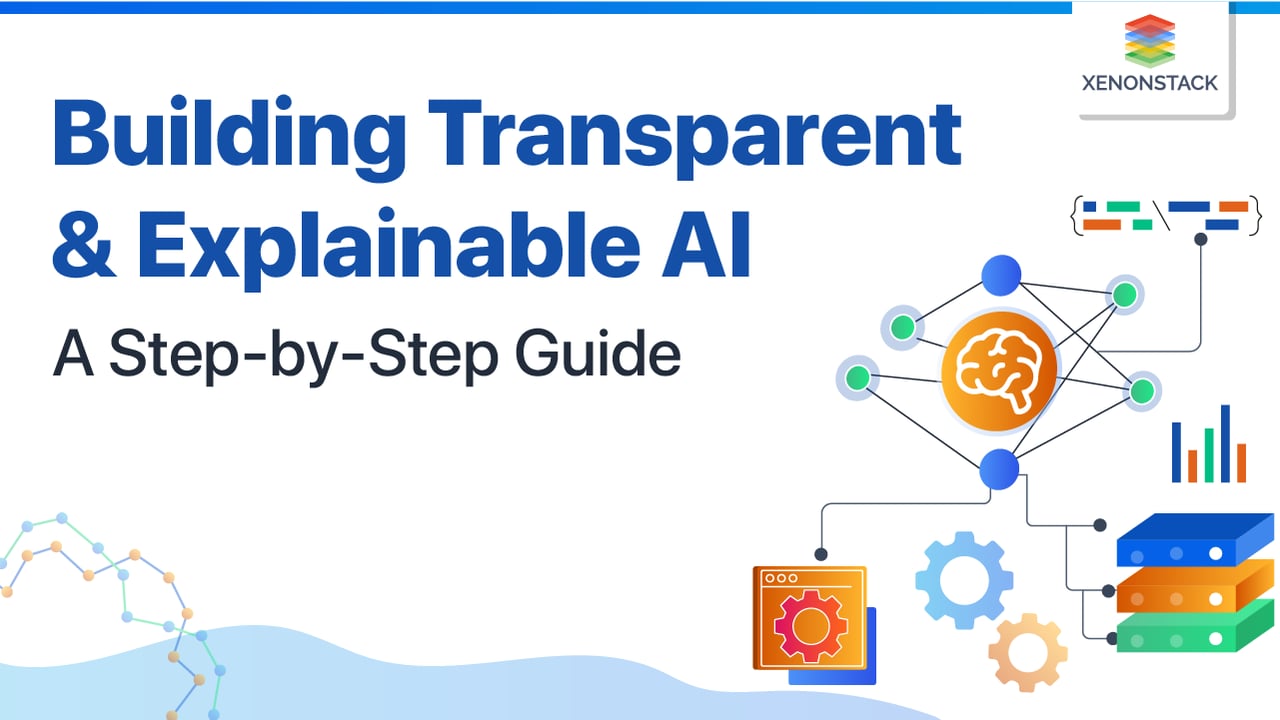
Introduction to Transparent and Explainable AI
The term "black box" in the context of artificial intelligence (AI) refers to the lack of transparency or interpretability of a machine learning model's decision-making process. A black box model is one where the inputs and outputs are observable, but the inner workings or decision-making processes are opaque and not easily understood by humans.
While black box models can achieve high levels of accuracy and efficiency, they are often criticized for being difficult to interpret, explain, or audit. This is particularly concerning in fields where healthcare, finance, or law enforcement decisions can have significant consequences. Researchers are developing more transparent AI models to address these concerns. Some approaches include developing models to supply visualizations of their decision-making processes, using techniques such as attention mechanisms or saliency maps. Others involve designing inherently interpretable models, such as decision trees or rule-based systems.
A way to develop machine learning techniques and technologies that produce more explainable models. Taken From Article, Explainable Artificial Intelligence (XAI)
Why Explainable AI is important?
Machine learning algorithms are quickly becoming the basis of almost everything online, from home assistants to predictive music recommendations. The difficulty humans face in understanding the reasoning behind AI decisions is well-known, though there are multiple ways of explaining AI decisions. Explainable Artificial Intelligence (XAI) aims to make AI decision-making understandable for humans and machines alike. And its 'interpretability' aspect must be included when any machine learning algorithm is used. XAI has many advantages over existing explanations, especially when the tasks involve inductive reasoning or extensive abstraction.
