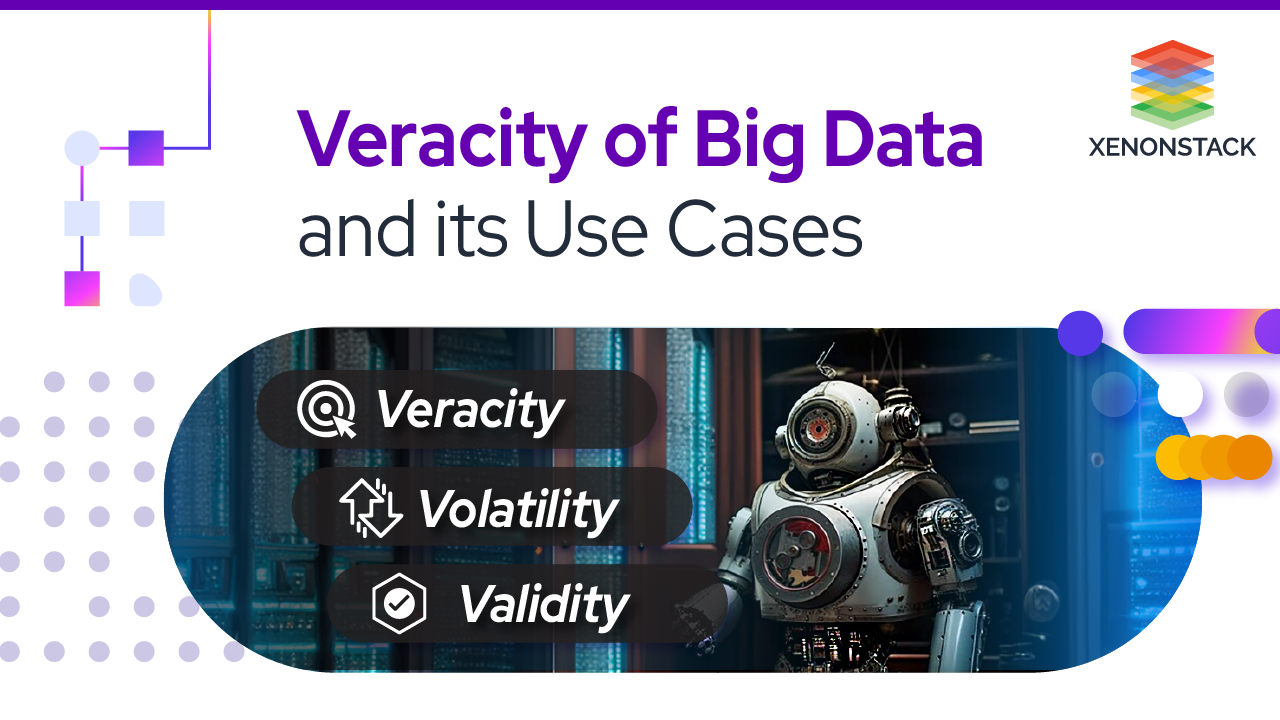
Introduction
In today's world, Big Data has become the lifeblood of businesses and analytical applications. Companies constantly strive to harness the power of all the available data to make informed decisions and develop effective strategies. In the past, analytical tools were limited to only utilizing a small portion of the data stored in relational databases, leaving behind a vast amount of untapped potential.
Big data has been renowned for its Three Vs, a triumphant trio that includes velocity, variety, and volume for years.
These V's have been the town's talk, but one more V poses a significant challenge: veracity. Veracity deals with the accuracy and quality of data, and unlike its V counterparts, it lacks a standardized approach for measurement, making it a complex and theoretical concept.: velocity, variety, and volume. However, there is one V that presents a significant challenge: veracity. Veracity pertains to the accuracy and quality of data, and unlike the other V's, it lacks a standardized approach for measurement, making it a complex and theoretical concept. Apart from 3Vs, there are four additional Vs of Data Veracity, these are:
1. Validity
2. Variety
3. Visualization
4. Value
What is Veracity in Big Data?
Veracity is a big data characteristic related to consistency, accuracy, quality, and trustworthiness. Data veracity refers to the biasedness, noise, and abnormality in data. It also refers to incomplete data or errors, outliers, and missing values. To convert this type of data into a consistent, consolidated, and united source of information creates a big challenge for the enterprise.
While enterprises’ primary focus is to use the total potential of data to derive insights, they tend to miss the problems faced by poor data governance. When we talk about the accuracy of it, it's not just about the quality of data but also depends on how trustworthy your data source and data processes are.
To illustrate the impact of data integrity, let's consider a scenario where communication efforts with customers fail to yield sales due to inaccurate customer information. When data quality is compromised or inaccurate, businesses risk targeting the wrong customers and delivering ineffective communications, ultimately resulting in revenue loss.
1. Validity
Every organization wants accurate results, and valid data is the key to making real results. Validity refers to the question, “Is the data correct and accurate for the intended use?”
2. Volatility
Volatility refers to the rate of change and lifetime of data. Organizations need to understand how long a specific type of data is valid. For example, sentiments frequently change in social media and are highly volatile. An example of low-volatile data is weather trends, which are easier to predict.
