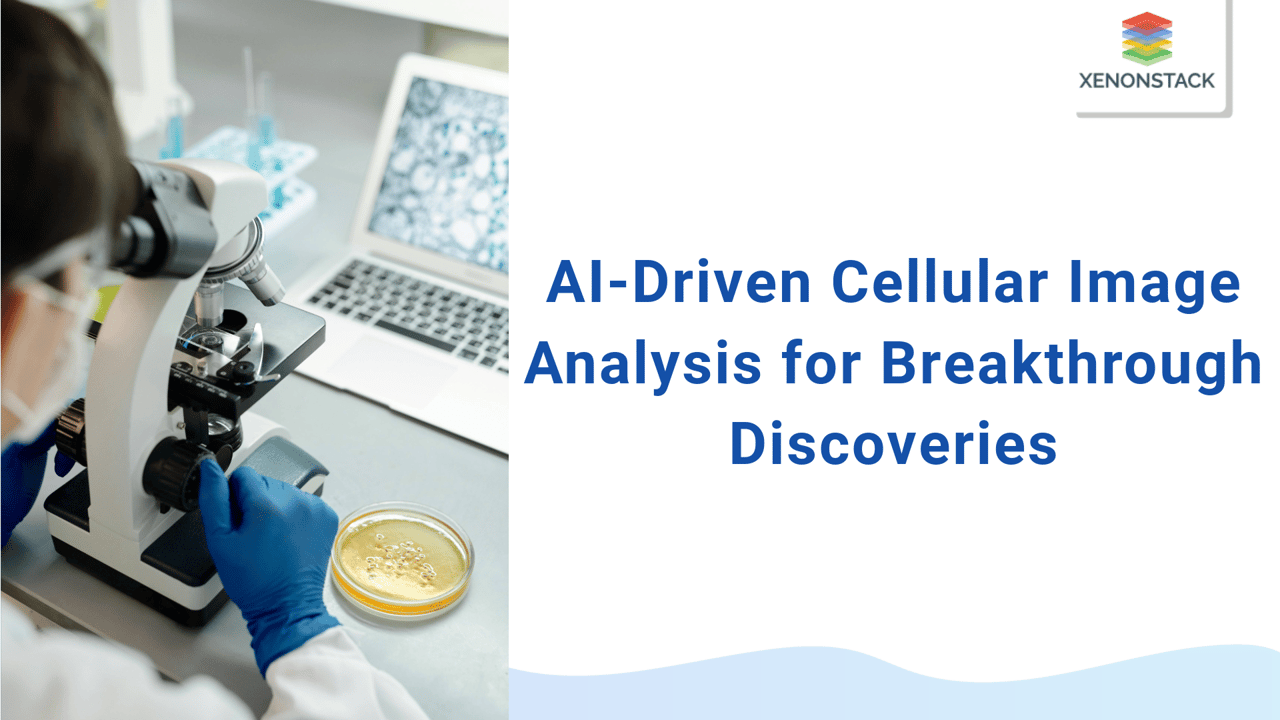
Light and electron microscopy and other cellular-level imaging have provided us with unprecedented magnification and resolution necessary to deal with the structural complexity defined at living organisms' cellular and sub-cellular levels. Nevertheless, many years ago, the hugely increased amount and variety of images created a problem for traditional analytical methods. AI is the perfect solution where researchers and clinicians can benefit from abilities that have never been possible in analysing microscopic and cellular images.
The Evolution of Microscopic Imaging
Microscopy has been essential in biomedical investigations for many years. The compound microscope, discovered in the 16th century, and modern fluorescence, confocal, and electron microscopy have expanded their capacities, offering researchers avenues to viewing samples based on structures and at the subcellular and molecular levels.
However, these advancements come with their own set of challenges:
-
Volume of Data: Dental high-resolution imaging creates image files in terabytes, which is monumental for manual analysis tools.
-
Subjectivity: Interpretation Was performed manually; it brings variability, creating potential inconsistency problems.
-
Complexity: Complex evaluation of living cells' dynamic processes or fine phenotype shifts is beyond human capacity.
Here, these challenges are addressed by automating analysis, enhancing precision, and identifying data points that may not have been discovered when analyzing the data manually.
How AI Transforms Microscopic Image Analysis
AI-powered tools enhance the analysis of microscopic images in several key ways:
Automated Image Segmentation
Segmentation isolates some areas of interest, whether cells, organelles, or tissue structures in the image. Conventionally, manual segmentation can be tedious, and the results may not be consistent. Specifically, CNN-based AI models can accurately and rapidly segment the epithelial tissues of interest areas.