Explainable Artificial Intelligence is becoming more critical for AI systems because it prompts research into developing better explanations for the decisions made by AI systems. The main reason is that humans tend to find it difficult to understand why an Artificial Intelligence system has decided. Thus, explainable Artificial Intelligence provides an opportunity to develop new valuable technologies and help us create better AI systems in the future.
What is Explainable AI?
Explainable Artificial Intelligence (or XAI) is an emerging field that integrates techniques in machine learning, statistics, cognitive science, and object-oriented programming. It aims to create artificially intelligent systems that people can understand through explanations rather than relying on high-level rules.
What is the goal of Explainable AI?
XAI systems aim to supply insights and explanations about how the AI model arrived at its decision. XAI aims to make AI more transparent and interpretable to humans, particularly in complex decision-making scenarios.
Many AI models are traditionally considered "black boxes" because humans find them challenging to understand and interpret. However, in many real-world applications, it is essential to understand how the AI system arrived at its decision.
Therefore, XAI systems aim to supply human-interpretable explanations of AI decisions. This can be achieved through techniques such as feature importance analysis, model visualization, and generating natural language explanations. XAI systems can help improve transparency, accountability, and trust in AI systems and enable humans to collaborate more effectively with AI systems for decision-making.
AI is a solution to the AI black box dilemma when technology needs to explain its conclusion and how it arrived at that decision. Taken From Article, Transparent AI Challenges and Its Solutions
How does Explainable AI make AI Transparent?
XAI makes AI transparent by supplying insights and explanations about how an AI model arrived at its decision. XAI techniques aim to make the decision-making process of AI systems more interpretable and understandable to humans, which can be crucial in various real-world applications.
There are several ways in which XAI can make AI transparent:
-
Model visualization: XAI can use visualization techniques to help users understand how an AI model makes decisions. This can include displaying the relationships between different variables, the weights assigned to each variable, and how the model processes data.
-
Feature importance analysis: XAI can help determine which features or variables are most important in decision-making. By understanding which features are driving the decision, humans can gain insights into the underlying mechanisms of the AI model.
-
Natural language explanations: XAI can generate natural language explanations that describe how the AI model arrived at its decision. It can help make the decision-making process more interpretable and understandable to humans.
-
Counterfactual explanations: XAI can provide "what-if" scenarios that show how a decision would change if certain variables were altered. It can help users understand how sensitive the AI model is to changes in input data.
Overall, XAI techniques can help make AI more transparent by supplying insights and explanations that help humans understand AI models' decision-making processes. This can improve the trust and accountability of AI systems and enable better collaboration between humans and AI.
Demand for explainability for the fair and governable AI framework increases due to increasing biased AI systems. Click to explore about our, Explainable AI in Finance and Banking Industry
Levels of AI Transparency
The level of transparency can vary depending on the type of AI system and the specific application, but in general, there are three levels of AI transparency:
-
Black Box: A black box AI system supplies no explanation for its decision-making process, and the user has no visibility into how the system arrived at its conclusion. This is the least transparent level of AI and is often seen in complex deep learning models where the system may need to be simplified for humans to understand.
-
Gray Box: A grey box AI system supplies limited visibility into its decision-making process but needs a complete explanation. The user may be able to see specific inputs and outputs or understand some aspects of the decision-making process, but only part of the picture. This level of transparency is often seen in simpler machine-learning models.
-
White Box: A white box AI system supplies complete transparency into its decision-making process, allowing the user to understand how the system arrived at its conclusion fully. This level of transparency is often achieved through rule-based systems, decision trees, or other interpretable models.
Note that the level of transparency needed for an AI system may vary depending on the specific application.
A process to address potential issues before leading to breakdowns in operations, processes, services, or systems with AI. Click to explore about our, Explainable AI for Predictive Maintenance
How do Explainable AI/White Box models work?
XAI or "white box" models work by providing insights and explanations into how an AI model makes decisions. Unlike traditional "black box" models, which humans find difficult to interpret and understand, XAI models are designed to be transparent and interpretable.
