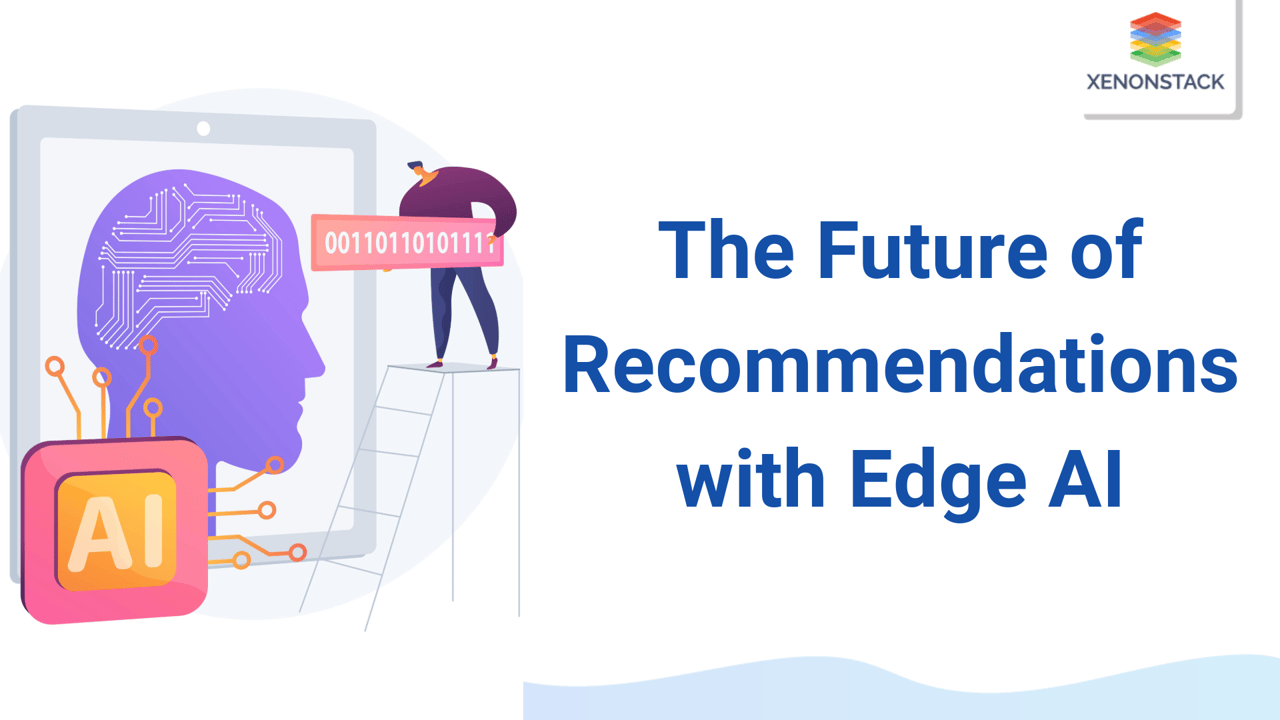
Edge AI Applications in Personalised Recommendations
Retail and E-Commerce
The concept of Edge AI finds application in improving the customer journey online and in physical stores. Applications include:
-
In-Store Recommendations: Edge AI in smart mirrors and shopping kiosks can help shoppers pick out clothing items based on a consumer's history.
-
Dynamic Pricing: A customer-specific price list is created in real-time based on factors such as the customer’s frequency of purchasing, his/her history of buying habits, and prevailing market conditions.
-
Offline Functionality: E-commerce applications integrated with Edge AI can operate normally with or without an internet connection and offer products and deals related to the data stored locally.
Entertainment and Media
Streaming platforms like Netflix and Spotify are pioneers in personalization, and Edge AI takes their capabilities to the next level:
-
Localised Recommendations: These recommendations are based on a user’s mood, popularity in specific video categories, or time of day.
-
Bandwidth Optimisation: Edge AI stores and processes data locally, so there is no interruption resulting from video buffering.
