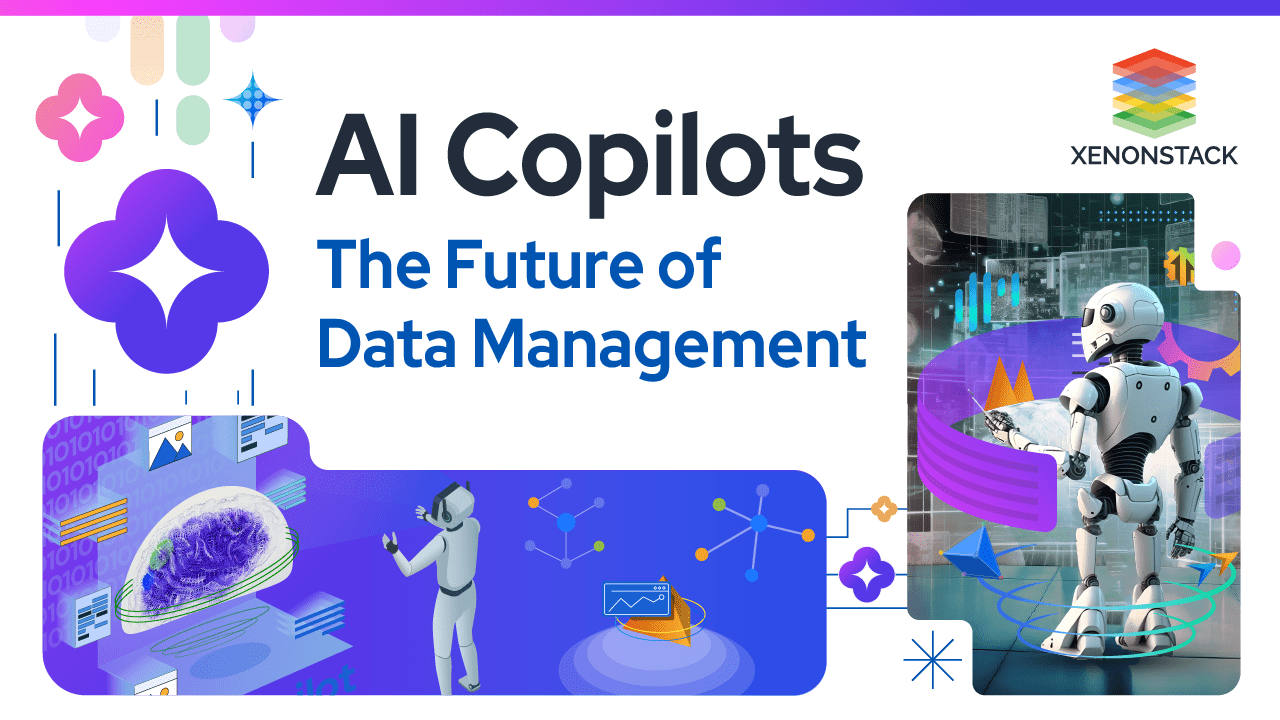
Introduction
Organizations are generating data at an exponentially higher speed than ever before, driven by advances in technology and the increasing digitization of business processes. For executives, especially those in roles related to Privacy, Security, or Data, this surge in data volume presents both opportunities and challenges. It is crucial for these leaders to have a fundamental understanding of the data within their organization. This knowledge allows them to effectively manage and safeguard the data, ensuring it is used in compliance with regulatory requirements and industry standards. A deep understanding of organizational data also aids in identifying potential security threats and implementing robust protection measures. Furthermore, it enables executives to leverage data for strategic decision-making, driving innovation and competitive advantage.
AI/ML often appears magical to business leaders due to its clear potential impact, yet they may struggle to fully grasp its complexities or the most effective ways to utilize these transformative innovations. These technologies serve as the foundation for a range of cutting-edge business solutions, including predictive analytics for optimizing next-best actions, real-time monitoring of customer satisfaction, streamlining operations for efficiency gains, and developing innovative products. Embracing AI/ML is crucial for businesses seeking to stay competitive in rapidly evolving markets, enabling them to harness data-driven insights for strategic decision-making and continuous improvement across various operational domains.
With the rise of generative AI set to accelerate these efforts, it's crucial to recognize its reliance on large language models (LLMs), which have voracious data requirements. LLMs necessitate extensive domain-specific data for training to ensure the accuracy needed for effective operation. It's imperative that this data accurately mirrors the current business landscape. Using poorly curated or insufficient data can severely hinder business initiatives, potentially leading to outcomes contrary to the intended goals. Therefore, ensuring the quality and relevance of data used in AI training is paramount to achieving positive impacts and avoiding detrimental effects on business outcomes.