XAI models work by incorporating techniques that enable the model to generate explanations for its decisions. Below are a few techniques of explainable AI:
Technique |
Advantages |
Limitations |
Visualization |
Simple and Effective |
It cannot give importance to factors leading to prediction. |
Logistic Regression |
Very widely used. It can give importance to factors in prediction. |
Cannot give threshold value of factors in prediction. It can be used to explain but not to advise. |
Decision Tree |
It can give a threshold value for factors in prediction. It can be used to explain as well as advice. |
The many levels in the decision tree can be complex to understand. |
Neural Network |
It can give a threshold value for factors in prediction. It can be used to explain as well as advice. |
The very high number of neural network layers can be complex to understand. |
SHAP |
Standardized approach to all models. It can be used to explain as well as advice. |
It can be compute-intensive. |
Overall, XAI or "white box" models provide transparency and interpretability to AI models. By providing insights and explanations into how the model makes decisions, XAI models can improve trust and accountability in AI systems and enable better collaboration between humans and AI.
A In the future of AI in the automotive industry, customers will prioritize vehicle function over form. Taken From Article, Responsible AI in Automotive Industry
Real-world Examples of Explainable AI in Action
It refers to transparent machine learning models that clearly understand how they arrived at their output. Here are some examples of it in action:
-
Medical Diagnosis: AI models can analyze medical images and help doctors diagnose diseases like cancer. Its models can highlight areas of concern in the image and show how they arrived at their diagnosis, providing doctors with valuable insights into their decision-making process.
-
Fraud Detection: Financial institutions use AI models to detect fraudulent transactions. Its models can help investigators understand the rationale behind the model's decision to flag a particular transaction as fraudulent, allowing them to make more informed decisions.
-
Autonomous Vehicles: Self-driving cars rely on AI models to make real-time decisions. Its models can help passengers understand how the car arrived at a particular decision, such as why it stopped suddenly or took a particular route.
-
Natural Language Processing: AI models can analyze text and extract valuable information. Its models can help researchers understand how the model arrived at its conclusions, such as which words or phrases were given more weight in the analysis.
These are just a few examples of explainable AI in action, and there are many more use cases across a wide range of industries.
What are the benefits of Explainable AI?
XAI refers to the techniques and methods used to create AI models and systems that humans can easily understand and interpret. The benefits of XAI include the following:
-
Increased trust: XAI can help increase trust in AI systems by explaining how a decision was made. People are more likely to trust the system when they understand why a decision was made.
-
Better decision-making: XAI can help humans make better decisions by giving them insights into how the AI model arrived at its decision. It can help humans find any biases or errors in the model and correct them.
-
Compliance: XAI can help organizations follow regulations and standards that require transparency and accountability in decision-making processes.
-
Improved human-AI collaboration: XAI can help improve collaboration between humans and AI systems, allowing for more effective and efficient decision-making.
-
Improved model performance: XAI can help find weaknesses and areas for improvement in AI models, leading to better performance.
Overall, XAI can help make AI more transparent, interpretable, and trustworthy, leading to AI's more effective and beneficial use in various applications.
What are the major challenges of Explainable AI?
It is a new area of research, and there are still many active challenges in Explainable models today. One of the challenges is that explainability can come at the expense of model performance accuracy, as AI systems tend to have lower performance than uninterpretable or black-box models. One of the main challenges of explainable AI is how to generate correct and understandable explanations.
Another major challenge is that explainable AI models can be more difficult to train and tune than uninterpretable machine learning models. AI systems can also be more difficult to deploy, as explainability features often require human intervention in the loop.
Conclusion
One of XAI's steps is to develop more sophisticated techniques to explain AI decisions. Currently, most XAI methods rely on generating explanations based on feature importance or sensitivity analysis. These approaches can be practical but could be improved in their ability to provide a complete picture of the decision-making process. Another area of interest for XAI is the development of methods for evaluating the quality of explanations generated by AI systems. There is yet to be a generally accepted standard for evaluating the quality of XAI annotations, making it difficult to compare different approaches and determine which works best.
The field of XAI is growing rapidly, and much research is underway to develop new techniques and methods for increasing the transparency and accountability of AI systems.
Next Steps
Talk to our experts about implementing Explainable AI systems and how industries and departments leverage transparent workflows and Decision Intelligence to make data-driven, trustworthy decisions. Utilize Explainable AI to optimize IT support and operations, enhancing efficiency, accountability, and responsiveness while fostering confidence in AI-driven insights.