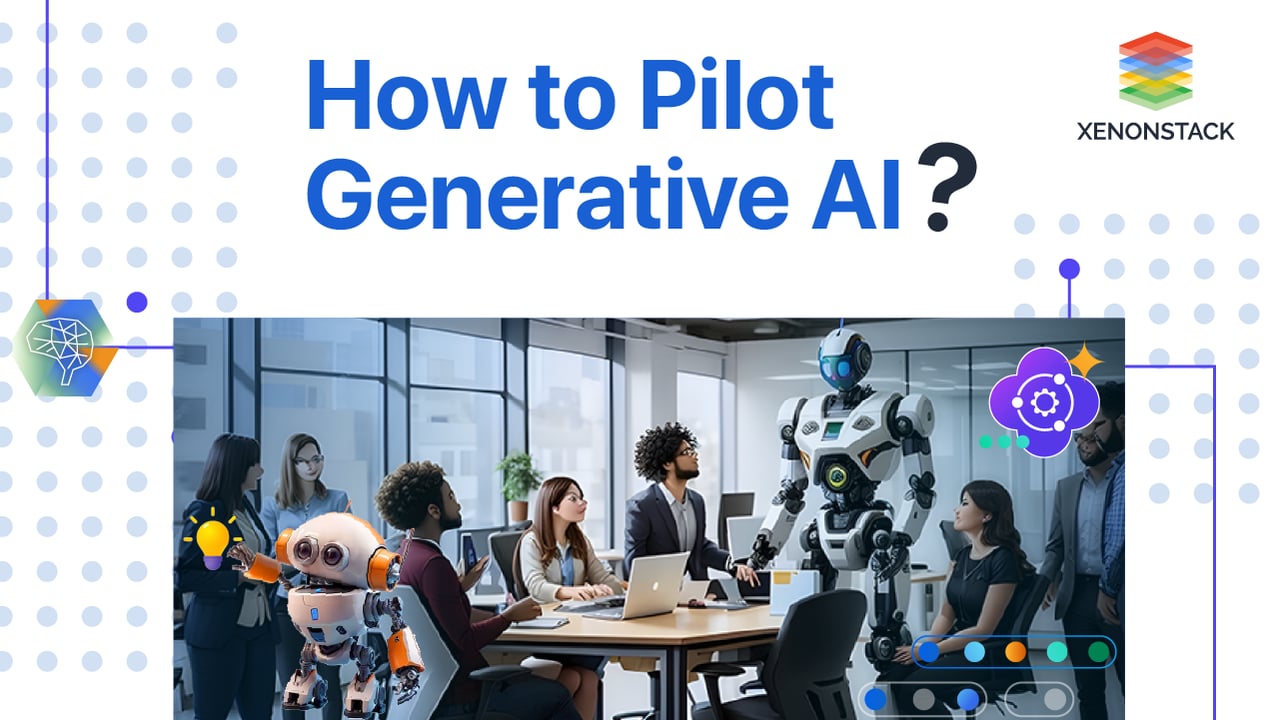
The Rise of Generative AI
As the world of artificial intelligence (AI) continues to evolve, organizations are increasingly exploring the potential of generative AI. With its ability to create new content, generate insights, and automate processes, generative AI has the potential to revolutionize the way we work. In this article, we'll explore the steps to take when piloting generative AI in your organization.
Adoption Readiness
76% of business leaders report their companies are prepared to integrate generative AI into their workflows
Increased Investment
45% of organizations have boosted AI investments following the launch of ChatGPT
What is Generative AI?
Generative AI is a type of AI that uses machine learning algorithms to generate new content, such as text, images, music, or code. Generative AI opened up new areas and enabled broader opportunities with multimodal and multilingual AI for different Data types and artifacts, including text, code, images, video, music, speech, and designs (e.g., 3D, parts, and buildings).
AI will improve human collaboration and relationships by transforming the lives of people with special needs. Humanoid robots will be new companions, and AI Agents will be new teammates, which enhance human intelligence.