Applications:
-
Segmentation of neurons in bright field images of brain tissue.
-
Automated detection of regions of interest in histological images for carcinoma identification.
Advancements:
State-of-the-art deep learning networks, including U-Net and Mask R-CNN, have set a standard of results with high similarity and almost no variability.
Feature Extraction and Quantification
AI is especially useful here as it can read images in ways the human eye cannot easily contain, such as texture, shape, intensity, spatial position, or arrangement. These features are applied and presented quantitatively to explain biological processes better and make hypotheses.
Applications:
-
That is, quantifying mitochondrial size and mitochondrial density within cells to evaluate the health of a given cell.
-
Protein colocalization in fluorescence microscopy: quantitative methods.
Advancements:
CNNs and autoencoders take raw images, extract hierarchical features, and provide information at different levels of biological organization.
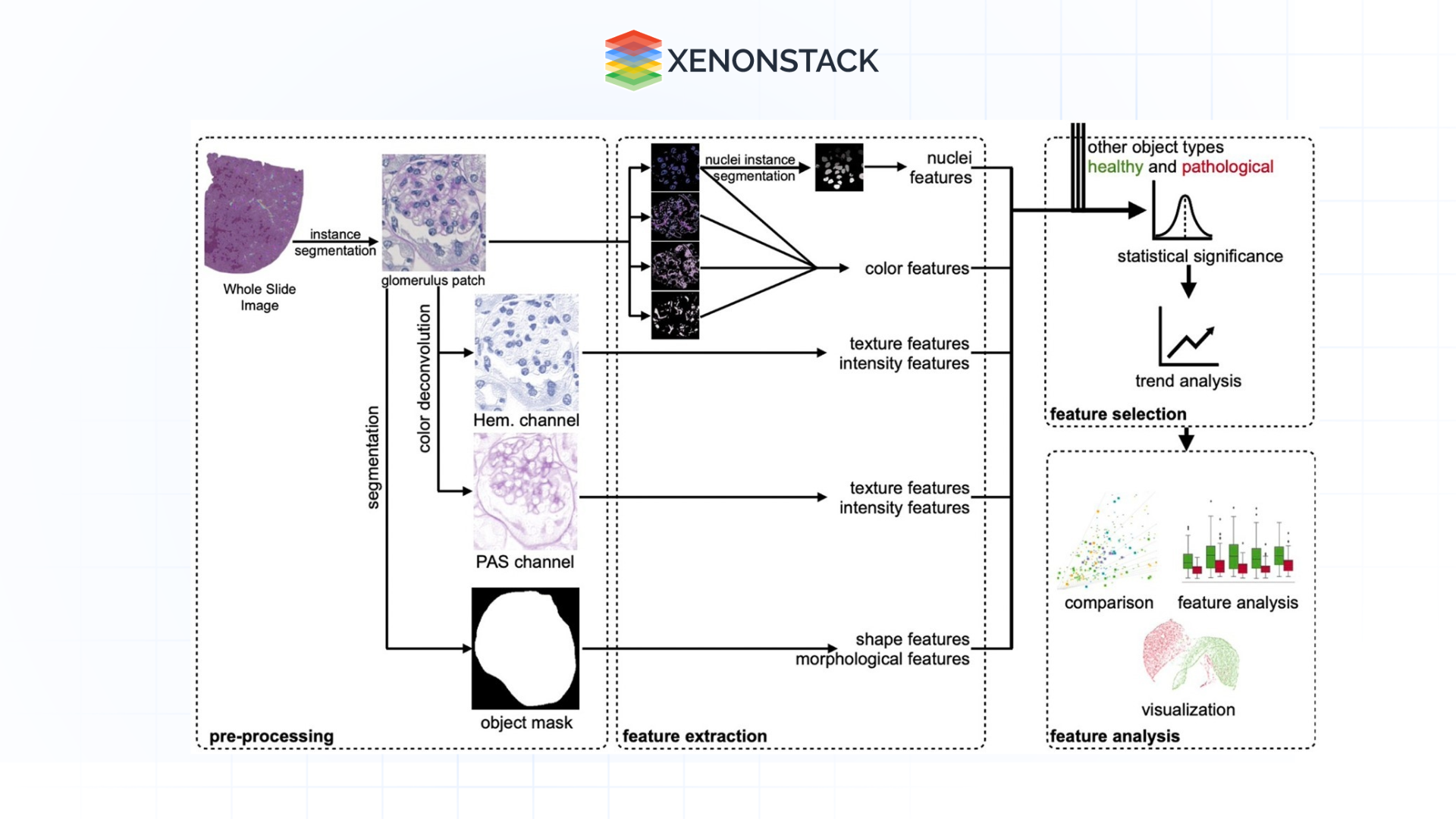
Predictive Analytics and Phenotyping
Machine learning can then use the patterns identified on cells to forecast biological outcomes, including drug sensitivity or resistance, disease status, or toxicity. This capability is particularly useful in a research and clinical context enterprise ai architecture.
Applications:
-
Phenotypic screening of drugs using cellular characteristics.
-
Automating the detection of tumour aggressiveness from its histopathological images.
Advancements:
GANs and transformers mimic the biological response, thereby minimizing the usage of physical experiments.
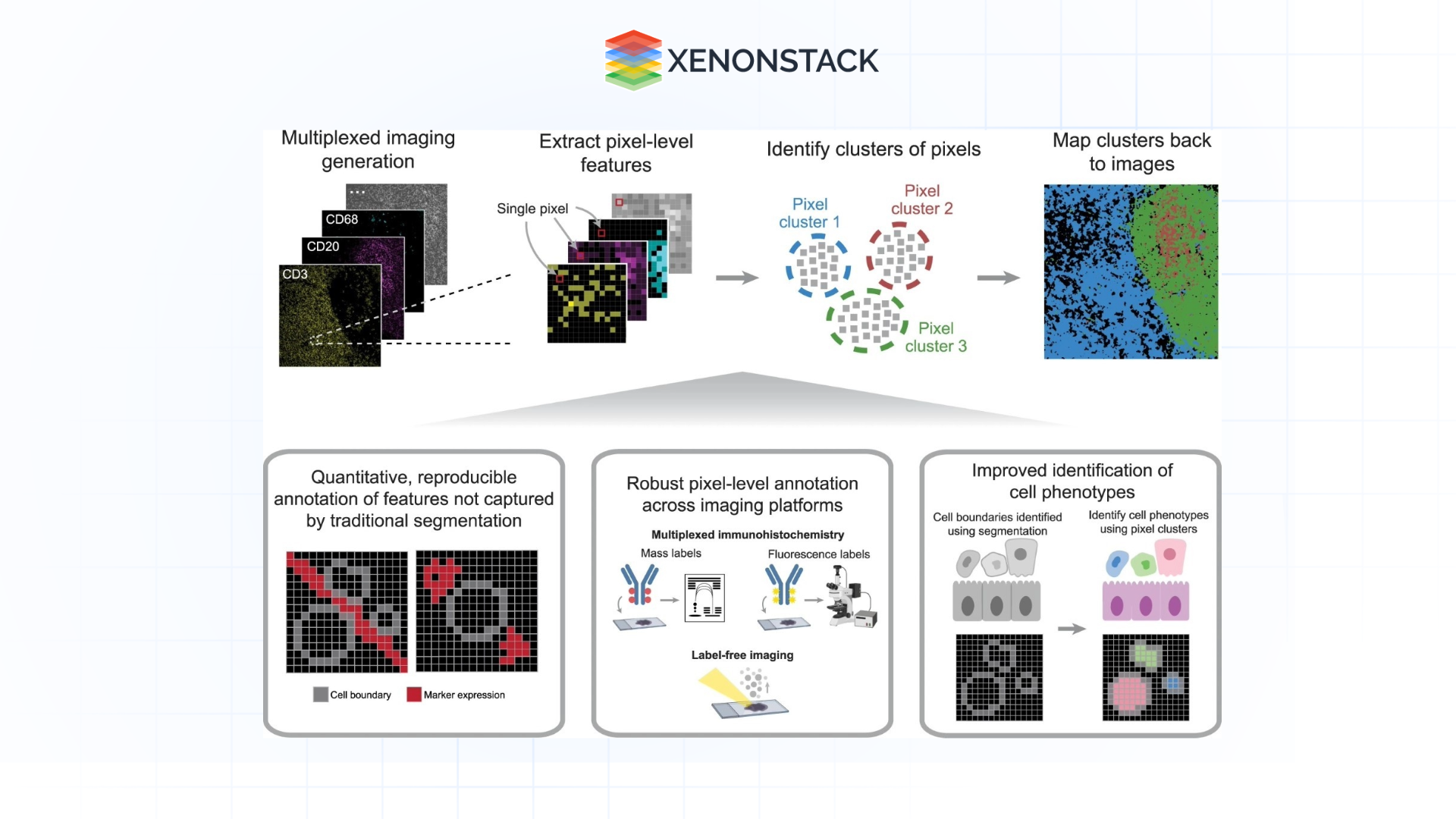
Biological Monitoring of Cellular Actions
AI permits examining functioning cells by handling enhanced microscopic images throughout the examination process.
Applications:
-
Examining the process of movement of immune cells during an infection.
-
Predict the movement of organelles and vesicles within living cells.
Advancements:
With the help of time-lapse imaging and subsequent analysis using AI algorithms, a series of pictures provides both high speed and acceptable accuracy.
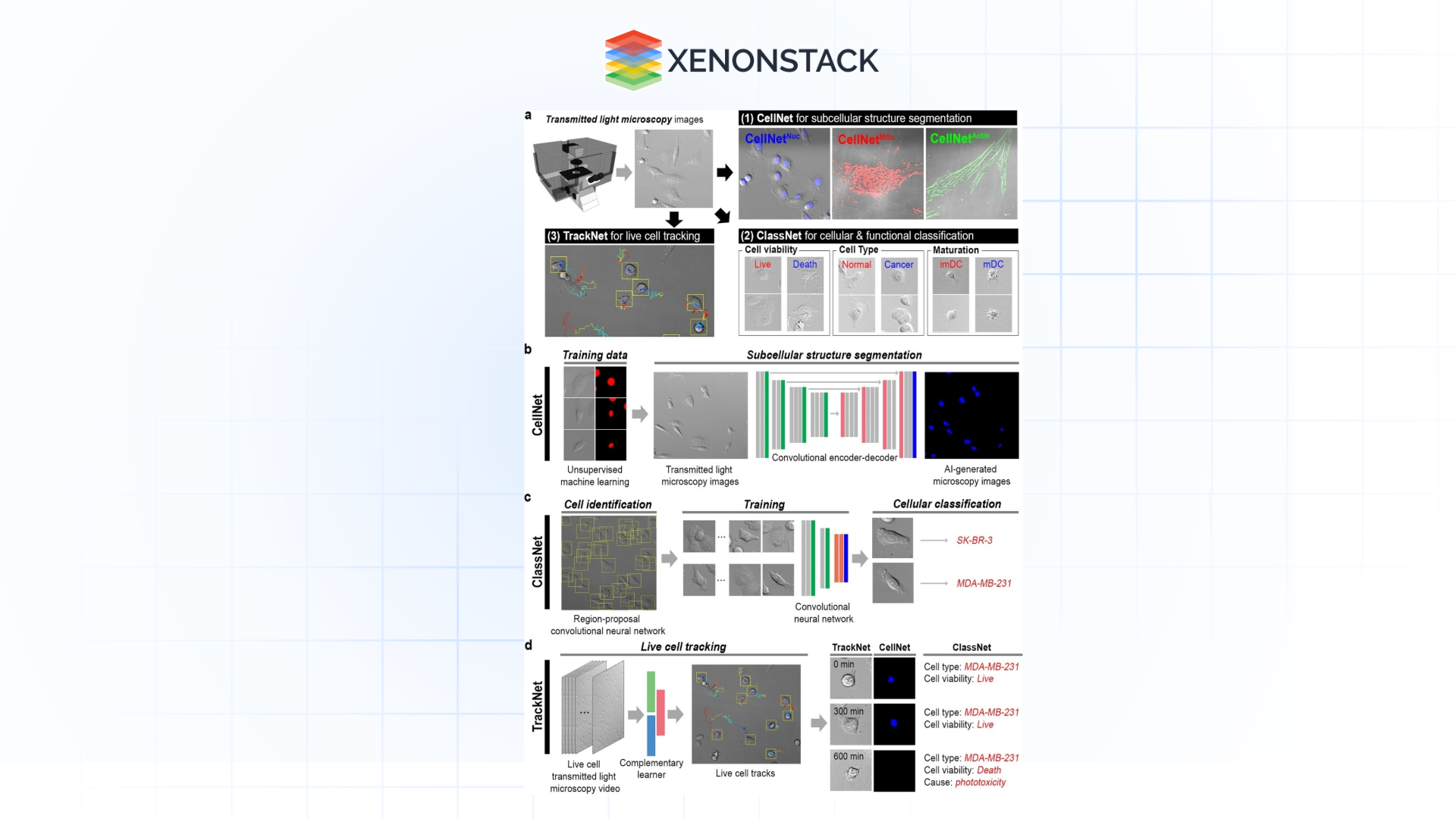
Multi-Modal Analysis
AI applies the integration of data from different imaging modes, such as fluorescent and electron microscopy, with other data, including genomic and proteomic data. This approach makes it easier to learn complex biological systems since all organization levels are covered.
