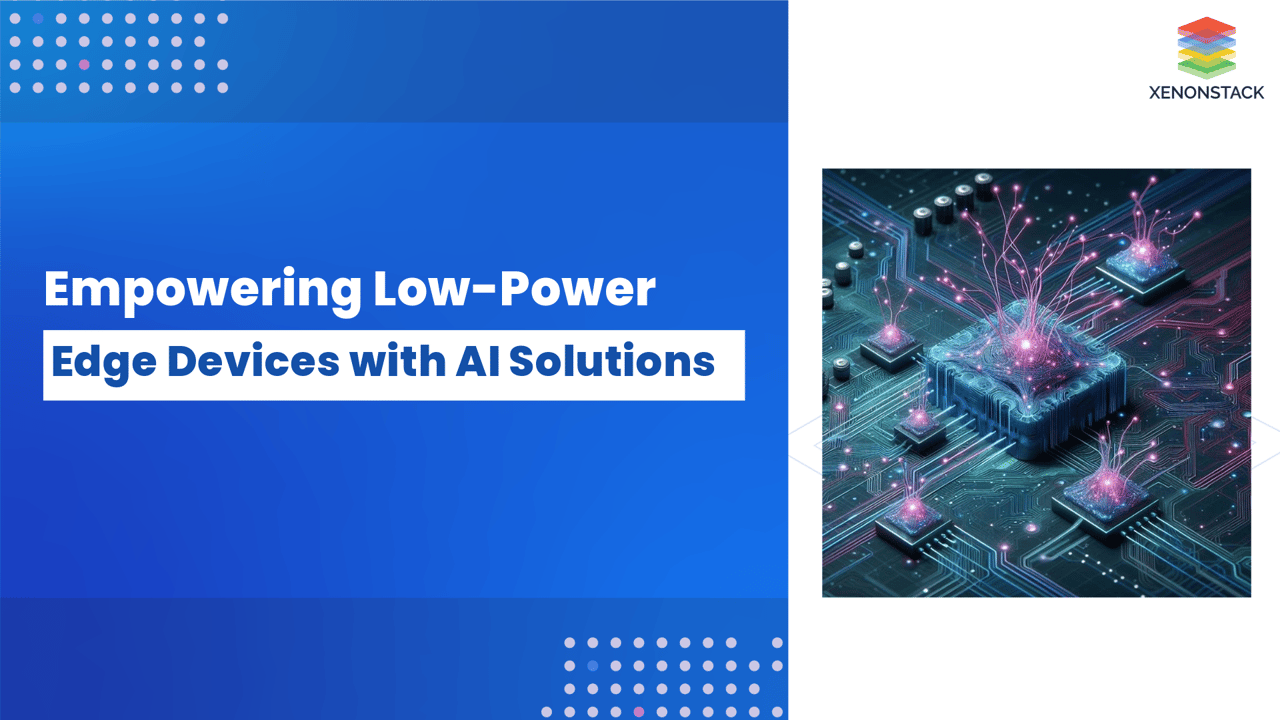
With the constantly changing face of the digital frontier, one can now incorporate AI into everyday items, changing what it means to engage a product. The huge revolution in computing is driven by edge devices like IoT sensors, wearables, smart appliances and even more. However, previous AI architectures that do not support the concept of neurosynaptic cores may consume significant amounts of computational power coupled with energy and, hence, cannot be implemented in low-power devices. In essence, as new possibilities for using AI are discovered, the ability to implement low-power AI solutions for edge devices has become an important invention that allows intelligent applications to thrive without using battery power. This blog will look at what low-power AI solutions are, why they are important, the developments that are currently being made, the effects of those developments, issues that have arisen, how these have been resolved, where the trends are evolving too and an outlook for low-power solutions.
Understanding Low-Power AI Solutions
What are Low-Power AI Solutions?
Low-power AI defines approaches and structures for AI modelling run on edge devices with low power consumption and resource demands. These solutions use light algorithms, model optimization, and optimized hardware for efficient Inference and learning in restricted power environments.
Major Features of Low-Power AI Systems
-
Energy Efficiency: Resource-efficient AI design reduces power consumption to the lowest possible level, allowing devices powered by low-energy sources like batteries or power-harvesting solutions to run longer.
-
Performance Optimization: These solutions define suitable performance levels, which depict the compromise of accuracy about computational complexity. Mainstream and efficient algorithms and models enforce low-power conductivity AI solutions to users without straining edge device resources.