Healthcare
In the healthcare industry, personalisation can significantly improve patient outcomes:
-
Wearable Devices: Elite wearables, such as smartwatches and fitness trackers, pull information from such systems in real-time to provide information about exercise, bedtime, or further suggestions about nutrition.
-
Remote Monitoring: This application of Edge AI in medical devices means that large amounts of patient data can be analysed in real time, and alerts for timely interventions can be made without invading patients' privacy.
Smart Homes and Iot
Edge AI enhances the intelligence of smart home ecosystems:
-
Customised Automation: Smart thermostats, bright lights, or smart speakers, for example, get accustomed to user habits to develop their smart schedules.
-
Energy Management: Automated energy systems can recommend specific patterns of energy utilization to save money on electricity consumption while maintaining comfort.
Automotive
Connected vehicles use Edge AI to provide personalized driving experiences:
-
Navigation: A real-time adaptive recommendation of routes to a driver, depending on the road's current situation and given the driver’s preference.
-
In-Car Entertainment: Having their very own personalized media and climate.
Advantages of Edge AI for Personalisation
-
Enhanced User Experience
Using Edge AI yields many targeted and timely recommendations because it allows us to respond quickly to users’ actions and provide much-needed real-time context. -
Improved Privacy and Security
Breach security: Since data computation and processing occur locally on the edge devices, no data has to be transported over the network. This avoids violating modern data protection regulations such as GDPR and CCPA. -
Cost Efficiency
It benefits businesses with cloud storage and bandwidth costs, but users also get very good services. -
Scalability
They are not Symplex prone, which means they do not burden a central server and can, therefore, be deployed in millions of devices, particularly for organisations with large clientele. -
Energy Efficiency
Handling data locally is more energy-efficient than sending it to the cloud to be processed, contributing to green artificial intelligence.
Overcoming Challenges in Edge AI
While Edge AI offers numerous advantages, it also comes with challenges:
-
Hardware Constraints: Computational requirements are high in AI models, and edge devices have restricted computational capacity and storage.
-
Model Optimisation: Designing efficient AI models for edge computing devices is difficult.
-
Interoperability Issues: Interoperability is one of the biggest concerns in the design of two or more distinct Platforms.
-
Data Fragmentation: Locally designed algorithms might also result in different conclusions observed on different devices.
Future Trends in Edge AI for Personalization
- Federated Learning: This technique enables AI models to be trained across different devices without the need to transfer original data to a central server for analysis, thereby protecting personal data while improving the ability to personalize data for the user.
- AI-Powered IoT Networks: Therefore, as IoT ecosystems grow, Edge AI will empower more intelligent and consistent interconnective devices, giving a unified persona experience.
- Explainable AI: Edge AI systems have multiple use cases for explainable models, enabling the system's users and developers to understand how various recommendations are made. This helps improve the trust that delegates to these smart systems.
- Cross-Device Synchronization: Subsequent iterations of our Future Edge AI will automate engaging, device-specific content curation more holistically across smartphones, smartwatches, and other home appliances.
- Edge AI in Emerging Markets: With the expansion of the availability of the latest hardware at lower costs, Edge AI will be accessible to everyone, including emerging markets, consumers, and businesses.
Ironically, Edge AI is a giant leap towards personalisation and recommendation systems. This processing approach helps AI convey quicker, secure and context-aware outcomes, which is what today’s consumer desires. Across industries as diverse as retail and healthcare, Edge AI is at the centre of all the exciting changes in how companies engage their customers. Indeed, as we go up the hierarchy, one can expect further developments toward federated learning, explainable AI, and instantaneous transfer of personalized elements between devices.
The next wave of personalisation happens at the edge, meaning that, in addition to reacting to our wants and requirements, intelligent systems proactively offer exactly what we may require with superb accuracy. This means Edge AI is more than a nice idea for businesses wanting to remain relevant. It is imperative. Thus, on the edge is not just where technology works, but it is where the future of personalisation starts.
Explore more insights and blogs to stay ahead in the world of AI and innovation: