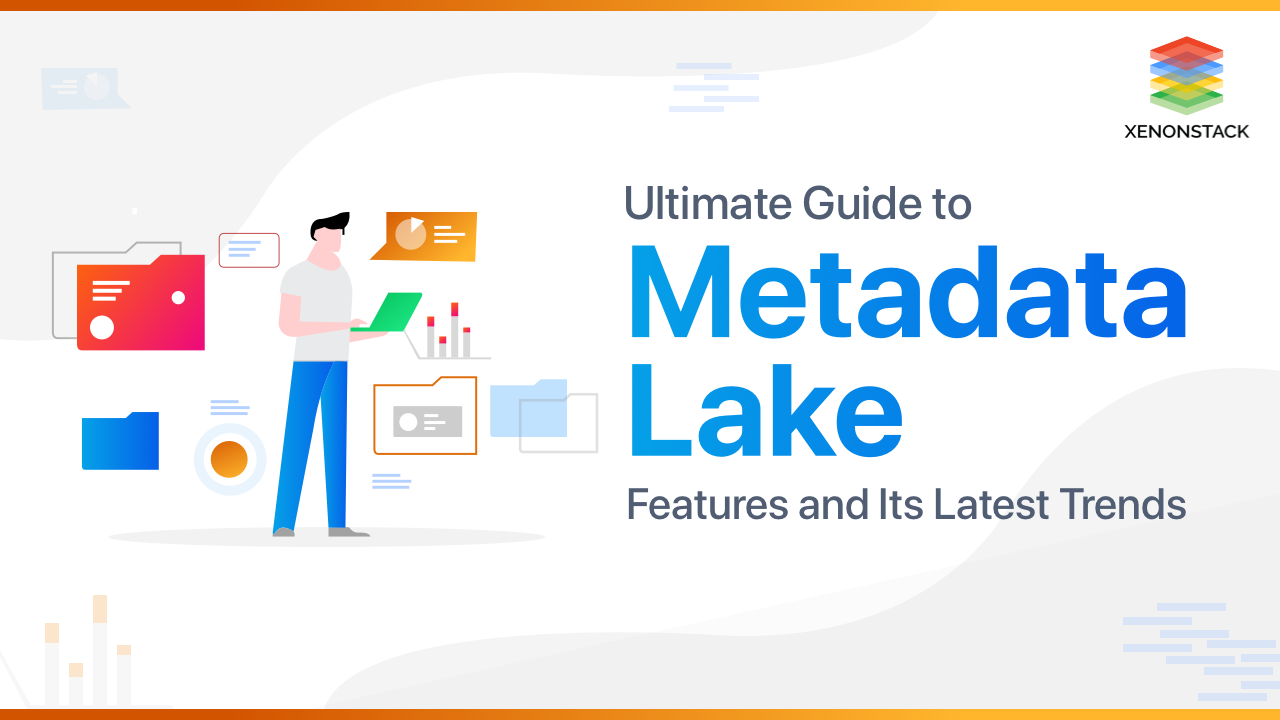
Introduction to Metadata Lake
We know we are already overwhelmed with data. Whether the use cases of the data, whether the tools and technologies which are used for those use cases and if coming to the people using those tools for working on those technologies for realizing those use cases they are more diverse than ever — data engineers, data scientists, business analysts, analysts, analytics engineers, product managers, citizen data scientists and so on with each of them having their roles and responsibilities. These data users all work with such advanced tools day in day out like data lakes, data warehouses, databases, BI tools, notebooks, streams, data visualization tools, etc.
Despite all this, the one thing that keeps getting missing from the data universe is context. That is where Metadata gets in.
The data lakehouse is an upgraded version of the data lake that taps its advantages, such as openness and cost-effectiveness while mitigating its weaknesses. Click to explore about, Data Lake vs Warehouse vs Data Lake House
From data taking all the highlights and yet not serving the context on its own, now Metadata is here to share its limelight by offering to be - a single source of truth.
Collecting metadata that can effectively serve different teams with their unified context of data, tools, and processes can resolve the purpose of their use cases much quicker. But what is Metadata, you ask? It's easily guessable that Metadata is “data about data”.
Now when Metadata is data about data, how can someone think it would be easy to manage metadata in the same space. This is where the Metadata asked for its own space giving origin to metadata lake.
How is Metadata Lake itself becoming Big Data?
Despite not getting the attention, metadata was there since always but wasn’t getting its enough share. Now more the data, more the Metadata.
Modern data stack’s every nook and corner generates Metadata. Besides just basic Metadata that we can think of like technical Metadata (e.g, schemas) and business metadata(e.g. glossary, taxonomy), different systems now create Metadata that can be categorized into entirely new forms of Metadata -
- Cloud compute ecosystems, and orchestration engines generate logs every second, called performance metadata.
- Social Metadata developed with users interact with the data assets and with one another.
- Logs from BI tools, notebooks, and other applications and communication tools like Slack generate usage metadata.
- Orchestration engines generate provenance metadata.
Living data systems are creating all these new forms of metadata. This has led to an explosion of metadata in size and scale. This is the time when Metadata has already begun its journey to becoming big data itself.
Serverless Cloud Computing enables self-service provisioning and management of Servers. Click to explore about, Serverless Solutions and Architecture for Big Data, and Data Lake
Why Metadata Lake is important?