3. Volume
Volume is the amount of data collected. An analyst must decide what data to collect and how much to collect for a particular use case. To give you an idea, let’s say you have a social media platform where people post photos, review your business, watch video content, search for new content, and interact with just about anything they see on their screen. Every interaction generates information about that person you can feed into your algorithms.
Explore more in detail about data veracity.
Big data architecture refers to the logical and physical structure that dictates how high volumes of data are ingested, processed, stored, managed, and accessed
What are the sources of Data Veracity?
Veracity is the degree to which data is accurate, precise, and trustworthy. Let’s have some sources of veracity in data.
1. Biasedness: Bias or data bias is an error in which some data elements have more weightage than others, resulting in inaccurate data when an organization decides on calculated values suffering from statistical bias.
2. Bugs: Software or application bugs can transform or miscalculate the data.
3. Noise: The non-valuable data in the datasets is known as noise. High noise will increase data cleaning work as they must remove unnecessary data for better insights.
4. Abnormalities: Abnormality or Anomaly in the data is the data point that is not normal or that stands out from the actual data. For example, credit card fraud can be detected based on the amount spent.
5. Uncertainty: Uncertainty can be defined as doubt or ambiguity in the data. The uncertain data contains noise that deviates from the correct, intended, or original values.
6. Data Lineage: Organizations collect data from multiple sources, but sometimes an inaccurate source is discovered without historical reference. It would not be easy to track the data source from which it has been extracted and stored.
Learn more about tools and best practices of data lineage.
How do we ensure low data veracity?
1. Data Knowledge
To fully harness the power of data, companies need to deeply understand its origins, destinations, users, manipulators, processes, and project-specific requirements. Implementing effective data management practices and creating a comprehensive platform that provides insights into data movements is essential for success in today's business landscape.
2. Input Alignment
Consider this scenario: Imagine collecting valuable customer information through the contact details form on your website. Each field represents crucial customer details, providing valuable insights for your business. However, what happens if a customer accidentally fills in the areas incorrectly? Unfortunately, this inaccurate information becomes useless unless we proactively take the initiative to correct it ourselves.
3. Validate your source
In today's data-driven world, organizations rely on data from various sources such as IoT devices, internal databases, etc. However, organizations must validate the information and references before extracting and merging the data into their central database. This ensures that the data used for analysis and decision-making is accurate and trustworthy, leading to more reliable insights and better business outcomes.
4. Give Preference to Data Governance
Data governance encompasses a comprehensive set of procedures, responsibilities, guidelines, and measurements that guarantee the reliability and protection of data and processes employed within an organization. By implementing effective data governance practices, businesses can enhance the precision and consistency of data quality, ensuring that accurate and reliable information is available for analysis and decision-making purposes.
Get a deep knowledge of data governance.
Big data platforms utilize a combination of data management hardware and software tools to aggregate data on a massive scale, usually onto the cloud.
Use Cases of Data Veracity
Accurate and high-quality data is crucial in any industry, ensuring reliable insights and enabling data-driven decision-making. Data veracity plays a significant role in obtaining accurate results that can be trusted and relied upon.
1. Health care
Hospitals, labs, pharmaceuticals, doctors, and private healthcare centers constantly improve and identify new healthcare opportunities. The data collected and analyzed from patient records, surveys, equipment, insurance companies, and medicines bring valuable insights and daily breakthroughs in the medical industry to improve diagnostics and patient care. Using big data analytics to provide information based on evidence will help define best practices, increase efficiency, decrease costs, and many other benefits.
Data veracity is a big challenge, and the healthcare industry faces other challenges. Veracity refers to whether the data collected and insights obtained can be trusted. The Healthcare industry relies on reliable data; they can not utilize the insights derived from biased, noisy, and incomplete healthcare data. They cannot compromise with patients' health. With the help of data governance frameworks and healthcare quality standards, organizations can ensure clean, ready, unbiased, and complete data.
2. Retail
The retail industry is the best example of it. A massive amount of data is collected from products bought by customers using different modes of payment, from searching for products online, comparing them with others, and putting them in a cart. It has a lot of potential and scope to learn and improve decision-making.
Whenever a project is planned to be implemented by a retailer, what and from where the data is collected is always an important question. Equally, an important question arises, “Is the collected data trustworthy? Can I rely on this data to make important business decisions?” Correct insights from data analysis require high-quality, clean, and accurate data.
If the data is inaccurate, not up to date, or poorly organized, the veracity of big data decreases drastically. Retailers must adopt a robust validation process that enables access to data needed for data-driven decisions, keeping data integrity in mind.
How do we tackle hurdles with the help of data veracity?
In today's digital era, businesses and organizations increasingly leverage Big Data as their primary tool for development and success. However, a significant challenge they face is ensuring the quality of their data. To overcome this hurdle, companies should prioritize data veracity and implement effective data governance practices. By doing so, they can unlock the full potential of their data, enabling them to access high-quality, reliable, and fast insights that drive optimal decision-making and business outcomes.
- Explore More About Apache Hadoop
- Discover More About Big Data Visualization Tools
- Know More About big data tools in aws
Next Steps Towards Data Veracity
Ensuring data veracity involves implementing robust data governance practices, enhancing data quality through validation and cleansing processes, and leveraging advanced analytics to detect inconsistencies. Regular audits, transparent documentation, and fostering a data-driven culture also play key roles in maintaining accuracy.