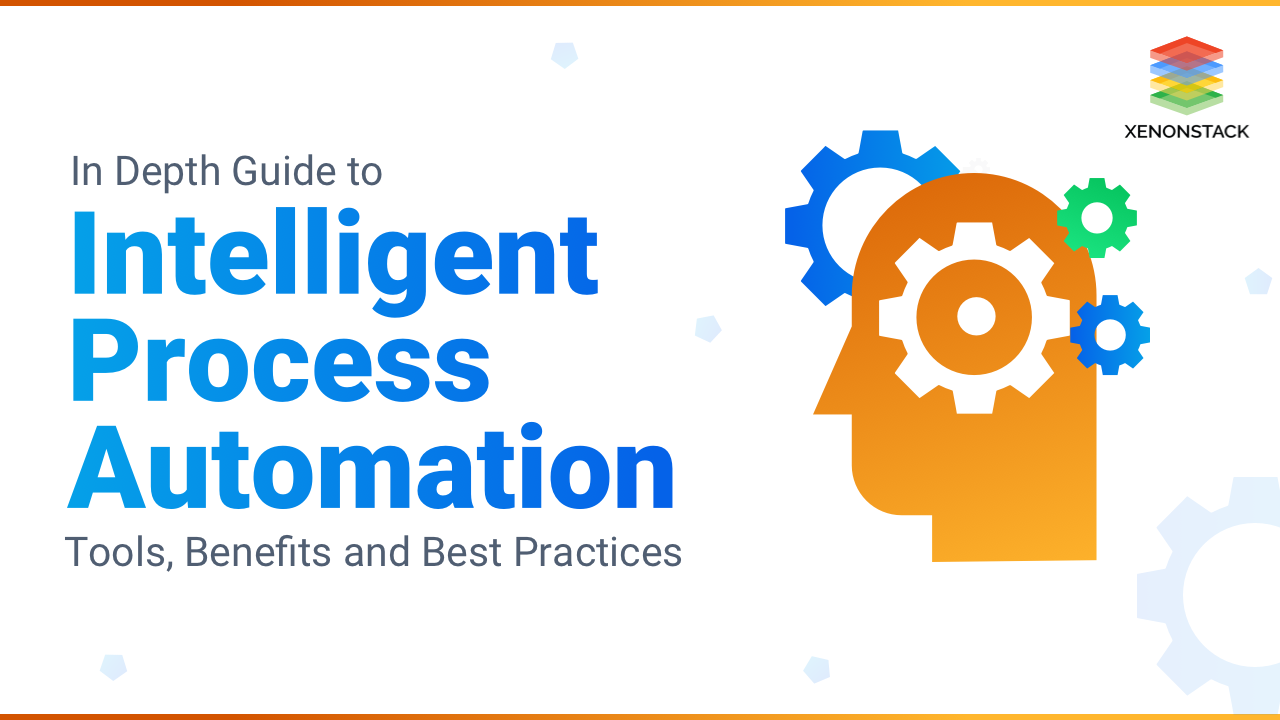
What is Intelligent Process Automation?
Artificial Intelligence is a vital factor in the field of Education, Market, or Finance. It is a step towards the introduction of intelligence in every field. The Integration of Intelligence with automation is known as "Intelligent Automation." The main points which can define the presence of Intelligence in automation are -
- There is an amount of some intensity of it is linked with the procedure of the components of the system.
- These components have the power to decipher the condition of the system's ecosystem and to identify the purpose of the other components.
The combination of Artificial Intelligence and Automation is Intelligent process automation. Click to explore about, Intelligent Robotic Process Automation
How Intelligent Process Automation works?
Intelligent Process Automation is divided into three prime modules, which have to follow their procedure and have to functionalize to achieve their different objectives.
- Chatbot - An interface that provides the impression of the "Doer."The main objective of this module should be "Automating the pursue the process."
- Decision System - A Decision System to decide what the "Doer" can do. The main target is that it can functionalize as a "Thinker." The main target is to "Automate the thinking process."
- Self-Learning Model - These modules provide the learning power to the whole automation process. The primary target is to "Automate the learning process." Give a read to this blog based on how Test Automation in Digital World matters the most.
Benefits of Intelligent Process Automation
Intelligent process automation Solutions (IPA) help to manage, automate, and integrate business processes and ensure that the right data gets to the right place at the right time, and facilitate end-to-end process automation.