Applications:
-
From the morphology to gene expression: mapping.
-
Exploring the interactions between different proteins in cell layout.
AI solutions in the identification of Microscopic Images
Pathology and Diagnostics
Diseases are usually diagnosed by identifying various tissue samples under the microscope. AI greatly expands this process when interpreted as automated, bias-free, and fast diagnostic assistance.
-
Example: AI models are used to diagnose early-stage cancer from histopathology slides with an efficiency of prediction that is equal to, or even better than, that of human pathologists.
-
Benefits: Fewer misdiagnosis, improved time, and cost efficiency while being flexible to understaffed environments.
Drug Discovery and Development
Drug identification is based on how cells respond to cure-related treatments. AI helps to shorten this process by performing high-throughput screening, identifying patterns in cellular phenotypes, and predicting drug efficiency.
-
Example: Assessing the impact of thousands of chemicals on liver cells to detect hepatotoxic hazards.
-
Benefits: Combining the two models increases accuracy and efficiency in target success rates and the cost and time effect.
Neuroscience
Neuronal morphology and connectivity diagrams are essential in unravelling the brain's anatomy and pathologies. In this case, AI helps to assess the relationships between multiple types of neuronal networks.
-
Example: Topographical wiring of neural connections for analysing neurological disorders such as Alzheimer’s.
-
Benefits: Knowledge about the development of disease and possible targets for treatment
Microbiology And Infectious Disease
AI assists with microbiology, investigating microbial associations, and dealings with hosts.
-
Example: Differentiating bacterial species in complex community structures by deep learning algorithms.
-
Benefits: Superior knowledge of position-specific microbial communities and quicker detection of organisms of concern.
The Progress that is Fuelling Progress in Microscopy
Several technological advancements underpin the success of AI in microscopy:
-
Advanced Deep Learning Models
It’s important to know that architectures such as U-Net, ResNet, and Transformers have significantly changed the approach to image analysis. Compared to the other models, these models are particularly robust for categorising, grouping, and recognising objects, making higher-level analyses possible AI-driven quality control. -
Cloud Computing, Cloud Computing and Scalability
AWS and Google Cloud are two of the most prominent cloud service providers that can scale up the processing and analysis of large imaging datasets. They also provide features for implementing an AI model in an operational environment. -
Explainable AI (XAI)