-
Scalability: With low power, AI solutions can be implemented from smaller IoT sensor nodes to slightly more capable edge gateway nodes, making them flexible for many environments and applications.
-
Real-Time Processing: Real-time data processing is also needed in several edge use cases, including self-driving cars and industrial control systems. Real-time Power AI systems make prompt decisions to create superior value for end-users and better resource utilization.
Early Developments in Low-Power AI
The requirement for low-power AI finally arose when the devices spread exponentially within the IoT market. The initial trials attempted to build simple algorithms and avoid the resource-intensive parameters found in conventional AI systems. This area of study began with exploring how precisely neural networks should be designed to maximize performance alongside the constraints of the small device, laying the groundwork for further integration of AI at devices.
Example Early Implementations
-
Embedded Systems: In executing artificial intelligence algorithms, the first use of low-power AI was observed in embedded systems through computational components such as DSPs and FPGAs.
-
Decision Trees and Linear Models: Decision trees and linear regression were the earlier low-power applications of ML models. These models were simple to solve and provided useful information when fewer computational resources were available.
-
Feature Selection Techniques: Earlier works also addressed FFA for feature selection methods that aim at dimensionality reduction to limit the input to AI models and allow them to be processed under a limited number of resources.
Advancements Over Time
The Timeline of Low-Power AI Techniques
As the demand for AI solutions in low-power environments grew, numerous advancements emerged to enhance the capabilities of these solutions:
Model Compression
Hence, methods like pruning the model, reducing the size of the data types used in the model, and transferring knowledge learned in a complex model into a much simpler one reduce computational complexity and ensure model accuracy, and they are suitable for edge devices.
-
Pruning: Pruning edges in neural networks to get fewer parameters to train and compute time to handle them better.
-
Quantization: By cast to lower-precision weight formats (e.g., int8), memory for inference is reduced, and inference speed is increased.
-
Knowledge Distillation: Teaching a model with fewer parameters (the student) to emulate the behaviour of a model with substantially more parameters (the teacher) leads to a reduced-size model with minimal decline in efficiency.
Efficient Neural Architectures
New neural network architectures for mobile and edge applications include MobileNet and Efficient Net, among others. These architectures are optimized for computational, presupposing the comparative accuracy necessary to work in low-power systems.
Figure 1: Standard Convolution and Depthwise Separable Convolutions
Edge Computing Frameworks
Ever since, TensorFlow Lite, PyTorch Mobile, and ONNX Runtime frameworks have been created to assist in deploying AI models to edges. These frameworks are for tuning models and always have some micro-concern about matching to low-power devices.
Specialized Hardware
Recent developments in the AI hardware front include accelerators and edge SoCs (Google Coral, NVIDIA Jetson, among others). These are hardware solutions designed to run machine learning workloads while being energy efficient.
Federated Learning and On-Device Learning
Using federated learning and on-device training approaches, different edge devices learn from the data without sharing it with the cloud. This cuts the communication overhead and promotes privacy while, on the other hand, constant model refinement occurs.
Impacts of Low-Power AI Solutions
Transforming Industries
Low-power AI solutions are revolutionizing various industries by enabling smarter applications and improving operational efficiencies:
-
Healthcare: Advances in wearables embedded with low-energy AI solutions enable real-time monitoring of patient's health conditions, with alerts for abnormality and timely corrective action, all without incapacitating the battery.
Figure 2: Applications of AI in Healthcare
-
Smart Homes: Intelligent AI works hand in hand with low-power devices to perform activities such as voice recognition, electricity conservation, and automatic responses depending on user activities. All this and more are possible while using energy resources.
-
Agriculture: In precision agriculture, AI-driven IoT sensors collect data from the soil and the environment with low power consumption. This approach reduces resource usage while increasing crop production, resulting in better yields and reduced environmental effects.
-
Manufacturing: Low-powered AI solutions provide an avenue for predictive maintenance through real-time monitoring of machine data. This capability helps manufacturers detect and prevent problems from arising, thereby cutting on periods and costs.
Low-power AI is necessary for automotive applications, including ADAS and self-driving cars. Algorithms work on sensors, and real-time decision-making with the analyzed data is possible on the targeted device, increasing safety.