Effective AI
Many people mistakenly believe that AI/ML can magically resolve all data quality and trust issues, effortlessly processing unstructured, nonstandard, and incomplete data to produce perfect results. However, this is a significant misconception. It is crucial to emphasize the importance of establishing a robust data management foundation focused on data quality for any successful AI transformation. AI/ML models can only perform well when built on accurate, well-organized, and comprehensive data. Without this solid foundation, the outputs of AI systems are likely to be flawed and unreliable. Thus, investing in high-quality data management practices is essential to harness the full potential of AI technologies.
To achieve effective AI models that utilize appropriate features, it is essential to access a diverse range of comprehensive, well-managed data sources from both internal and external sources. This data must adhere to high standards of quality and governance. By integrating and utilizing this varied data effectively, organizations can construct and train AI models effectively, powering large language models (LLMs) through robust data management practices. This holistic approach ensures that AI systems are well-equipped to deliver accurate and valuable insights, supporting informed decision-making and driving innovation across various business domains.
Role of AI in Data Management
AI is essential in data management because it automates repetitive tasks, significantly enhancing efficiency and reducing human error. It automates various Data Management Operations like enhances data cataloging by automating the organization and tagging of data assets, making them easily searchable and accessible. It can also ensures data governance by enforcing policies and standards, maintaining data quality and compliance. It also aids in metadata management, providing detailed context and lineage for data. It also helps in monitoring data usage and access, ensuring security and privacy regulations are followed.
Benefits of leveraging AI in Data Management
-
Productivity: AI Copilots can act as a recommender system in swiftly creating mappings for extracting, transforming, and delivering data. By learning from existing mappings and understanding the business context, it suggests suitable transformations to standardize and cleanse data for target systems and consumers.
-
Efficiency: AI helps in increasing efficiency of your teams by automating daily operations like Monitoring & Alerting. AI leverages historical time-series data from logs and monitoring files to anticipate and preemptively address potential issues before they escalate. This proactive approach enhances operational efficiency and reduces downtime by predicting and mitigating potential disruptions in data integration workflows.
-
Data Accessibility: In the realm of Big Data, data is typically gathered from diverse sources and stored across multiple tables, requiring Data Engineers to manually establish relationships between entities. AI offers the capability to automatically detect and reconstruct these relationships, simplifying the process of querying and analyzing data. This eliminates the need for users to recall outdated primary-key/foreign-key documentation or manually integrate datasets. Additionally, AI can identify datasets that exhibit similarities and provide recommendations based on usage trends, data quality assessments, and collaborative insights from the community. This enhances efficiency in data management and analysis, enabling more informed decision-making and streamlined operations within organizations.
-
Data Governance: AI and ML play a crucial role in automating complex data governance tasks such as data discovery, quality assessment, and facilitating collaborative governance efforts. It automates the creation of policy rules, such as data quality standards, and links business context to technical metadata. It assists users by recommending the most relevant and trustworthy data tailored to their business requirements, thereby enhancing decision-making and operational efficiency.
AI Copilot for Data Catalog
AI Copilot serves as a pivotal tool in modern organizations by overseeing and optimizing data catalog management through advanced AI and machine learning capabilities. Its automation features streamline the process of cataloging data assets, ensuring comprehensive coverage and accuracy across diverse datasets. Copilot utilizes machine learning algorithms to automatically categorize and tag datasets based on content and usage patterns, improving data discoverability and accessibility for users. Moreover, it facilitates the integration of business context with technical metadata, enhancing the relevance and usability of data within organizational workflows. By continuously learning from user interactions and feedback, Copilot refines its algorithms to provide more precise recommendations and insights.
Automated Metadata Management
AI Copilot can play a crucial role in automating metadata management by handling the generation, updates, and validation processes. This automation ensures that metadata, which includes information about data attributes, usage, and relationships, remains accurate and consistent across all data assets within an organization. By automating these tasks, AI Copilot reduces the likelihood of human error and ensures that metadata is always up-to-date and reflective of the current state of data. This not only enhances the efficiency of data management processes but also improves the reliability and usability of metadata for various analytical and operational tasks.
Smart Tagging
AI Copilot can allow tags to streamline the discovery and labeling of data fields significantly. Users assign simple tags to unclassified columns, and it learns from these tags, automatically applying them to similar columns. It aids in categorizing and tagging datasets automatically based on content and usage patterns, enhancing data discoverability.
