What are Autonomous Agents?
Autonomous agents are computer systems that are capable of independent decision-making and taking actions in their environment without direct human intervention. They are designed to perceive and understand the surroundings and situations, and they will perform actions to complete their tasks. They operate without human control and rely on their own internal logic and reasoning capabilities to make decisions. They can learn and adapt to changes in the environment and adjust their work strategies.
Some examples of Autonomous Agents -
-
Self-driving cars: By adding sensors and advanced algorithms to navigate roads, these cars can notice their surroundings and then decide about direction, speed, and obstacles.
-
Personal assistants: Virtual assistants like Siri and Alexa can understand user commands and information to perform tasks like setting alarms or booking appointments.
-
Trading robots: These Robots use algorithms to analyse market data, identify trading opportunities then execute the trades automatically.
-
Game-playing agents: AI-powered characters in video games can learn and adapt their strategies based on their opponent's behavior.
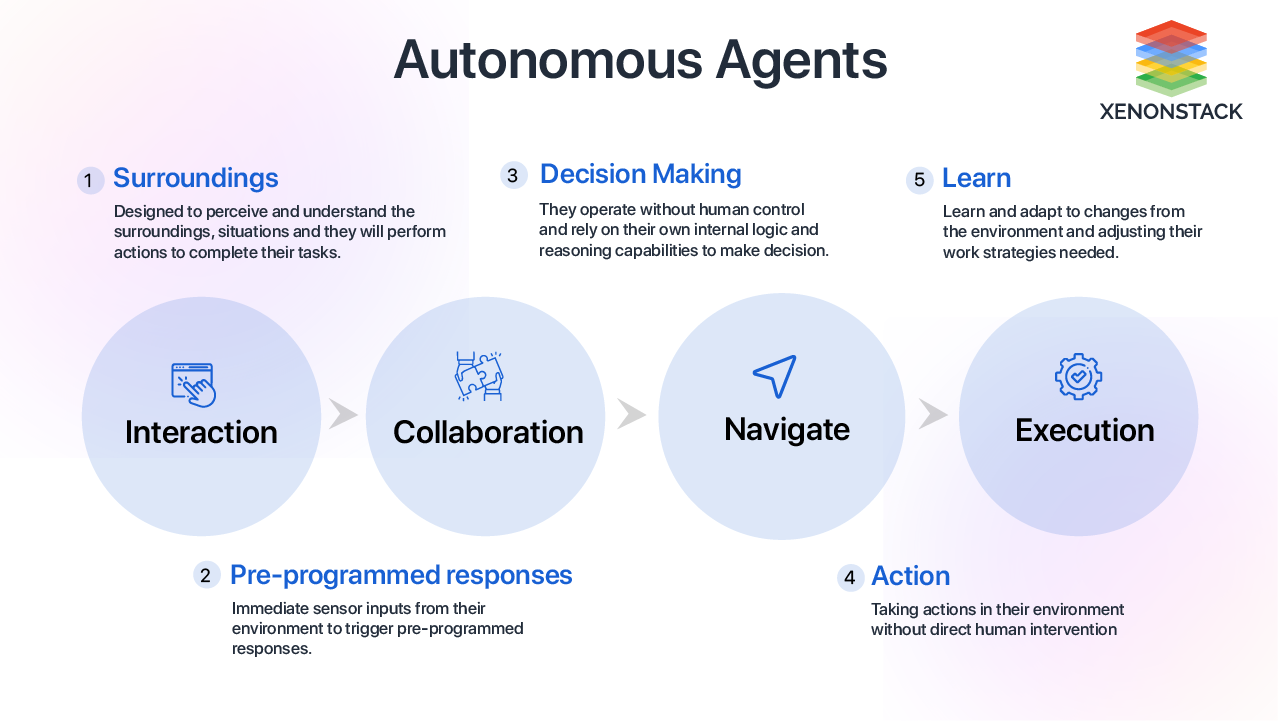
Why are Autonomous Agents Important?
Autonomous agents are playing an important role in our world by bringing significant benefits in terms of efficiency, safety, exploration, and innovation. Autonomous agents hold significant importance for many reasons:
Increased efficiency and productivity: They can handle repetitive tasks and reduce human efforts, while humans can focus on more complex tasks and operate continuously without breaks; hence, this will increase productivity.
Enhance Safety and Reliability: They can reduce the chances of human error and perform risky tasks where humans are at risk of accidents and injuries. They can perform tasks without taking any breaks and ensure reliable outcomes. Also, they can work for monitoring and efficient management.
New possibilities and applications: Autonomous agents can assist humans in their complex tasks, provide real-time data insights, or take autonomous actions based on their understanding of situations.
Innovation and growth: The advancements of autonomous agents contribute to progress in areas like healthcare, manufacturing, transportation, etc. As technology evolves, collaboration between humans and autonomous agents can unlock innovation.
Use cases of Autonomous Agents across different domains
Autonomous agents have great ability to perceive, reason and interaction with technology, here are some key use cases across different domains:
1. Robotics: Assembly line tasks, material handling and quality control in factories this comes under the Industrial automation domain, we can use them in logistics and warehousing in which the automated guided vehicles (AVGs) for efficient movement of goods in the warehouses and distribution centres these robots equipped with sensors and cameras for navigating and locating environment.
2. Healthcare: The robot surgeons can perform complex procedures with increased precision and minimal invasiveness theses AI-powered agents analyze large dataset and simulations to accelerate research and development processes.
3. Transportation: Autonomous vehicles navigate roads, perceive their surroundings and make decisions for safe and efficient transportation, the autonomous drones deliver packages and goods and enables cost-effective delivery options. AI-powered systems analyse traffic patterns and optimize traffic flow to reduce congestion and improve congestion.
4. Business and finance: We can use customer service chatbots which provide 24/7 customer support, answer inquiries and resolve basic issues. We can analyse financial transaction in real-time to identify suspicious activity and prevent fraud.
5. Education: Personalize learning assistants that adapt to individual student needs and provide targeted instruction.
6. Cybersecurity: Autonomous agents can detect and respond to cyber-attacks in real-time protecting systems and data from security breaches.
7. Agriculture: Autonomous systems monitor crops, manage irrigation and optimize the farming practices for improving working efficiency.
Core Concepts and Capabilities
Perception: Sensing and Interpreting the Environment
Perception is the foundation upon which autonomous agents operate. It's the process by which they gather information about their surroundings and transform it into a meaningful understanding of the world. This understanding is crucial for them to make informed decisions and take appropriate actions.
-
Sensing: Agents use various sensors to gather raw data from the environment. These sensors can include Cameras that can capture visual information like objects, shapes, and colors. LiDAR can create 3D maps of the surroundings using lasers. Radar that can detect objects and measure their distance and speed. Microphones that can capture sounds and identify spoken language or environmental noises.
-
Processing: The raw data from the sensors is processed to extract meaningful features and patterns. This involves filtering and removing irrelevant information or noise from the data. Feature extractions Identify key characteristics of objects, such as size, shape, and texture. Data fusion combines information from multiple sensors to create a more complete picture of the environment.
-
Interpretation: The processed data is then interpreted to give the agent a meaningful understanding of its surroundings. This involves object recognition, which can identify the objects present in the environment; scene understanding, which comprehends the relationships between objects and their spatial layout; and event detection, which can recognize significant events happening in the environment.
Reasoning: Making Decisions and Taking Actions
Reasoning is the core decision-making engine of an autonomous agent. It allows the agent to take the information gathered through perception (understanding the environment) and translate it into concrete actions to achieve its goals.
Goal Setting and Planning: The agent starts with a set of goals it wants to achieve. These goals can be pre-programmed or dynamically determined based on the situation. Based on the tasks, the agent creates a plan that outlines the steps it needs to take to reach them. This plan may involve some practices like sequencing actions to determine the order in which actions need to be performed, considering alternatives, exploring different possible courses of action, and choosing the most suitable one.
Decision Making: The agent continuously evaluates the state of the environment and its progress towards its goals. Based on this information, the agent makes decisions about what action to take next. This may involve reasoning about cause and effect and predicting the consequences of different actions. Learning from experience, the agent adapts its decision-making based on past successes and failures. The agent considers trade-offs and balances different factors like efficiency, safety, and resource constraints.
Learning and Adaptation
Autonomous agents aren't static entities. They possess the ability to learn and adapt to their surroundings, making them more effective and versatile in achieving their goals.
Learning: Autonomous agents learn by acquiring new information and experiences from their interactions with the environment. This information can come from various sources, including sensor data, rewards or penalties received for their actions and observations of other agents.
Types of Learning:
-
Supervised Learning: The agent is provided with labeled data (e.g., correct actions and their outcomes) and learns to map inputs to desired outputs.
-
Unsupervised Learning: The agent identifies patterns and relationships in unlabelled data, uncovering hidden structures in the environment.
-
Reinforcement Learning: The agent learns through trial and error, receiving rewards for successful actions and penalties for failures.
Adaptation: The agent uses its acquired knowledge and understanding to adjust its behaviour and decision-making strategies. This allows it to respond more effectively to changes in the environment or its goals.
Examples of Adaptation -
-
Refining action selection: The agent learns which actions lead to better outcomes and prioritizes them in future situations.
-
Improving perception: The agent's ability to interpret sensory data evolves, leading to a more accurate understanding of the environment.
-
Dynamic goal adjustment: The agent can adapt its goals based on new information or unexpected circumstances.
Types of Autonomous Agents
Reactive agents
Reactive agents are a fundamental type of autonomous agent that exhibit a simple yet effective approach to interacting with their surroundings. They function based on the following core principles:
-
Stimulus-response: Reactive agents primarily rely on immediate sensory inputs from their environment to trigger pre-programmed responses. They don't possess internal models of the world or the ability to reason about past experiences. Their actions are directly tied to the current stimuli they perceive.
-
Simple decision-making: Reactive agents employ a set of predefined rules or lookup tables to map sensory inputs to corresponding actions. They lack the ability to plan or strategize for future scenarios. Their decision-making process is fast and efficient but may not be optimal in all situations.
-
Limited adaptability: Reactive agents are generally not designed to learn or adapt their behavior based on experience. They operate within the confines of their pre-programmed responses and may struggle in dynamic environments where conditions change frequently.
Deliberative Agents
Deliberative agents represent a more sophisticated type of autonomous agent compared to reactive agents. They possess the ability to reason, plan, and make informed decisions before acting. Here's a breakdown of their key characteristics:
-
Internal world model: Deliberative agents maintain an internal representation of the world based on their perception of the environment. This model includes information about objects and their relationships with the current state of the world. It allows agents to simulate potential outcomes of different actions before committing to a specific course of action.
-
Planning and Reasoning: Deliberative agents employ planning algorithms to generate sequences of actions that will lead them toward achieving their goals. They use reasoning techniques to evaluate the feasibility and potential consequences of these plans, considering factors like efficiency, safety, and resource constraints. This process allows them to make informed decisions that are more likely to achieve their desired outcomes.
-
Learning and Adaptation: While not as prominent as in some other agent types, deliberative agents can exhibit some degree of learning through experience. They may update their internal world models based on new information and adapt their plans accordingly. This allows them to handle unforeseen situations and improve their performance over time.
Learning Agent
Learning agents represent a significant advancement in the realm of autonomous agents. They possess the ability to not only perceive and react to their environment but also continuously learn and adapt their behavior based on experience. This allows them to become more effective and versatile in achieving their goals over time.
-
Learning Mechanisms: Learning agents employ various algorithms and techniques to extract knowledge from their interactions with the environment. This knowledge can come from positive and negative reinforcement, receiving rewards for successful actions and penalties for failures. Observation and exploration involve actively seeking out new information and experimenting with different strategies. Data analysis involves Identifying patterns and relationships in the data they collect.
-
Adaptive Behaviour: Based on their acquired knowledge, learning agents can adjust their Decision-making by refining their choices based on what has worked well in the past and action selection prioritizing actions that are likely to lead to success. Internal models that update their understanding of the environment to reflect new information.
Social Agents
Social agents are a type of autonomous agent that specifically focuses on interaction and collaboration with other agents, whether human or artificial. They possess capabilities beyond just perception and action, incorporating social skills and understanding to navigate social environments effectively. Key characteristics of social agents:
-
Social Intelligence: They can understand and respond to social cues, including communication styles, emotions, and social norms.
-
Communication Skills: They can exchange information and collaborate with other agents using appropriate communication methods.
-
Theory of Mind: Social agents may exhibit some level of understanding of the mental states (beliefs, desires, intentions) of others.
-
Collaboration and Negotiation: They can work together with other agents to achieve common goals, potentially involving negotiation and compromise.