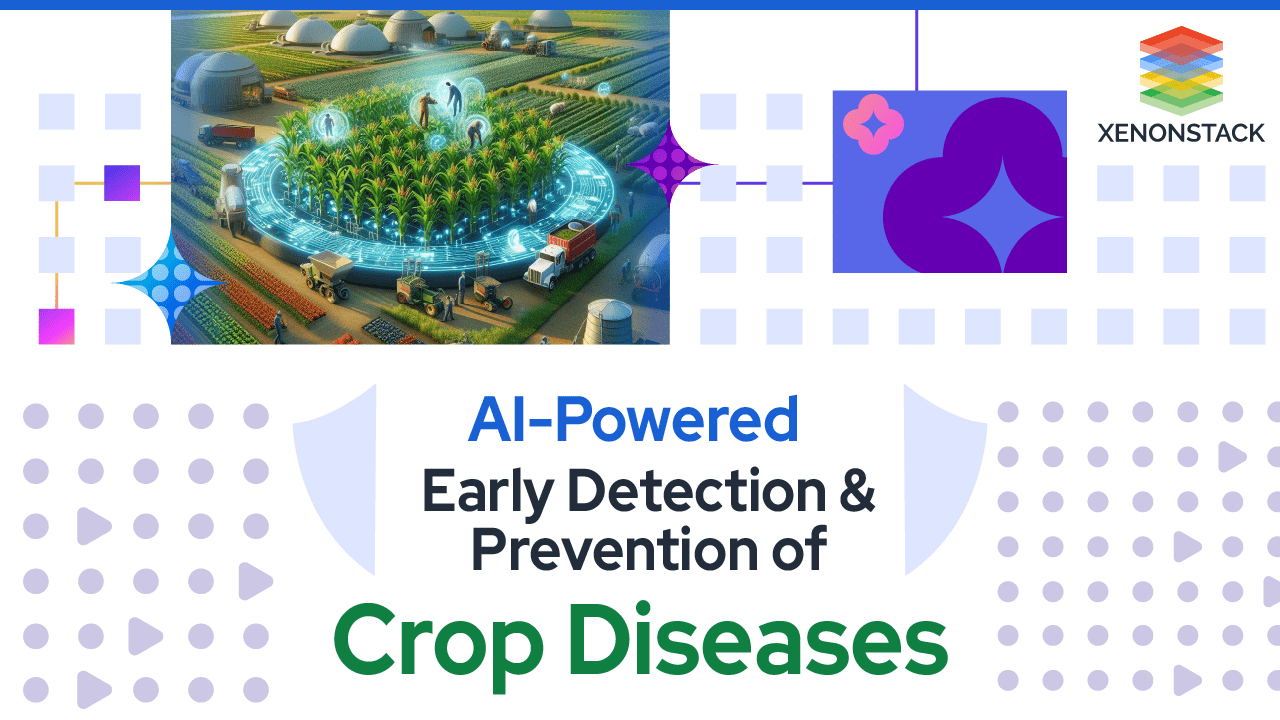
Challenges and Opportunities in AI-Driven Detection
What are the challenges?
While AI-based crop disease detection holds immense potential, several challenges must be addressed to realize widespread adoption and effectiveness.
-
One significant challenge is the availability of high-quality training data. Building annotated datasets that capture the variability of crop diseases in different regions and under various growing conditions can be resource-intensive and time-consuming.
-
Moreover, ensuring the robustness and generalizability of AI models across different crops and environmental factors remains a persistent challenge.
What are the opportunities?
However, despite these challenges, the opportunities presented by AI in crop disease detection are vast.
-
Rapid advancements in imaging technologies, such as drones and satellite imagery, enable large-scale monitoring of agricultural landscapes, facilitating early disease detection over vast areas.
-
Additionally, the integration of AI with Internet of Things (IoT) devices allows for real-time monitoring of plant health parameters, such as temperature, humidity, and soil moisture, further enhancing disease surveillance capabilities.
Prevention Strategies and Sustainable Agriculture
In addition to early detection, prevention remains the cornerstone of effective disease management in agriculture. Below are the strategies we can use for the prevention:
1. Integrated pest management (IPM) - Farmers can decrease their dependence on synthetic pesticides and lower the chances of disease outbreaks by incorporating integrated pest management (IPM) strategies and embracing agroecological methods.
2. AI technologies - AI technologies complement these efforts by providing decision support tools that optimize resource allocation and help farmers implement targeted interventions.
3. Predictive models - AI-powered predictive models can forecast disease outbreaks based on weather patterns, historical data, and environmental factors. Armed with this information, farmers can adjust planting schedules, select disease-resistant crop varieties, and deploy biocontrol agents to mitigate the risk of disease establishment.
4. AI-driven precision agriculture techniques – These methodologies allow for the accurate utilization of inputs like fertilizers and pesticides, reducing environmental harm while optimizing crop well-being and yield.
Conclusion
Crop diseases pose significant challenges to global food security, but with the advent of AI technologies, farmers now have powerful tools to combat these threats. By harnessing the capabilities of machine learning and computer vision, researchers and farmers can detect diseases early, implement targeted interventions, and promote sustainable agricultural practices.
However, realizing AI's full potential in crop disease detection requires concerted efforts to address challenges related to data availability, model robustness, and equitable access to technology. With continued innovation and collaboration, AI holds the promise of transforming agriculture into a more resilient, efficient, and sustainable endeavor, ensuring a brighter future for generations to come.
Know more about Multimodal AI for Enhanced Image Understanding Explore more about Utilizing Artificial Intelligence for Customer Query Management