Overcoming Challenges in Low-Power AI
While low-power AI solutions offer numerous benefits, several challenges must be addressed to maximize their potential:
Short Resources
A key assumption must be made that the agents have limited computational capability. AI models used in edge devices also present challenges in tasking computation capabilities, which makes it very difficult to deploy powerful AI models at the edge.
Solution: Model Optimization Techniques
Optimizing the models using Model Optimization techniques can help reduce the computational load of AI models, as done using pruning and quantization. When optimizing model accuracy while simultaneously minimizing size, organizations can enable the use of AI solutions at the edge.
Energy Consumption Trade-offs
Choosing a good compromise between the model's accuracy and consumption is incredibly daunting, even though high-performance models might require more power, and highly optimized models often sacrifice accuracy.
Solution: Adaptive Resource Management
Adaptive Resource Management can be described as workloads that alter the usage of resources in devices based on demand. Other discursive techniques, like adaptive voltage and frequency scaling (AVFS), are useful in manipulating the technology's performance and power demands in real time.
Data Privacy and Security
This is important as edge devices often meet private or sensitive data most of the time.
Solution: End-user computing, or endpoint computing, enables data to be processed on the device from which it was captured. Federated learning is one of the subcategories of on-device processing techniques used when training an ML model.
To maintain users’ privacy, it is appropriate to execute decisional procedures at the device level and perform federated learning to deliver AI execution. Organizations can enact privacy and maximise results by combining data fragmentation and knowledge.
Interoperability Issues
This increases the interoperability issues arising from the varying hardware and software platforms characterizing edge devices.
Solution: Standardization and open framework. As mentioned and pointed out earlier, change occurs in degrees. It is influenced by many factors, such as precise and equal distribution, which promotes standardization between edge devices, while open frameworks like ONNX can improve compatibility.
Breakthroughs in Low-Power AI Tech
As low-power AI solutions continue to evolve, several trends are shaping their future:
Edge AI Ecosystems
Complex-edge AI solutions integrating hardware, software, and networking are being developed. These ecosystems facilitate strong partnerships between device makers, software creators, and service-delivering parties.
AI in Wearable Technology
Smart clothing noninvasively incorporates low-energy AI for health and fitness and real-time telemetry. User health and well-being are major reasons for the ideas of AI-based wearable fashion and the enhanced usability and effectiveness of wearables while wearing them.
Integration of 5G and Edge AI
The set of 5 G networks is expected to expand the roles of edge AI solutions. Further, 5G can support advanced AI data affairs with higher MI, low latency, and vast bandwidth.
Figure 3: Comparing 4G and 5G
Future of Low-Power AI Solutions for Edge Devices
The future of low-power AI solutions for edge devices is promising, with several factors driving growth and innovation:
- Advancements in Hardware
Furthermore, improvements in further differentiating hardware systems like AI accelerators and efficient power consumption circuits will improve low-power AI solutions. These innovations will help to scale the use of even larger AI models while keeping efficiency high.- Focus on Sustainability
Improving energy efficiency to oracle sustainable growth goals will lead to the incorporation of low-power AI. There will be emerging priorities where solutions are environmentally friendly and provide insights about automated solutions.- Further R & D
Current research studies will reveal future developments of low-power AI techniques, architectures, and algorithms. Close collaboration between academia, industry, and research will drive the development of new technologies and the faster introduction of radical solutions.- Real-World Applications
With organizations beginning to understand the numerous application possibilities for low-power AI, we will inevitably see a craze from the simplicity that we have noticed thus far. More success stories will be demonstrated to demonstrate the changes in using these solutions in different sectors; therefore, their use will continue to increase.
Key Takeaways
Conventional low-power AI for smart devices effectively changes smart AI solutions as they encapsulate intelligent applications and solve core problems tied to power consumption and low computing capability. With the help of model optimization tricks, proper use of hardware and software, and efficient algorithms, it is possible to use AI for an organization’s needs without any negative impacts on devices and user experience.
In terms of future development, improvements in low-power AI solutions will transform industries and revise how people interact with products. They range from health care, production lines, homes and even agriculture. Anyone who could think of an application has an endless pool. Nonetheless, there will always be obstacles to deploying low-power AI solutions that continue to develop and improve to become more integrated into our existence regarding intelligent connectivity. There are tremendous opportunities for low-power AI at the edge femtosecond from now, reinforcing our world through intelligent simplicity, efficiency and sustainability.
Learn More About How Edge AI is Powering the Future of Smart Cities Discover more Edge AI vs Federated Learning