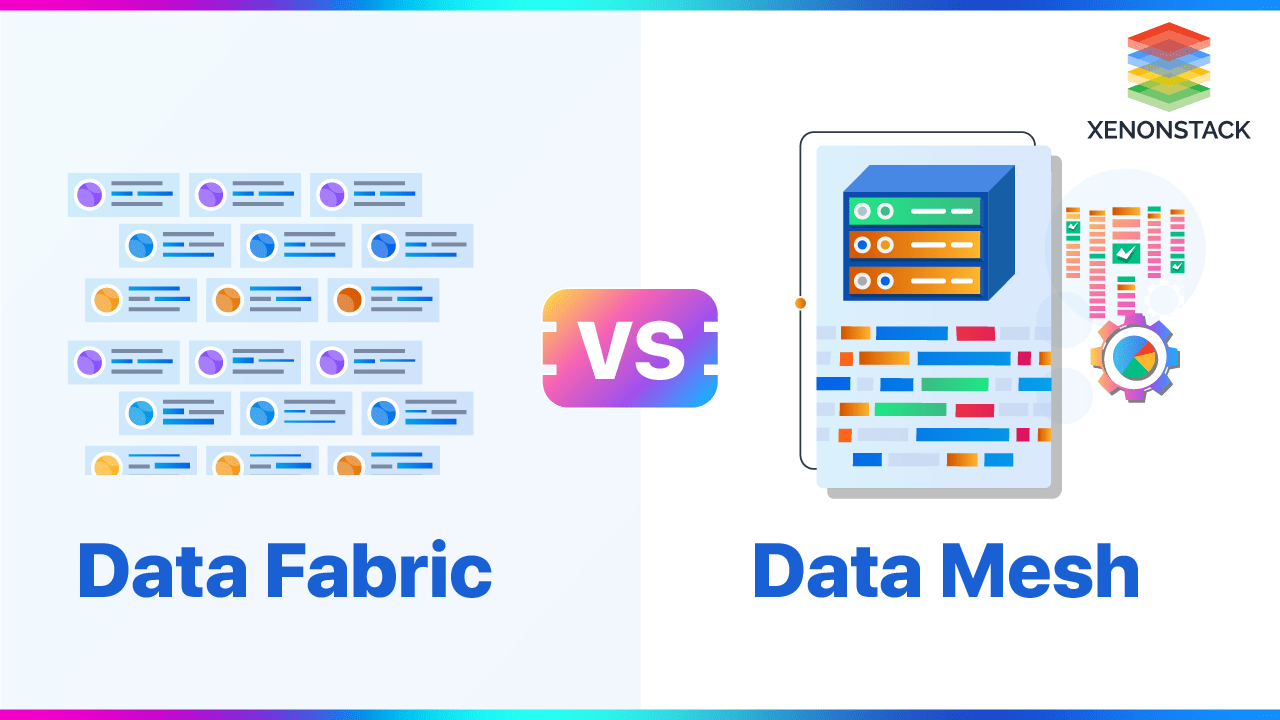
Introduction to Data Fabric and Data Mesh
In the hyper-connected world of the cloud and the Internet of Things (IoT), each processing network gadget is associated with one more through a perplexing, interconnected network. Current ventures attempt to become information-driven associations and get more business esteem from their information. However, the ascent of the cloud, the Internet of Things (IoT) development and different elements imply that notification isn't restricted to on-premises conditions. This represents a genuine test for future Data Management, as a definitive objective of Data Management is sharing business information across different stages and innovations.
The terms "data mesh" and "data fabric " are frequently utilized reciprocally to demonstrate data-access architecture in a hyper-associated Data Management world. Data fabric is more of an architectural approach to data access, whereas the mesh connects data processes and users. Some of the core principles of data mesh architecture are:
1. Domain-oriented data ownership and architecture
2. Data as a product
3. Self-service data platform
4. Federated computational governance
Data fabric should work with various data delivery methods (including, but not limited to, ETL, streaming, replication, messaging, and data virtualization or data microservices).Read more about Big Data Fabric Implementations and Its Benefits
What You Will Achieve: Centralized vs. Decentralized Data Platforms
A centralized data architecture implies that every domain/subject (for example, finance, operations) is duplicated to one area (for example, a data lake under one account). It likewise implies centralized responsibility for data (typically IT). The information from the different domains is joined to make centralized data models and bring together perspectives. This is the methodology utilized by a Data Fabric.
A decentralized distributed data Fabric vs. data Mesh data architecture means the data from each domain isn't duplicated but instead kept inside the field (each domain/subject has its data lake under one account). It also means distributed data ownership, with each part having its owner. Each field has its data models.
Explore more about the challenges faced by data architecture.
Specific Applications: Exploring Data Fabric with Examples
A data fabric is an architecture that runs technologies and services to assist a corporation in managing its data. This data can be stored in relational databases, tagged files, flat files, graph databases, and document stores.
A data fabric architecture facilitates data-centric tools and applications to access data while working with varied services. These can include Apache Kafka (for real-time streaming), ODBC (open database connectivity), HDFS (Hadoop distributed file system), REST (representational state transfer) APIs, POSIX (portable operating system interface), NFS (network file system), and others. It's likewise crucial for a data fabric architecture to support rising standards.
A data fabric is agnostic to architectural approach, geographical locations, data use case, and process and deployment platforms. With data fabric, associations can pursue meeting one of their most wanted objectives: approaching the correct information progressively, with a start-to-finish administration, and for a minimal price.
Example of Data Fabric
The adaptability of a data fabric architecture helps in many ways than one. Some of the data fabric examples include the following:
Enhancing Machine Learning (ML) Models
When the correct data is taken care of by AI (Machine Learning) models without wasting time, their learning capacities improve. ML calculations can screen data pipelines and suggest reasonable connections and incorporation. These calculations can get data from data associated with the data fabric, go through all the business data, inspect that data, and distinguish relevant associations and connections.