To be decisive, transparency is important in areas such as diagnostics. XAI techniques increase the understanding of AI model decision-making and improve trust between researchers and clinicians. -
Federated Learning
Federated learning allows training new and enhanced AI models on distributed datasets without the exchange of data to prevent data violations and maintain data privacy policies, such as GDPR and HIPAA.
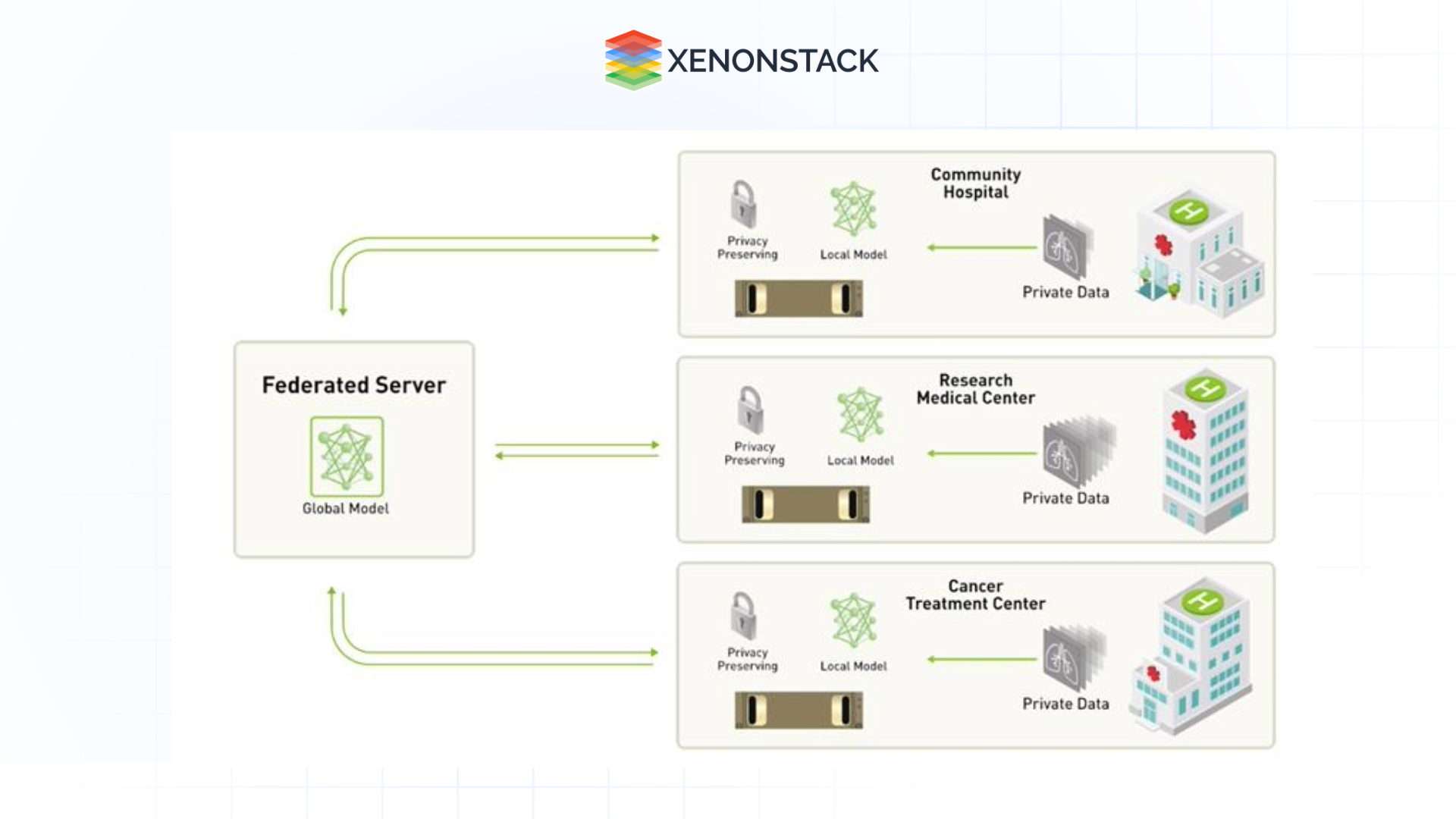
Issues In Microscopy: An Analytical Approach Based on Artificial Intelligence
Despite its transformative potential, AI in microscopy faces several challenges:
-
Data Quality and Annotation
Training in AI models requires high-quality annotated datasets. However, generating these datasets is time-consuming and demands understanding the specified context.
-
Model Generalization
They lack robustness due to variations in imaging conditions and methods on which AI models trained on specific datasets are developed.
-
Ethical and Regulatory Requirements
Employing AI in delicate contexts like health care requires the applicator to always work within particular standards of ethical practices and legal requirements.
Future Trend of AI Microscopy
The future of AI in microscopy promises exciting developments:
-
Multi-Modal Data Integration
Imaging data integrated with other data types will generate comprehensive descriptions of biological systems, for example, through genomics.
-
Real-time analysis with Edge AI
Using AI models on microscopes and other edge devices will make decisions in real-time, easing word flow in laboratories and clinics.
-
Fully Automated Laboratories
AI automation for imaging techniques, analysis, and reporting will cut human interaction for results in half to enhance discoveries.
Conclusion
AI has moved the bar in microscopic and cellular image analysis, permitting new tools for research and clinical communities. AI can perform complex tasks and improve and accelerate the quality of research and its provision of real-time data, allowing researchers and clinicians to unlock new dimensions of biological exploration.
Considering further progression in technology, where issues such as data quality and generalization of the model are being tackled, AI will advance to become increasingly core to microscopy. In diagnosis, drug discovery, neuroscience, and microbiology, incorporating AI will raise the world's level of discovery in the microscopic world, bringing more solutions into the health sector.
Next Steps with Microscopic and Cellular Images
Connect with our experts to explore implementing advanced AI systems and how industries and departments leverage Decision Intelligence to become more insight-driven. Discover how AI enhances the analysis of microscopic and cellular images, automating workflows, improving accuracy, and accelerating research and diagnostics.