The Emergence of Data as the New MOAT
Data has become an unparalleled asset, fueling predictive insights, personalized experiences, and unprecedented efficiencies. As we navigate this data-dense realm, Sovereign AI has emerged as a potent ally, tailored for an era where responsiveness, adaptability, and intelligence define market leadership.
Potential Use Cases of GenerativeAI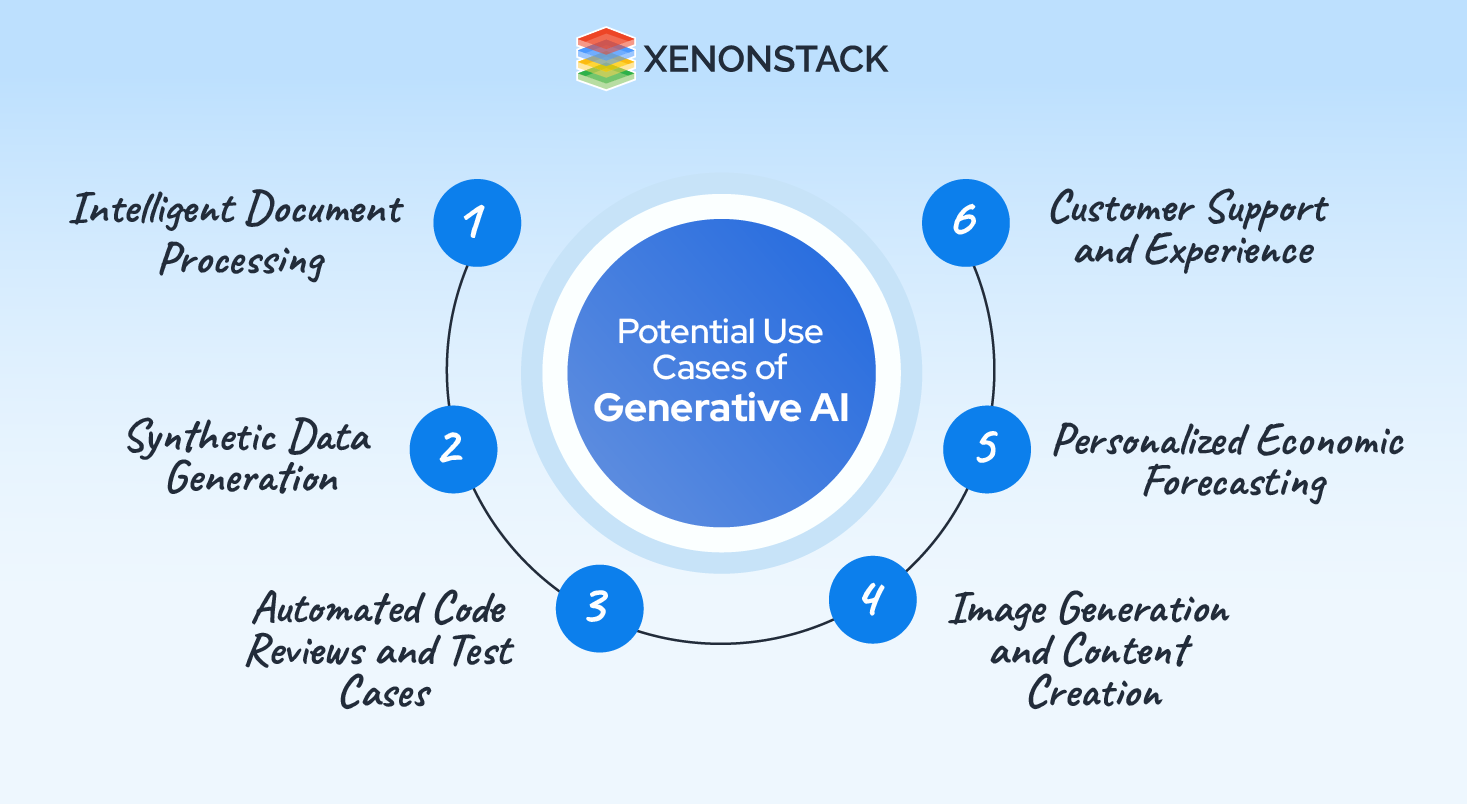
From automating loan application reviews to generating personalized economic forecasts, generative AI has the potential to transform various industries. Here are some potential use cases:
-
Intelligent Document Processing: Automate loan application review, extract key facts from financial statements and generate reports with extraordinary accuracy.
-
Personalized Economic Forecasting: Use AI to analyze vast datasets and predict future cash flow needs, enabling businesses to make data-driven decisions.
-
Automated Code Reviews and Test Cases: Improve developer productivity and experience by automating code reviews and test cases.
-
Leveraging Image Generation and Content Creation: Create marketing materials and product knowledge bases with ease.
-
Synthetic Data Generation: Address data gap challenges and train ML models to improve speed to market with new AI solutions.
-
Customer Support and Experience: Handle multiple customer inquiries simultaneously, respond instantly to frequent questions, and troubleshoot issues.
Choosing the Right Model
Companies do not need to opt for large language models. Leaders have to look into the domain context, who will use your AI, and where you will use it. In Some Cases, they must consider Response time, Cost, Data Privacy, and specialized needs like On-Device Intelligence to finalize the business case.
SLMs (small language models), LAMs (Language Action Models), DLMs (Domain Language Models), and traditional ML and Deep Learning Models have many applications, particularly for organizations with specialized needs. On Device Intelligence, Apple, hugging Face, and Microsoft launched small Models for Specific use cases and needs.
Different implementation strategies exist for the use cases, ranging from customizing models to building from scratch or buying from an external Cloud or API provider.
Building Compound Systems - Monolithic Models to Compound AI
