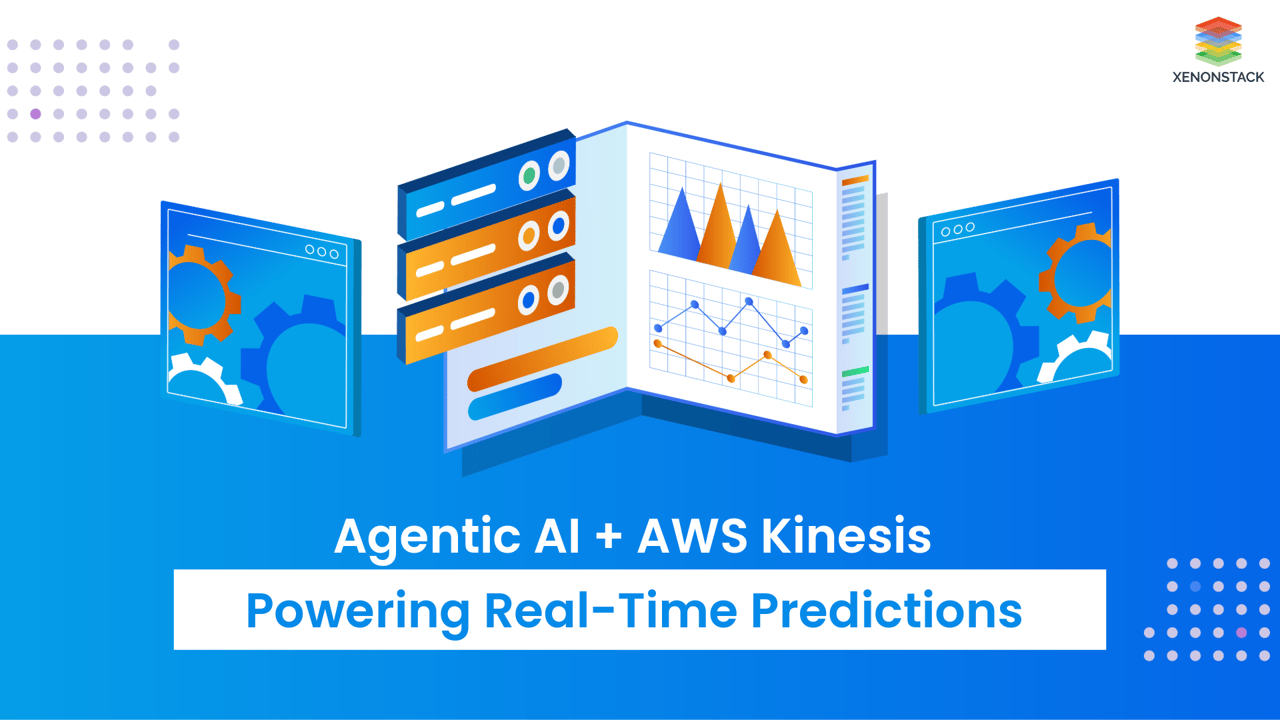
Challenges When Integrating Agentic AI with AWS Kinesis
While the combination of AWS Kinesis and Agentic AI offers immense potential, several challenges must be addressed.
-
Data Privacy and Security - Real-time data streaming involves the transmission of sensitive information. Ensuring data privacy and security requires the implementation of encryption, secure access protocols, and compliance with data protection regulations like GDPR or HIPAA.
-
Model Accuracy and Latency - Ensuring that predictive models remain accurate while minimizing latency can be a delicate balance. Real-time predictions require models that are both precise and fast, which can be challenging to achieve, especially as the volume and complexity of the data increase.
-
Scalability and Cost Management - As the scale of data grows, both the AWS infrastructure and the AI models must scale accordingly. Managing the cost of data processing, storage, and model deployment is a key concern that organizations must address to avoid excessive expenditure.
Discover how Akira AI Agents power autonomous operations with intelligent decision-making.
- Agent Analyst – Transforms data into actionable insights for smarter business strategies.
- Agent Force – Automates workflows and enhances operational efficiency across teams.
- Agent SRE – Ensures system reliability with proactive monitoring and self-healing capabilities.
Use Cases for Real-Time Predictive Analytics with AWS Kinesis and Agentic AI
Several industries stand to benefit significantly from integrating AWS Kinesis with Agentic AI:
1. E-Commerce Personalization
In e-commerce settings, companies can utilize real-time analytics to personalize customer experiences dynamically:
-
By analyzing user behavior during browsing sessions—such as clicks, or time spent on product pages—merchants can provide tailored recommendations or targeted promotions instantly.
-
This not only enhances customer satisfaction but also increases conversion rates by delivering relevant content at the right moment.
2. Financial Services and Fraud Detection
Financial institutions leverage predictive analytics powered by real-time streaming data to combat fraud effectively:
-
By analyzing transaction patterns in real time using Agentic AI models trained on historical fraud cases, banks can identify potentially fraudulent transactions as they occur.
-
Immediate alerts allow institutions to take preventive actions quickly—such as freezing accounts or flagging transactions for review—thereby minimizing losses.
3. Predictive Maintenance in Manufacturing
Manufacturers can use real-time predictive analytics to optimize maintenance schedules:
-
By collecting sensor data from machinery through AWS Kinesis and applying Agentic AI algorithms to predict equipment failures before they occur, companies can schedule maintenance proactively.
-
This approach reduces downtime significantly while extending the lifespan of machinery through timely interventions.
Benefits of Real-Time Predictive Analytics
The advantages of adopting real-time predictive analytics are manifold:
1. Enhanced Decision-Making
Access to real-time insights empowers organizations to make informed decisions quickly:
-
Leaders can respond proactively rather than reactively when trends emerge, or anomalies occur.
-
Enhanced decision-making capabilities lead directly to improved business outcomes across various functions—from marketing strategies to operational efficiencies.
2. Operational Efficiency
Automating responses based on predictive analytics streamlines operations:
-
Organizations can reduce manual interventions by implementing automated workflows triggered by specific predictions (e.g., restocking inventory when sales exceed thresholds).
-
Increased efficiency not only lowers operational costs but also improves overall productivity levels within teams.
3. Competitive Advantage
Organizations leveraging real-time analytics gain a significant edge over competitors:
-
The ability to act swiftly based on current market conditions or consumer behaviours positions businesses favourably against those relying solely on historical analyses.
-
Companies that harness these technologies effectively are better equipped to anticipate market shifts or consumer preferences ahead of their rivals.
Future Trends in Real-Time Predictive Analytics
Looking ahead into the future landscape of real-time predictive analytics reveals several emerging trends:
-
Increased Automation through AI: As machine learning algorithms become more sophisticated, automation will play a larger role in decision-making processes across industries.
-
Greater Emphasis on Data Privacy: With rising concerns about privacy violations and regulatory compliance requirements increasing globally (e.g., GDPR), organizations will need robust frameworks for managing sensitive information responsibly while still deriving valuable insights from it.
-
Advancements in Machine Learning Algorithms: Continuous improvements in algorithm efficiency will enable even faster processing times while enhancing accuracy levels—making it possible for businesses across sectors—from healthcare providers predicting patient outcomes accurately based on live health metrics—to leverage these capabilities effectively without incurring excessive costs or resource burdens.
Kinesis and AI: Transforming Business Today
The integration of AWS Kinesis with Agentic AI for real-time predictive analytics represents a powerful tool for businesses seeking to gain insights from live data. By enabling accurate and timely predictions, this technology provides the foundation for smarter decision-making, enhanced operational efficiency, and a competitive edge in various industries. As the technology continues to evolve, it promises even greater potential for real-time innovation.