Enhanced Search and Retrieval
Copilot can enhance search capabilities through the implementation of intelligent algorithms designed to prioritize datasets that are most relevant to user queries and historical usage patterns. By leveraging machine learning and advanced search techniques, Copilot ensures that users can quickly and efficiently retrieve the data they need. This intelligent search functionality not only saves time but also improves the overall user experience by delivering more accurate and tailored search results. Copilot continuously learns from user interactions and feedback to further refine its search algorithms, thereby enhancing its ability to provide meaningful and timely data retrieval capabilities for data-driven decision-making and operational efficiency within organizations.
Enhanced Discovery of Relationships
An essential task in data cataloging and modeling is documenting relationships among datasets. AI Copilot can employ machine-learning methods to automatically detect primary and unique keys and connections across structured datasets. This reduces months of documentation work to mere minutes. It continually enhances its ability to identify relationships by involving humans in the data curation process. For instance, users can approve or decline inferred relationships and refine capabilities through these interactions as their copilot.
Intelligent Data Similarity
Leveraging ML techniques like clustering, serves as a copilot to identify similar data across vast databases and file sets. This capability of intelligent data similarity is crucial for various tasks such as data identification, duplicate detection, and consolidating individual data fields into cohesive business entities. By propagating tags across datasets and recommending datasets as a trusted copilot, it enhances data management efficiency. Data similarity assesses the degree of similarity between data in two columns, a task impractical with brute-force methods across large enterprise datasets. Instead, it utilizes machine-learning to cluster similar columns and pinpoint likely matches, optimizing data comparison and integration processes.
Analytics Copilot
AI Copilot can play a crucial role in recommending data sources for data preparation tasks by utilizing its advanced capabilities in data discovery, analysis, and user interaction. Through sophisticated algorithms and machine learning techniques, Copilot can automatically identify relevant datasets across various sources based on their relevance to specific data preparation needs. It analyzes historical usage patterns, data quality metrics, and user preferences to recommend the most suitable data sources. Additionally, Copilot learns from user interactions and feedback to continuously improve its recommendations, ensuring that the suggested data sources align closely with the requirements of data engineers and analysts. This capability streamlines the data preparation process, enhances productivity, and facilitates more informed decision-making by providing access to high-quality and relevant data sources.
Usage Pattern Analysis
AI Copilot can utilize historical usage patterns and user preferences to analyze how data has been interacted with and consumed in the past. By examining these patterns, Copilot can recommend data sources that are most relevant to current needs, ensuring that the recommended datasets align closely with user expectations and requirements. This analysis enables Copilot to provide personalized recommendations, enhancing the efficiency of data discovery and selection processes for data engineers and analysts. Additionally, by continuously learning from user interactions and feedback, Copilot improves its recommendation accuracy over time, adapting to evolving data needs and preferences within the organization.
Similarity Detection
Copilot can leverage machine learning techniques to detect similarities between datasets or data sources by analyzing their content and structure. This capability allows Copilot to identify datasets that exhibit comparable attributes or patterns, thereby suggesting alternative or complementary sources of data. By leveraging advanced algorithms, Copilot can recommend additional datasets that may provide valuable insights or fill gaps in existing data collections. This approach enhances data exploration and analysis by facilitating the discovery of related datasets, ultimately supporting more comprehensive and informed decision-making processes. Copilot continuously refines its similarity detection capabilities through ongoing learning from data interactions and user feedback, ensuring that its recommendations remain relevant and beneficial to data engineers and analysts.
Collaborative Filtering
AI Copilot can utilize collaborative filtering algorithms to recommend data sources that are popular or frequently accessed by similar users or teams within the organization. This approach involves analyzing the historical usage patterns and preferences of users to identify similarities in data consumption behaviors. By understanding which datasets are commonly utilized by comparable user groups, Copilot can suggest relevant data sources that align with the specific needs and interests of individual teams or departments. This capability enhances data discovery and selection processes by providing tailored recommendations that reflect the collective preferences and behaviors of similar user profiles. Additionally, Copilot continuously improves its recommendations through ongoing feedback and learning from user interactions, ensuring that its suggestions remain accurate and beneficial for optimizing data utilization and decision-making within the organization.
