CCPA.
-
Automated compliance audits: Automated compliance audits benefit from generative AI's ability to generate comprehensive audit plans and reports, analyzing data to flag non-compliance issues, thereby improving the accuracy and efficiency of audits.
-
In risk assessment and scenario modeling: Generative AI leverages historical data and external factors to simulate various compliance scenarios, aiding in proactive risk management and compliance strategy formulation.
-
Content filtering and moderation: Generative AI models trained on diverse datasets can identify and moderate content in compliance with regulatory guidelines and community standards, ensuring a safe and compliant digital environment.
Generative AI expedites compliance assessments and transforms them into a more streamlined, accurate, and cost-effective process, proving invaluable across various industries and regulatory environments.
Benefits of Generative AI for Risk Management
- Rapid Analysis and Reporting: Generative AI significantly accelerates the process of analyzing regulatory documents and generating compliance reports, making it possible to assess the impact of regulatory changes swiftly.
- Consistent Compliance Checks: Generative AI ensures that compliance checks are performed uniformly, maintaining consistency across assessments and audits.
- Advanced Risk Assessment: Generative AI can sift through extensive datasets, pinpointing and forecasting potential risks, thereby enabling proactive risk management.
- Real-time Regulatory Updates: AI systems can monitor and alert about regulatory changes in real-time, ensuring that organizations remain up to date with compliance requirements.
- Decision-making Assistance: By providing detailed analyses and recommendations, generative AI aids in making informed decisions regarding compliance strategies and risk mitigation measures.
- Strengthened Cyber Security Measures: In the realm of cybersecurity, generative AI enhances the ability to detect and respond to sophisticated threats through continuous monitoring and predictive analytics.
- AML/KYC Compliance: AI enhances anti-money laundering (AML) and know-your-customer (KYC) processes by automating the detection of suspicious activities and improving customer verification processes.
- Vendor Risk Assessment: It can also automate the assessment of third-party vendors, identifying potential risks in the supply chain or service delivery.
- Continuous Improvement: As generative AI models learn and adapt over time, they offer ongoing improvements in risk and compliance processes, ensuring that organizations stay at the forefront of best practices.
- Strategic Insights: The insights generated by AI can reveal new opportunities for enhancing efficiency, reducing risks, and achieving a competitive advantage in the market.
Use cases for Generative AI for Risk Management
Zbrain
Problem: Frequently relying on manual procedures and fragmented data, resulting in inefficiencies, heightened risks, and delays in compliance processes.
Solution: Enhance the efficiency of risk assessment processes and compliance processes. It leads to a significant decrease in manual time from about 34 hours to approximately 5 hours.
-
The dataset goes through Exploratory Data Analysis (EDA) before being converted into embeddings.
-
Simultaneously, a user query is handled by the ZBrain API, leading to a ZBrain Flow.
-
The ZBrain Flow, combined with embeddings, helps create a Prompt Template in an LLM Chain.
-
The LLM Chain employs OpenAI and possibly Azure to produce output.
- The generated output is analyzed to generate a final report.
Key Impacts:
-
Saves time by automating tasks, reducing manual work from 34 to 5 hours.
-
Enables quick responses to emerging risks.
-
Enhances decision-making with informed insights.
-
Improves efficiency in risk assessment.
-
Facilitates effective risk mitigation strategies.
-
Elevates overall risk management practices for stability and success in financial operations
KPMG
Problem: Struggled with resource management, inefficient resource allocation, and slow handling of alerts
Solution: A trusted AI framework helps ensure that AI implementation and usage are ethical, trustworthy, and responsible for early alert detection and solution.
-
Business data undergoes analysis using an AI model.
-
The AI model utilizes an underlying model infrastructure for data processing.
-
After analysis, the AI model issues risk alerts and offers solutions to mitigate identified risks.
-
This scenario exemplifies a standard AI-powered risk management system.
-
Privacy: AI solutions adhere to privacy laws and regulations.
-
Fairness: Designed to minimize bias against individuals, communities, or groups.
-
Transparency: Responsible disclosure for stakeholders to understand the AI lifecycle.
-
Data Integrity: Data acquisition complies with laws and regulations.
Points to be considered
-
Data Privacy and Security
-
Ensuring confidentiality and integrity of sensitive data used by generative AI models.
-
Protecting AI models from cyber threats to prevent data breaches.
-
Model Transparency and Explainability
-
Addressing the challenge of understanding decisions made by AI systems.
-
Ensuring compliance with regulations by enabling auditing of AI processes.
-
Dependence on Quality and Quantity of Data
-
Data Quality: Ensuring accuracy by using high-quality and unbiased training data.
-
Data Scarcity: Addressing limitations in data availability for compliance area.
-
Integration with Existing Systems and Processes
-
Compatibility Challenges: Overcoming obstacles in integrating AI with current infrastructure.
- Use of Generative Agents in Security Operations
-
Change Management: Implementing organizational adjustments for AI adoption in compliance workflows.
-
Ethical Considerations and Public Perception
-
Ethical Use: Upholding ethical standards in AI applications to prevent adverse impacts.
-
Public Trust: Building confidence among stakeholders regarding AI's ethical and responsible use.
Conclusion for Generative AI
Generative AI offers a promising avenue for enhancing risk and compliance management; its successful implementation hinges on addressing the inherent challenges and ethical considerations associated with AI technologies. Organizations can leverage generative AI to comply with regulatory requirements and gain a competitive edge through innovation and improved risk management by adopting a balanced approach that emphasises governance, transparency, ethics, and continuous learning.
As businesses navigate the complexities of regulatory compliance, data privacy concerns, and cybersecurity threats, the adoption of generative AI presents a promising solution to streamline operations, drive efficiency, and ensure regulatory requirements. By embracing generative AI and leveraging its advanced capabilities, organizations can enhance risk management processes and position themselves for sustained growth, resilience, and competitive advantage in an increasingly dynamic and regulated environment.
- Read here about SOC 2 Compliance and its Best Practices
- Explore about RPA Risk Management and its Various Methods