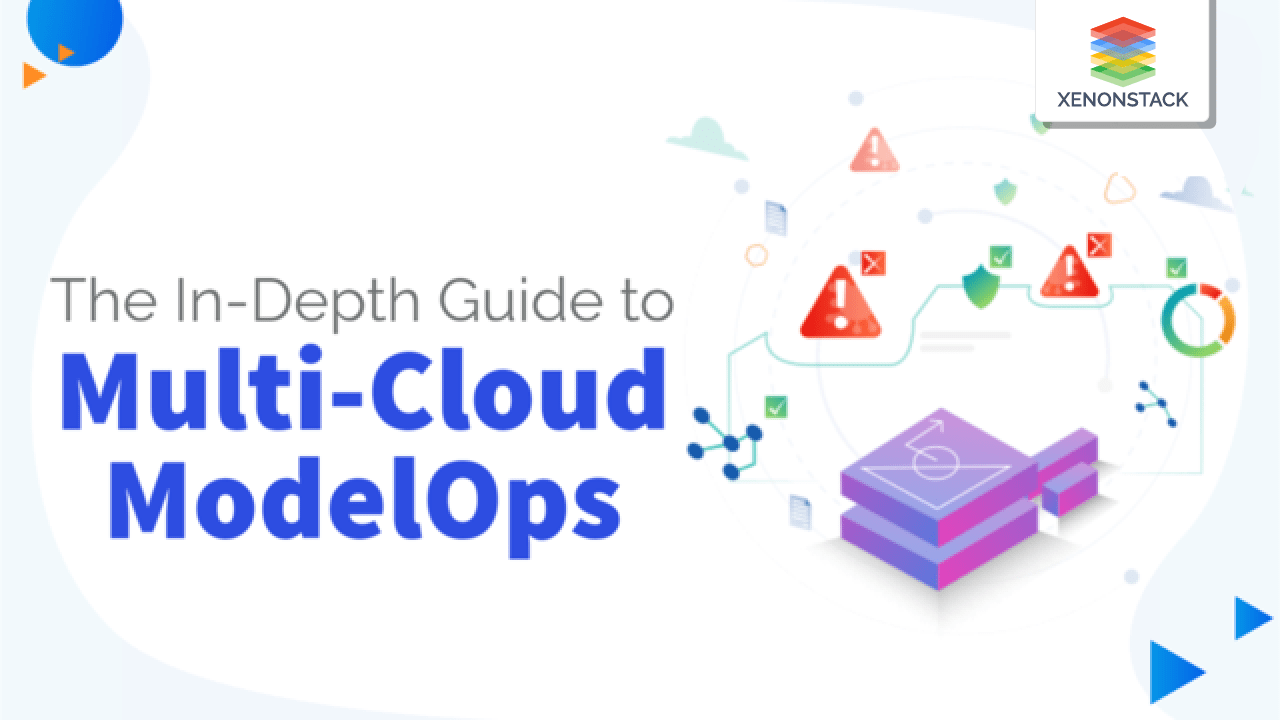
What are the Benefits of Multi-Cloud ModelOps?
-
Avoids Vendor Lock-In: By utilizing multiple cloud providers, organizations can enhance flexibility and portability for their AI systems, making it easier to switch providers if necessary.
-
Optimizes Performance: Different clouds excel in different areas; for example, AWS may be better for serverless applications, while Google Cloud Platform (GCP) might offer superior machine learning infrastructure. A multi-cloud approach allows organizations to leverage these strengths effectively.
-
Increases Resilience: Distributing workloads across multiple clouds minimizes risks associated with service outages or data loss, ensuring greater operational continuity.
-
Enhances AI Lifecycle Management: Automated management of the AI lifecycle can speed up time-to-value by improving team collaboration and streamlining processes for model deployment and monitoring.
-
Facilitates Compliance: Multi-cloud strategies can help organizations meet regulatory requirements by allowing them to store data in specific geographic locations as needed
-
Generate a Model Pipeline Leaderboard: Data is automatically prepared, the model chosen, feature engineered, and hyperparameter optimized.
-
Monitor Machine Learning Models: See potential models and learn how they can be mitigated and how findings can be explained.
-
Examine and Debias models: Generate and illustrate the explainability of debiased model points.
-
Deploy model Deployment Functions with Applications: Preprocessing of data before sending it to models and handling bugs, including calling several models
-
Mix and Match Cloud to Build and Deploy Models: Deploy and virtually drive models everywhere.
-
Build, Run, and Manage Models on a Unified Interface: Prepare knowledge, construct templates, and calculate results. Models with feedback loops are improved continuously.
Edge computing is transforming how data from millions of sensors worldwide are treated, stored, and distributed. Click to explore our, Drivers of Edge Computing and Edge AI
Final Thoughts
A fully integrated multi-cloud data and AI infrastructure can help you simplify AI lifecycles while incorporating hand-offs between technical and operational silos, speeding exploration and execution. A platform also makes AI model consumption simpler and encourages beginners to communicate with experts and learn from them to become main contributors quickly. This is why a data and AI network is the perfect setting for the app and AI cadence synchronization to construct Model Operations (ModelOps) using the Development Operations community (DevOps).
- Click to know What is ModelOps and its Operationalization?
- Read more about Overview of Edge AI Implementation