User Feedback Integration
Copilot can be integrated with user feedback regarding recommended data sources to enhance and optimize its recommendations progressively. By gathering insights from users about their experiences and preferences with suggested datasets, Copilot refines its algorithms to better align with evolving data requirements within the organization. This iterative process allows Copilot to adapt its recommendations based on real-time feedback, ensuring that the suggested data sources remain relevant and valuable to users. By continuously learning from user interactions and refining its recommendation models, Copilot enhances its ability to provide personalized and effective data source suggestions. This approach not only improves the efficiency of data discovery but also supports better decision-making by delivering more accurate and tailored recommendations tailored to the specific needs of data engineers and analysts.
Contextual Understanding
Copilot can takes into account the specific context of data preparation tasks, including project requirements, data quality standards, and compliance regulations, when recommending suitable data sources. This capability ensures that the recommended datasets align closely with the unique needs and constraints of each project or initiative within the organization. By understanding the context surrounding data preparation activities, Copilot can provide more targeted and relevant recommendations that meet specific criteria such as data integrity, security, and regulatory compliance. This contextual awareness enhances the efficiency and effectiveness of data sourcing efforts by ensuring that the recommended sources not only fulfill technical requirements but also adhere to organizational policies and standards. By integrating contextual understanding into its recommendation process, Copilot supports data engineers and analysts in making informed decisions and achieving their objectives more effectively.
Data Governance and Data Quality Copilot
AI and ML play a crucial role in automating complex data governance tasks such as data discovery, quality assessment, and facilitating collaborative governance efforts. AI Copilot can automates the creation of policy rules, such as data quality standards, and links business context to technical metadata. It assists users by recommending the most relevant and trustworthy data tailored to their business requirements, thereby enhancing decision-making and operational efficiency.
Policy Enforcement
Copilot can automates the enforcement of data governance policies by continuously monitoring various aspects of data management, including data usage, access permissions, and compliance with regulatory standards. This involves implementing automated checks and controls to ensure that data handling practices align with established policies and regulations. Copilot proactively identifies and addresses potential policy violations or discrepancies by analyzing data activities in real-time. By leveraging machine learning and advanced analytics, Copilot can detect anomalies, unauthorized access attempts, or deviations from predefined governance rules. This automated enforcement not only helps maintain data integrity and security but also streamlines compliance efforts by reducing manual oversight and intervention. Copilot's proactive approach to policy enforcement ensures that data governance practices remain robust and consistent across the organization, promoting trust, transparency, and accountability in data management processes.
Data Quality Management
Copilot can play a pivotal role in overseeing and enhancing data quality through automated processes that identify anomalies, inconsistencies, and errors within datasets. By leveraging advanced algorithms and machine learning techniques, Copilot can detect deviations from expected data patterns or quality thresholds in real-time. This proactive monitoring ensures that data remains accurate, reliable, and fit for its intended use. Copilot not only identifies potential issues but also supports data engineers and analysts in addressing them promptly. Through continuous monitoring and analysis, Copilot contributes to ongoing improvements in data quality by facilitating timely corrections and adjustments. This capability is crucial for maintaining the integrity and trustworthiness of data assets across the organization, supporting informed decision-making and operational efficiency.
Access Control and Security
Copilot can play a critical role in enforcing access controls and enhancing data security measures within an organization. By leveraging its capabilities, Copilot recommends appropriate permissions based on the sensitivity of data and the roles of users accessing it. This guarantees that sensitive information is accessible only to authorized personnel, safeguarding data confidentiality and integrity. Copilot automates the allocation of access rights, ensuring data security and compliance with regulatory standards. It also continuously evaluates access patterns and user behaviors to detect and mitigate potential security risks or unauthorized access attempts. By integrating with existing security frameworks and policies, Copilot enhances the overall governance of data access and security, promoting a secure data environment. This proactive approach helps organizations mitigate security threats and maintain trust in their data management practices, supporting overall business continuity and compliance efforts.
Compliance Monitoring
Copilot can enhance compliance monitoring by actively tracking various aspects of data management, including data usage patterns, audit trails, and adherence to regulatory requirements. This capability enables organizations to monitor and enforce compliance with industry regulations and internal policies effectively. By analyzing data usage and access patterns in real-time, Copilot identifies potential compliance issues or deviations from established standards. It facilitates proactive measures to mitigate risks by alerting stakeholders to potential violations and providing insights into corrective actions. Copilot supports continuous monitoring and auditing of data activities, ensuring transparency and accountability in data governance practices. By automating compliance monitoring tasks, Copilot helps organizations maintain regulatory compliance, mitigate risks associated with data breaches, and uphold data protection standards. This proactive approach strengthens overall governance and risk management efforts, fostering trust and confidence in data management practices across the organization.
