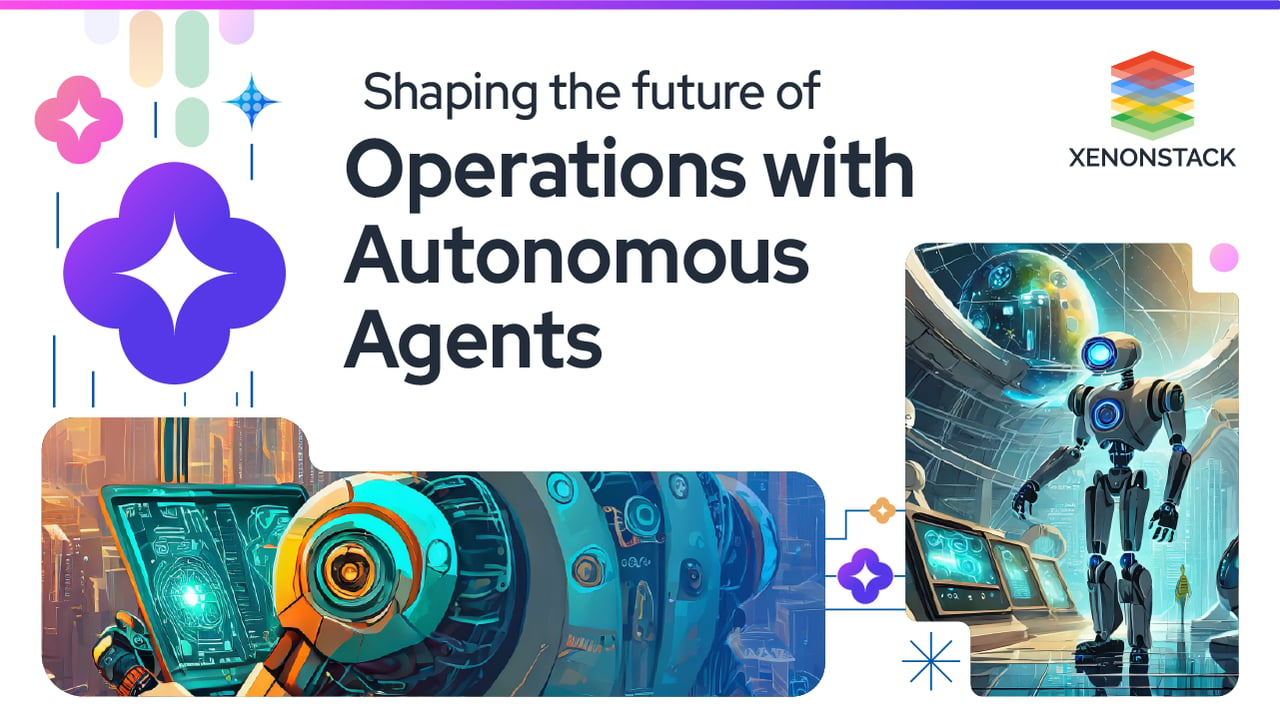
Fundamentals of Operations
Operations management is at the core of any organization. It ensures efficiency, productivity and smooth workflows. We’re on the brink of a technological breakthrough, and the introduction of autonomous agents into operations is changing the way businesses manage their operations. We’ll cover some of the key ideas in operations management and explore what challenges and opportunities autonomous agents present.
Operations management's core is the development, implementation, and enhancement of business processes that generate and deliver goods and services. Operations cover everything from manufacturing to supply chain to inventory management and beyond. AI and machine learning-powered autonomous agents are now playing a critical role in optimizing these operations.
Overview of Operations Management
Operations management is a set of processes that focus on making the best use of resources to achieve organizational goals. With the help of autonomous agents, you can perform tasks like demand forecasting, inventory optimization, and quality control with unprecedented accuracy. Intelligent systems can adjust in real time to keep up with changing market conditions.
Key Concepts and Principles in Operations
1. Data-driven decision-making: Data is at the core of operations management, and autonomous agents leverage huge data sets to make better decisions, resulting in better overall performance.
2. Collaboration between Humans and Machines: Autonomous agents and human labor work together to create an environment of harmony, where each contributes to the other’s strengths.