We're shifting from models to compound systems. Organizations must adopt data-centric AI and build compound AI systems with agentic workflow. RAG is an example of a compound system.
Reasons Why Enterprises Adopting Generative AI
Large enterprises are looking to adopt generative AI to enhance their services, creating a competitive edge and addressing risk management and regulatory compliance.
-
Improve Efficiency and Productivity
-
Enhance Creativity and Innovation
-
Improve Decision-Making and Analytics
-
Personalize Customer Experiences
Step to Successfully Pilot Generative AI and Deliver Business Value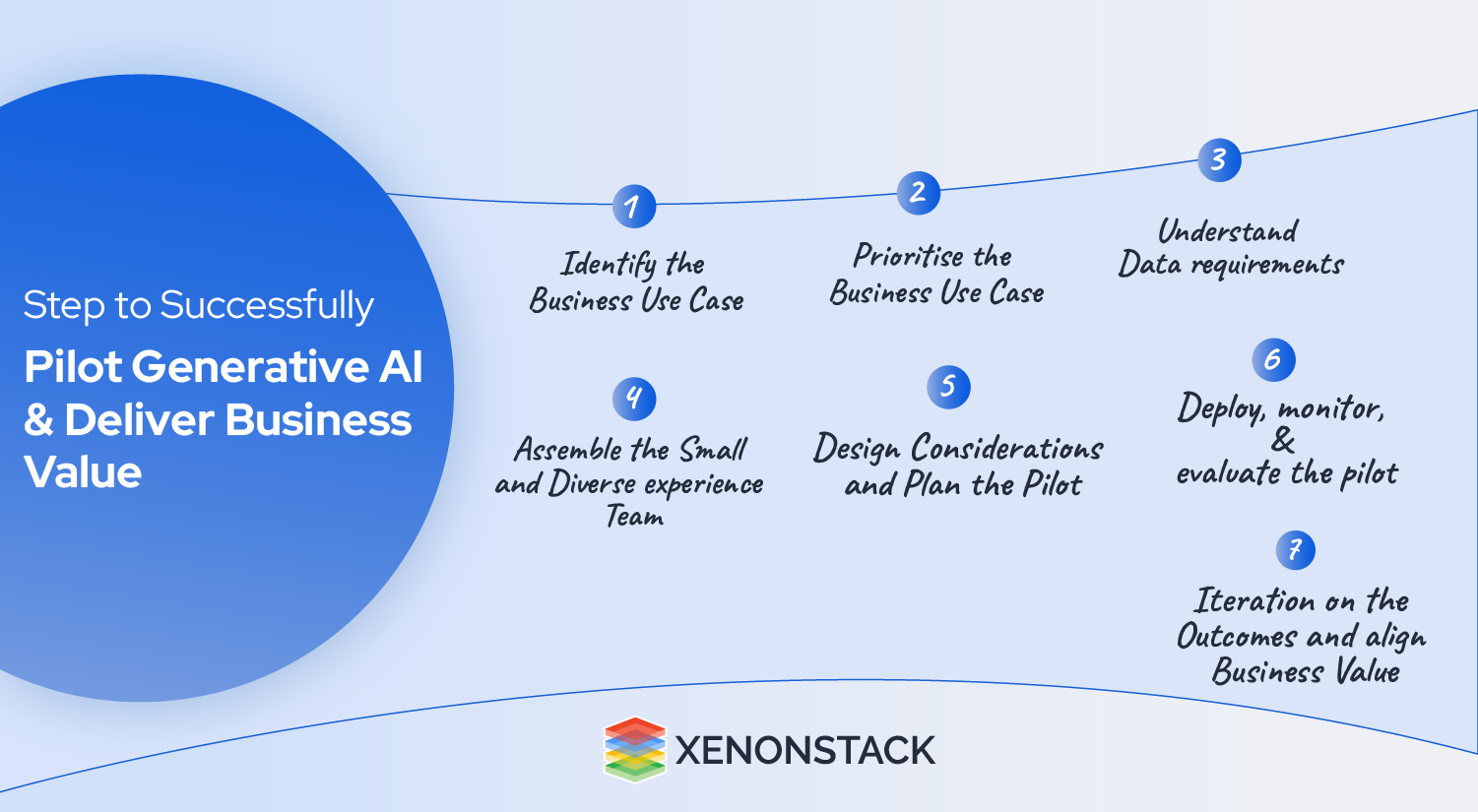
1. Identify the Business Use Case
-
Get input from the business on potential use cases
-
Align use cases to your business and IT strategy.
-
Select the best use cases from among the many available options.
2. Prioritise the Business Use Case
3. Understand Data requirements
4. Assemble the Small and diverse Experience Team
5. Design Considerations and Plan the Pilot
-
Determine Pilot Objectives and KPIs
-
Determine Risks and Mitigations for Pilot Use Cases
-
Decide on the Deployment Approach
6. Deploy, monitor, and evaluate the pilot
7. Iteration on the Outcomes and align Business Value
Data Readiness for Enterprises
Gartner reports that organizations utilizing active metadata, ML with Unified Data Platform to dynamically connect, optimize, and automate data management processes will reduce time to integrated data delivery by 30%. Augmented data management uses ML and AI techniques to optimize operations.