Well, if talking about the importance of a data lake that we are already familiar with, then these are set up for serving different use cases and users at the same time with access to unlimited data that has limitless potential. Of Course, instead of storing data in ‘some best form’ for the latter, a data lake is single, massive storage to store data of all kinds, be it structured, unstructured, and in both its raw and processed forms. Data Lakes serve a purpose from various use cases and users from analytics to data science to machine learning.
This is exactly where we are when it comes to the potential and need of metadata storage and its use cases and users. More than ever, Metadata is being collected, and where comes the need to store it in a place that can serve the same purpose as a data lake? Then why not give Metadata its lake to live in. Metadata lake will serve as a unified repository to store all kinds of metadata in raw and processed forms and also can be used to drive both the use cases known as far and which can come up tomorrow.
Use Cases of Metadata Lake
In real-life examples and problems, metadata lake will act as a centralized repository for storing the extracted, processed, visualized metadata, etc., from various tools and pipeline workflows to be useful enough to power some recently floated use cases. Modern Metadata Lake architectural components -
- As a central repository for saving the data -
The modern data stacks that we use today under different roles and uses can ultimately save their data here in the metadata lake as a centralized repository.
Below are the domains which make use of the different tools, technologies, and platforms and must be able to save data into metadata lake-
- Data Ingestion - Fivetran, Singer, Stitch
- Data Warehouse - BigQuery, RedShift, Snowflake
- Data Lake - Databricks, Delta Lake, S3
- Data Transformation - ETL process, dbt, Matillion, R/Python + Apache Airflow
- BI - Looker, Mode, Tableau
- Data Science - Anaconda, Dataiku, Domino
- As a central repository for powering relative use cases -
When considering the cases where this Metadata is going to be used to do the
wonders and which need storage from where the data can be used for
realizing those technologies. The use cases are mentioned below -
- Data discovery
- Metrics repository
- Data Observability
- Data Lineage & RCA
- Auto-Tuned Data Pipelines
A Data Lake is a secured centralized repository that stores data in its original form, ready for analysis. Click to explore about, Governed Data Lake | The Advanced Guide
What are the expected features of a metadata lake?
When we see a new storage type coming up with term data in it, we know they serve different purposes and have different use cases though their names are similar. The features expected from the metadata lake are slightly far more than just being a data lake. Let's look into them -
Support of Knowledge Graphs
The true potential of Metadata lies in recognizing the connection between the data assets. This is what knowledge graphs are used for. Knowledge graphs are the most effective way for these interconnections to be stored. When knowledge graphs will be able to integrate with Metadata properly and create relationships, the use cases like metadata discovery can serve so many users so easily.
Serving as open APIs and interfaces
When accessing the data, we want it to be easily accessible, so is the case with the Metadata. It is accessible not just as a data store but via an open API. This is where its power of becoming the single source of truth at every stage of the modern data stack comes in. It would become easy to access metadata for use cases like integrating the Metadata for the dashboards. Metadata powers metadata provenance and metadata lineage to get better results on data observability. Similarly is the case with the data discovery, which comes in hand when Metadata is leveraged properly. Considering the use cases that metadata can serve, it must be taken into account that the flexibility of the use cases must be served in the future.
Flexibility in basic Architecture
For empowering both humans by serving as a base for the data discovery and helping in understanding the context and resolving the purpose of machines and tools by assisting the auto-tuning data pipelines, metadata must serve the purpose for both human intelligence and automatic work of machines and tools.
The fundamental architecture for metadata lakes must reflect this flexibility as a reality.
What are the latest trends in Metadata lake?
As Metadata is occupying its space slowly in the data world, the intelligence we can derive from it increases day by day as more use cases are coming up simultaneously. Today the data-driven organizations have only scratched the surface of the use cases served with Metadata. But using metadata to its fullest is yet being awaited, and to realize it can fundamentally change how our data system operates.
With every new requirement coming up on data is going to increase the pressure and load back to Metadata. Considering the following scenarios coming up in the future where -
- Where system leverages past logs to automatically tune data pipelines and optimize compute performance by reshuffling loads based on the data asset usage stats and optimizing the schedule pipelines.
- Data Quality issues come up in a source table, and the downstream system automatically stops the pipelines to ensure that incorrect data does not process any further or make its way to the dashboard. They are predicting the data quality failures and fixing them without any human intervention.
These are concepts that are being worked upon. Recently technologies like Data Mesh, Data Fabric, and DataOps have created a buzz. However, these concepts are fundamentally based on being able to collect, store and analyze Metadata.
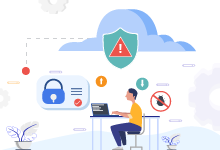
Conclusion
The deeper we get into the world of Metadata, the more pronounced the concept of metadata lake is manifested. Metadata lake will be the cornerstone for the new changes and innovations in data management. It wouldn't be a surprise if tomorrow a metadata lake solution is just introduced in the market. The only thing that will be awaited then is a whole new big metadata world taking rise and companies being powered based on this concept of metadata lake, revolutionizing the analytics and machine learning industry.
- Discover more about GCP Data Catalog - A Guide to Metadata Management
- Click to explore Data Lake Services for Real-Time and Streaming Analytics