Key Benefits | Description |
Reductions in cost | Takes less maintenance cost and also reduces the co-worker's loss as well as takes off the burden from them. |
Increment in Performance | Provide accuracy in computation-related problems, which results in an increase in performance. |
Advance Experimentations | New products and services can be introduced using endless experimentation. |
Expedition in the time taken by market | With the help of it, the time to market can be accelerated. |
Reductions in the systems and operations complexity | The operations complexity and system complexity can be reduced as it provides very high computation power. |
Increment in production | Automation with AI can also increase the power of the production for an enterprise. |
Increment inefficiency | As computation power increases due to the introduction of AI, time complexity reduces as well as efficiency increases. |
An increment in the enterprise's innovation power | The introduction of AI relatively increases the innovation power of the enterprise, as new challenges arrive which require innovations to tackle those challenges. |
Why is Intelligent Process Automation important?
- Processing Speed - The speed of the process gets increase with the introduction of Artificial Intelligence in it.
- Accuracy Improvement - Artificial Intelligence is a computation process deep down inside, which makes it less error-prone and increases accuracy.
- Immense Availability - There are no issues in making available a robot for 24/7 and apparently without any pay.
- Reduction in Cost - Every robotic or automation process requires a low maintenance cost compared to the human workforce.
- The Increment in Adaptability - Intelligent Automation is the non-living entity that can be turned off /on at will.
Intelligent process automation software is designed to assist processes in more than managing operations. Click to explore about, Intelligent Process Automation vs RPA
How to adopt Intelligent Process Automation?
Every product seems to be revolutionary on paper. Whether someone knows every step or every concept but the main game is providing the theory of a model a life or, in the technological jargon, "bringing any model on to the production level." The following diagram demonstrates of the components and their placement at the production level.
Steps to follow while developing an Intelligent Automation -
- Initialize by finalizing a theory and then try to give demonstrate the approach.
- Decide the appropriate exceptions.
- Try to determine robust solutions.
- Identify the aid needed for the whole project and import the missionary persons on the platform.
- Identify and bring the tools required for automation.
- Follow the rules of delivering the methodology quickly.
- As intelligence involves intelligence, choose the procedure intelligently.
- Make sure that Information Technology should be the internal part.
- Record and collect the targeted benefits concurrently.
- Define the roadmap for tenability.
RPA in Human Resource Management significantly reduces the time employees devote to these tasks, enabling them to focus on more quality tasks Click to explore about, RPA in Human Resources Management
What are the best practices of AI in Automation?
Rules which should be followed to achieve the best results while implementing Artificial Intelligence in the field of automation -
- Give preferences to the perfectly satisfying Intelligent Automation use cases.
- Calculate the correct and pragmatic exceptions of Automation.
- Develop a well-defined roadmap of the whole procedure while getting into the process.
- Elect the appropriate and well-suited Automation tools and Operators.
- Re-architect the entire method to boost to achieve the targeted benefits.
- Empower the active cooperation between the IT field and the business field.
What are the best Intelligent Process Automation Tools?
Type of Tool | Key Features | Example |
Bot programming tool | Recorders of Macro size, Push and Pull (Flexible) visual programming tool | UiPath |
Bot Managing tool | A tool which can handle the exception of business, Tool which can provide the user access levels differently. | UiPath Orchestrator |
Bots | Integrations | York fusion RPA Express |
Thinkers | Cognitive automation can deal with the structured and unstructured type of data | Have to implement according to use-case |
Learners | Which can use historical as well as current data, which can be used by the employees without any complexity? | York fusion |
Our solutions cater to diverse industries with a focus on serving ever-changing marketing needs. Click here for our RPA Services and Solutions
Intelligent Process Automation Solutions
Intelligent Process Automation Solutions provides a unique and differentiating approach that includes an end-to-end perspective from ideation to production, allowing you to scale up operations, putting your organisation at the centre of all activities, stimulating the erosion of organisational silos around your front, middle, and back-office processes, and resulting in the emergence of a new, borderless, highly-automated client-centric organisation.
Conclusion
The combination of Artificial Intelligence and Automation is Intelligent process automation. It brings out new abilities to automation. Moving beyond traditional business process management boundaries. IPA software is designed to assist the processes more than managing operations.
- Click to know about Robotic Process Automation in Cybersecurity
- Explore the Machine Learning Trends for Businesses