Scaling Generative AI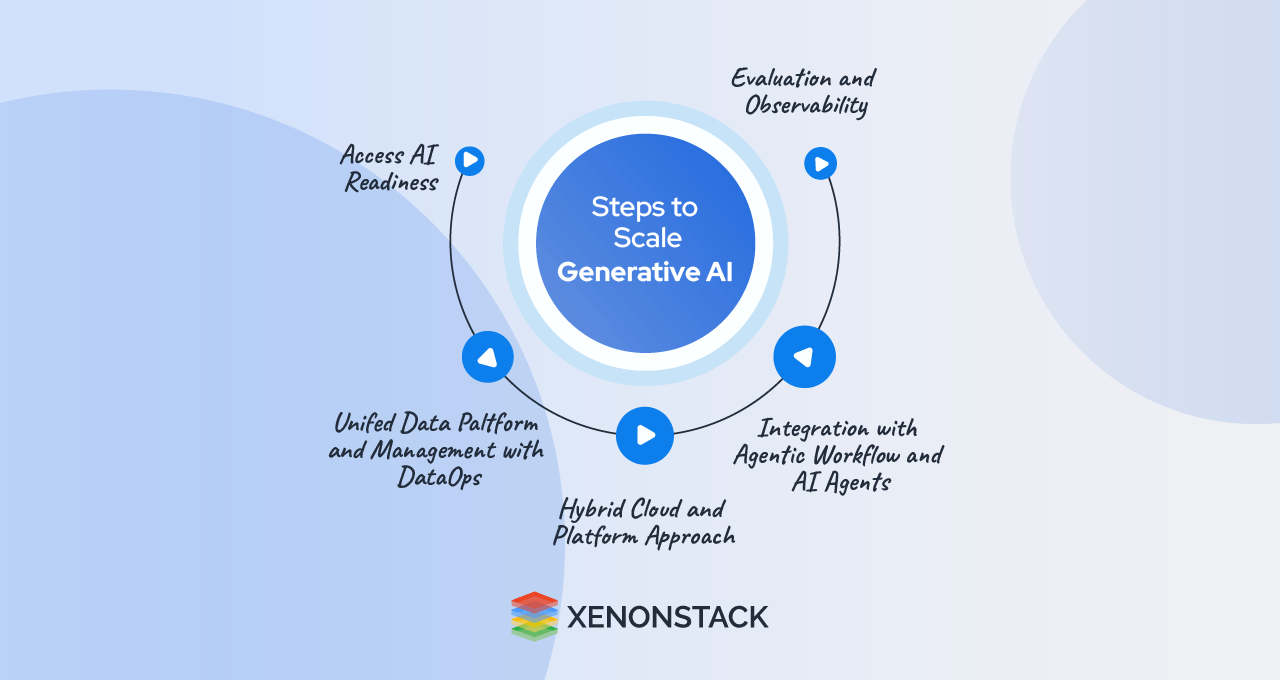
As generative AI models continue to evolve, scaling their capabilities to accommodate increasing complexity and dataset sizes is a crucial challenge
1. Ensure AI Readiness
Before scaling generative AI, ensure your organization is ready. Assess data maturity, workforce skills, and technical capabilities to identify areas for improvement.
2. Implement a Unified Data Platform
A unified data platform and DataOps are crucial for scaling generative AI. This ensures seamless data flow, reduces silos, and provides real-time insights.
3. Choose the Right Platform and Hybrid Cloud
Select a platform and hybrid cloud that can handle AI workloads, offering scalability, security, and reliability. SRE practices ensure infrastructure is designed to scale with your business needs.
4. Integrate with ERPs and Business Processes
Integrate generative AI with existing ERP systems and business processes using agentic workflows and AI agents. This automates tasks, provides predictions, and frees up human agents.
5. Monitor Observability and Evaluation
Monitor key performance indicators like model performance, data quality, and user adoption to ensure your implementation meets its goals. Identify areas for improvement and optimize for business value.