Organizations can utilize a data fabric to outfit data from client exercises and see how cooperating with clients can offer more worth. This could incorporate uniting constant data of various deals exercises, the time it takes to obtain a client locally, and consumer loyalty KPIs(key performance indicators).
Use Cases in Action: Understanding Data Mesh through Examples
The Data Mesh is a new approach based on a modern, distributed architecture for analytical data management. This decentralized technique distributes data ownership to domain-specific teams that manage, own, and serve the data as a product. It empowers end clients to effectively access and query data where it resides without shipping it to a data lake or warehouse.
Research more regarding data warehousing.
Example of Data Mesh
Data mesh upholds various analytical and operational use cases across different spaces. The following are a few examples.
1. Customer 360 views
It helps client care diminish average handle time, increase first contact resolution, and develop consumer satisfaction. A solitary perspective on the client may likewise be deployed by marketing to predictive churn modeling or next-best-offer decision.
2. Hyper segmentation
Enables marketing teams to deliver the proper mission to the right client at the ideal opportunity and utilize the right channel.
3. Data privacy management
To protect customer data by complying with ever-emerging regional data privacy laws, like VCDPA, before making it accessible to data consumers in the business domains.
Data meshes address the shortcomings of data lakes by giving data owners more autonomy and flexibility, allowing for more data experimentation and innovation, and reducing the burden on data teams to meet the needs of every data consumer through a single pipeline.
Key Differences: Comparing Data Fabric and Data Mesh
A Data Mesh and a Data Fabric give an architecture to get data across numerous platforms and technologies. Still, a Data Fabric is technology-centric, while a Data Mesh centers around organizational change.
In the Data fabric, the data access is centralized (single point of control), for example, a rapid server cluster for network and superior resource sharing. On the other hand, in a Data Mesh, the data is stored within each unit (domain) within a company. Each node has a local storage and computation power in a distributed Data Mesh, and no single point of control (SPOC) is necessary for operation. In a Data Mesh climate, original information stays inside areas/domains; duplicates of datasets are created for clear use cases.
Data Fabric leverages automation finding, associating, perceiving, proposing, and conveying information resources for customers dependent on a wealthy endeavor metadata establishment (e.g., a knowledge graph). Data mesh depends on data domain owners to drive the requirements upfront for data products.
1. Data Fabric over Data Mesh
In Data Mesh, Data integration across many enterprise source systems often requires domain-specific expertise in data pipelining; using data fabric, domains do not need to deal with underlying source systems. At the point when a data product is a business entity managed in a virtual data layer, there's no need for domains to deal with underlying source systems.
The Data Mesh's fully distributed data management practice is sometimes a recipe for chaos, silos, and lack of adherence to standards and global identifiers.
Data fabric can be built without adopting a data mesh architecture. Data mesh must depend on the data fabric's discovery and analysis principles to create data products.
Learn about why or why not to adopt data mesh.
2. Data Mesh over Data Fabric
Data products are based on product usage patterns in Data Fabric, whereas in Data Mesh, Data products are designed by business domains and original Data.
Data Fabric uses artificial intelligence to generate data semantics and perform data integration automatically, whereas humans do the same.
It can be good if context and implicit knowledge, critical in understanding a dataset, are best done by human domain experts. Data Mesh may result in fewer silos because it is easier to make datasets available to other teams. As long as they are appropriately incentivized, data product owners will try to integrate their products with the other datasets within the enterprise.
Deep study about data fabric implementations.
Benefits Unpacked: Advantages of Data Fabric and Data Mesh
The benefits of data fabric and data mesh are mentioned below:
1. Benefits of Data Fabric
A. Data scale, volume, and performance: Dynamically scale up and down seamlessly, regardless of the data volume. It supports both operational and analytical workloads at an enterprise scale.
B. Accessibility: Support all data access modes, sources, and types, and integrate master and transactional data at rest or in motion.C. Distribution: Data fabric is deployable in multi-cloud, on-premise, or hybrid environments.
D. Data Integration: Improve data integration between applications and sources.
2. Benefits of Data Mesh
A. Business Agility and Scalability:- It powers decentralized data operations, independent team performance, and data infrastructure as a service provision, improving time-to-market, scalability, and business domain agility. It eliminates the process complexities and IT backlog to reduce operating and storage costs.B. Faster Access and Accurate Data Delivery:- It offers easily governable and centralized infrastructure based on a self-service model without underlying complexity for faster data access and accurate delivery.
C. Sales and Marketing Benefits:- The distributed data enables sales and marketing teams to curate a 360-degree perspective of consumer behaviors and profiles from various systems and platforms to create more targeted campaigns, increase lead scoring accuracy, and project customer lifetime values.
D. AI and Machine Learning Training:- Enable development and intelligence teams to create virtual data warehouses and data catalogs from different sources. So that they can feed machine learning (ML) and artificial intelligence (AI) models to help them learn without consolidating data in a central location.
E. Loss Prevention and Low Costs:- Data mesh implementation in the financial sector creates faster time-to-insight at lower operating costs and operational risks.
Conclusion: Summarizing Insights and Next Steps
Data mesh is more about people and processes than architecture. In contrast, a data fabric is an architectural approach that tackles the complexity of data and metadata in a savvy way that functions admirably together. We can see more Data Mesh deployments in the near term since the Data Mesh can be implemented today with existing technology. At the same time, the Data Fabric depends on additional improvements in AI-driven data integration before it can genuinely take off.
Get more information related to data mesh architecture.
- Enow more about Master Data Management in Banking Sector
- Discover more about Data Quality Management
- Explore further about Data Management with Intelligent Data Agents