3. Automation and Efficiency: Autonomous agents streamline routine tasks, allowing human resources to focus on strategic decision-making and creative problem-solving.
Challenges and Opportunities in Operations
1. Integration Complexity: Autonomous agents must interoperate with legacy systems, and while this may seem like a tall order for businesses, overcoming this hurdle opens up unprecedented business opportunities.
2. Workforce Adaptation: As autonomous agents take on routine tasks, the workforce needs to adapt to focus on higher-level skills such as problem-solving, creativity, and collaboration. Upskilling becomes imperative for employees to thrive in this evolving landscape.
3. Data Security and Ethics: With the growing dependence on data, information security and privacy become top priorities. Organizations need to implement strong policies to protect sensitive data and follow ethical AI practices.
Technologies for Autonomous Agents
The integration of autonomous agents into operations relies on cutting-edge technologies that empower these intelligent systems to learn, adapt, and communicate effectively.
Machine Learning and Artificial Intelligence
Machine Learning (ML) and Artificial Intelligence (AI) are foundational technologies for autonomous agents. Autonomous agents are powered by ML and AI. These technologies allow agents to analyze large data sets, identify trends, and make decisions without the need for explicit programming. ML algorithms within autonomous agents facilitate predictive analytics, aiding in forecasting demand, optimizing inventory, and anticipating operational challenges.
Reinforcement Learning for Autonomous Agents
Reinforcement Learning is a branch of Machine Learning (ML) that trains agents to make decisions in a sequence in a dynamic environment. In reinforcement learning, agents learn by reacting to their environment and getting rewarded or penalized for doing so. Reinforcement learning is an ongoing learning process that enables agents to adjust to changing operational environments and make better decisions.
Deep Learning and Neural Networks
Deep learning, particularly neural networks, enables autonomous agents to carry out high-level operations by learning complicated patterns from data. It is used for image processing, NLP, etc.
Deep Learning, a subset of Machine Learning (ML), concentrates on training Deep Learning Networks (DNNs) to address intricate challenges. Unlike traditional ML, neural networks are computational models inspired by the brain's structure and functions. Deep Learning empowers autonomous agents to acquire hierarchical data representations, extract crucial insights, and form high-level abstractions. Neural networks have demonstrated significant advancements across diverse domains like image processing, natural language processing, and speech synthesis.
Natural Language Processing for Agent Communication
Natural Language Processing (NLP) enables autonomous agents to comprehend and create natural language. This is essential for agent-to-agent communication, agent-to-operator interaction, and user experience. Natural Language Processing (NLP) allows agents to understand and act on verbal and written inputs, resulting in more natural and user-friendly interactions within the operating environment. Natural Language Processing (NLP) allows autonomous agents to interact with people and other agents through natural language processing. This allows for smooth interactions and collaboration, allowing agents to get directions, explain information, and negotiate tasks.
Future Trends
1. Exponential Technological Advancements: The autonomous agents of future will leverage cutting-edge technologies such as AI, ML and robotics to solve increasingly complex problems with increased efficiency.
2. Edge Computing Integration: The rise of edge computing and the Internet of Things (IoT) is poised to greatly influence autonomous agents.
In addition, autonomous agents will increasingly use edge computing to process data on-premises, allowing them to make faster decisions and reduce reliance on centralized systems.
3. Multi-Agent Systems and Collaboration: Multi-agent systems will allow autonomous agents to work together to make decisions, resulting in more flexible and collaborative operating environments.
Conclusion
However, autonomous agents come with their own set of unique challenges. To train and evaluate agents, organizations must carefully collect and preprocess data, as well as carefully select algorithms. Ethical and social considerations, as well as explainability and transparency, become increasingly important when autonomous agents make important decisions. The future of autonomous agents will also be shaped by collaboration in multiple-agent systems, as well as integration with new technologies such as edge computing and the Internet of Things (IoT). Organizations must approach autonomous agent development and deployment with ethical and social considerations, fairness, transparency and accountability, as well as compliance with regulations and guidelines, to harness the power of autonomous agents to bring about positive change and significant value across different industries. As autonomous agents continue to develop, it is important to stay up to date with the latest advances and insights.
-
Click to explore about Large Language Models in AI Agents