Automated Data Discovery
Automated data discovery uses AI and machine learning to identify and catalog data assets from various sources and environments within an organization. By automating this process, Copilot can ensure comprehensive coverage and visibility of all data assets, regardless of their location or format. This capability facilitates effective data governance by providing data stewards and administrators with a centralized view of the organization's data landscape. Copilot can automatically scan databases, file systems, and other data repositories to discover new datasets, ensuring that no critical data is overlooked. This automated approach not only saves time and effort but also improve the accuracy and efficiency of data governance initiatives. It allows organizations to maintain a current inventory of data assets, track data lineage, and ensure compliance with data management policies and regulatory requirements.
Collaborative Governance
Copilot can facilitate collaborative governance by offering intuitive interfaces and recommendations that encourage active participation from data stewards, business users, and IT teams in the formulation and implementation of governance policies. It provides a platform where stakeholders can interact, share insights, and contribute to the development of governance frameworks tailored to organizational needs. Copilot enhances collaboration by suggesting best practices and policy guidelines based on industry standards and regulatory requirements. This fosters alignment between business objectives and data management practices, ensuring that governance policies are comprehensive and effective. By promoting transparency and accountability, Copilot enables stakeholders to make informed decisions about access controls, data usage, and compliance measures. It supports ongoing communication and feedback loops, allowing governance policies to evolve in response to changing business dynamics and regulatory landscapes.
Popular Copilots in the market
Informatica Claire
Informatica enhances data management with its Intelligent Data Management Cloud, a comprehensive platform designed for efficiency across diverse environments. It offers seamless connectivity and robust capabilities like metadata management and operations management. The platform supports various data sources from on-premises to multi-cloud setups.
Modular in design, it allows organizations to start with specific capabilities and expand gradually. Informatica excels in metadata management, collecting technical, business, operational, and usage metadata crucial for AI/ML. This metadata enriches machine learning algorithms, enhancing their accuracy and adaptability.
CLAIRE, powered by this rich metadata, drives Informatica's AI/ML initiatives. It offers intelligent recommendations, automates project development, and adapts to dynamic enterprise needs. CLAIRE acts as a copilot, integrating metadata intelligence across all data management functions, ensuring optimized operational efficiency and decision-making.
Microsoft Fabric Copilot
Microsoft Fabric Copilot for Data Science and Data Engineering is an AI assistant tailored to analyze and visualize data seamlessly. It supports Lakehouse tables, Power BI Datasets, and various dataframes like pandas, spark, and fabric. Users integrate their data as dataframes for optimal interaction, posing queries through a chat panel where Copilot responds with relevant insights or code snippets directly applicable in their notebooks. Copilot comprehends data schemas and metadata, utilizing dataframe awareness to enhance interactions. It facilitates tasks such as generating visualizations, transforming data with code snippets, and referencing files effortlessly. Copilot simplifies data analysis by minimizing the need for intricate coding processes.
BigID AI Copilot
BigID AI Copilot enables rapid comprehension of structured and unstructured data sources, applying consistent data labels critical for business, privacy, and security stakeholders. By standardizing data label logic at the source, it ensures clarity and coherence throughout all business processes. This approach eliminates incomplete tagging issues by integrating AI to fill any gaps, ensuring comprehensive data understanding and context across all downstream applications and users from the outset.
Cribl
Cribl Copilot stands out as an AI-driven solution designed to enhance the efficiency of managing large-scale IT and security data operations. It leverages its AI capabilities to swiftly deliver operational value, enabling users to expedite data deployments and achieve rapid access to desired information within minutes. By harnessing Cribl's extensive engineering knowledge, Copilot empowers organizations to optimize workflows and address complex data challenges effectively, all without the usual learning curve delays.
Conclusion
The integration of AI and machine learning within this platform marks a transformative leap forward for organizations aiming to capitalize on their data assets. By acting as a strategic enabler, it not only facilitates data-driven innovation but also fosters continuous productivity improvements across all operational domains. This platform empowers decision-makers with actionable insights, enhances operational efficiency through automation, and enables proactive strategies based on predictive analytics. As organizations navigate an increasingly data-centric landscape, leveraging such advanced capabilities becomes essential to staying competitive, driving growth, and delivering superior value to stakeholders. Embracing this integrated platform promises not just enhanced performance but also resilience in adapting to evolving business demands and technological advancements.
-
Why Rust is necessary for Big Data Processing Applications?
-
What is the importance of distributed Data processing with Apache Flink?