Challenges in Scaling Generative AI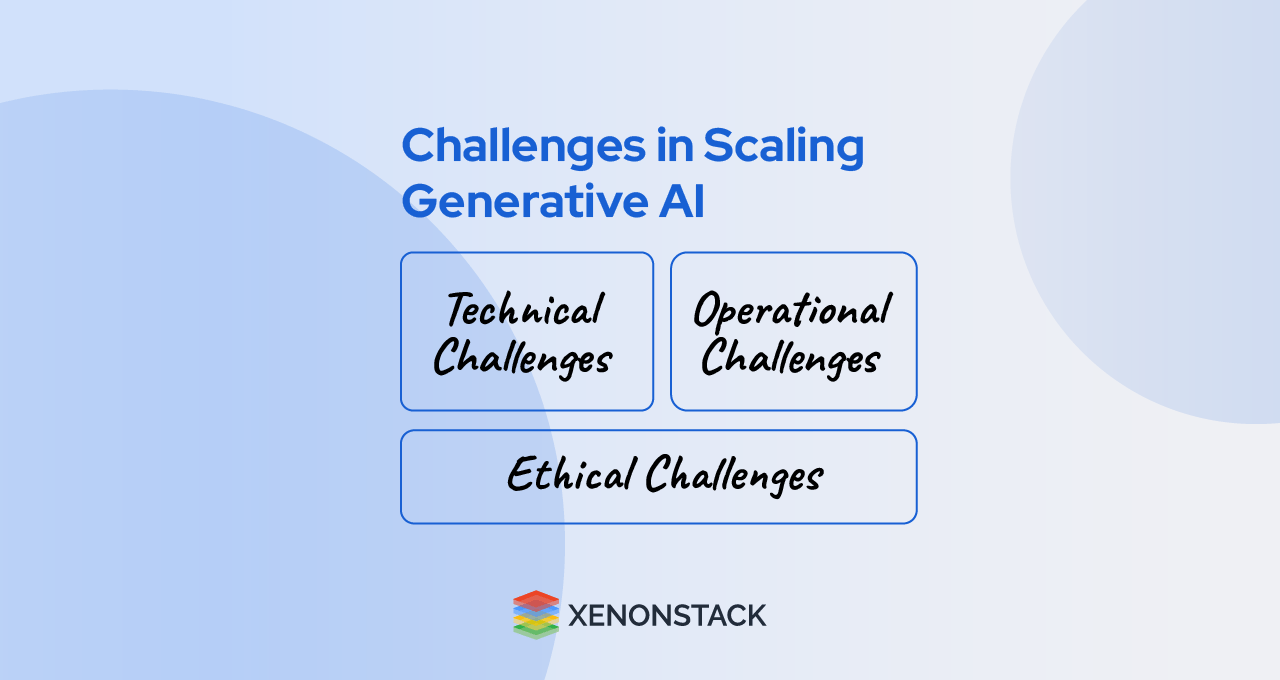
-
Infrastructure Integration: Integrate infrastructure with existing technology stacks carefully to avoid issues.
-
Siloed Data: Break down data silos to ensure seamless data flow.
-
Data Quality: Ensure high-quality data for accurate models.
-
Scalability: Design systems to handle increasing volumes of data, users, and processing power.
-
Business Process Integration: Define clear roles, responsibilities, and workflows for human-machine collaboration.
-
Team member Up-Skilling: Educate employees on AI capabilities and limitations.
-
Ethical Considerations: Mitigate bias, protect privacy, and maintain security standards to build trust.
Lessons for the Future of Autonomous Operations
-
Reimagine Business Processes and Manage Change
-
Prioritising Gen AI and Automation Solutions that address specific business needs and offer clear value.
-
Data Quality and Data Value Chain is Key to support AI initiatives and drive accurate, actionable insights.
-
Continuous Experimentation and Fostering a Cultivation Culture that embraces change and innovation
Conclusion
In a world where the boundaries of innovation are being rewritten, generative AI is poised to revolutionize the way we operate. But, to harness its full potential, you need a clear strategy. Don't just adopt the tech - master it.
In this article, we've outlined the essential steps to pilot generative AI in your organization and reap its transformative benefits. From automating tasks to enhancing customer experiences, the possibilities are endless. But, it's not just about having the technology - it's about building a data-centric strategy that drives real business value.
So, what are you waiting for? Watch the video to discover how to stay ahead of the curve and unlock the full potential of generative AI
Link - Pilot Generative AI - Transform Business Operations and Accelerate Innovation
-
Read more about LLMs and Knowledge Graph for Generative AI
-
Know more about Generative Adversarial Network Architecture