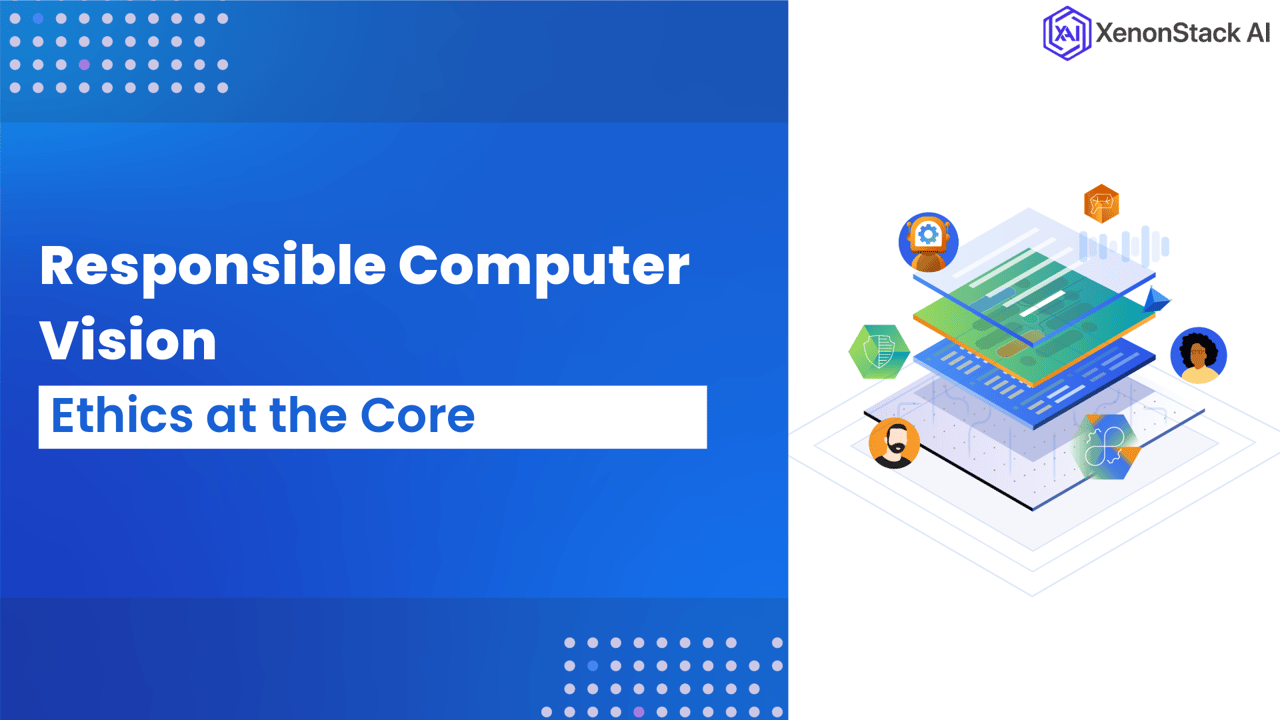
Who controls the ownership of images and videos depicting you or your property? How should they be used—if at all? And how do individuals provide consent for the use of personal data or even discover where it is being stored?
According to McKinsey, computer vision ranks second among all AI solutions in terms of adoption. Statista forecasts the computer vision market to grow at an annual rate of 11.69%, reaching $50.97 billion by 2030
As AI-powered technologies increasingly penetrate various aspects of life, this unchecked expansion can feel invasive. Tools like computer vision and facial recognition rely on personal data—such as physical appearance, location, habits, and behaviour—to deliver innovative services that enhance our lives in ways once unimaginable. However, this reliance on personal data raises significant ethical concerns around privacy, discrimination, and security.
In this blog, we explore these critical issues and propose strategies to minimize risks while maximizing the benefits of computer vision—ensuring technological advancements come with responsible stewardship of personal information.
Key Takeaways
- Ownership and Consent: Using personal images without consent raises privacy concerns.
- Bias and Discrimination: Algorithms can reinforce biases; diverse training data is essential.
- Legal and Ethical Obligations: Organizations must adhere to data protection laws and secure informed consent.
- Transparency and Accountability: Developers should communicate data collection methods and potential risks.
- Privacy-Preserving Practices: Techniques like homomorphic encryption can protect data privacy while allowing analysis.
- Collective Responsibility: Stakeholders must collaborate to create ethical frameworks that foster public trust.
The Cognitive Power of Computer Vision
Computer vision combines two advanced technologies— deep learning and Convolutional Neural Networks (CNNs)—to analyze and interpret images. Deep learning, a branch of machine learning, leverages algorithms trained on vast datasets to evaluate new images. These algorithms are continuously refined by adjusting the neural network’s parameters, enhancing performance over time.
CNNs define how the neural network processes information, breaks down images into individual pixels or objects, labels them, and performs “convolutions”—a series of predictions, accuracy checks, and adjustments. This iterative process continues until predictions align with actual outcomes, mimicking human brain function.
The computer vision-based technology that detects and analyzes human posture. Taken From Article, Human Pose Estimation for Sport Analytics
Ethical Challenges in CV
In Public dataset
What is important to note is that to train and test algorithms and, therefore, design efficient Computer Vision systems, public datasets are crucial. As has been deduced above, using these datasets entails great ethical issues in most cases. Most datasets contain images of individuals to whom owners cannot seek permission to use the images, and this raises serious privacy concerns that one cannot avoid. However, it is the case that such datasets might often embed/reinforce existing biases of society, and the models created from them may, thus, amplify such biases.
For example, the training of algorithms using a limited dataset leads to the facial recognition systems underperforming, particularly when detecting coloured people. It raises very basic issues of equity and societal welfare in applying AI technologies to enhance the delivery of healthcare services. Quantify the risk of privacy breaches.
- N is the no.of points in the dataset
- P(Reidentification /DataPoint i)PReidentification /DataPoint i
- The probability that an individual can be re-identified from data points
Individuals whose images are included may be entirely unaware of their involvement, the intended use of their data, or the entities that will utilize it. This lack of transparency and accountability poses ethical challenges, particularly when such technologies are applied in sensitive contexts like surveillance and law enforcement. Addressing these concerns is essential for fostering trust and accountability in AI technologies, ultimately paving the way for their responsible use in society.
Other challenges
Facial recognition is one of the examples of biometrics that can be highly beneficial, but at the same time, It has numerous difficulties and problematic issues. Here’s a closer look at some critical challenges:
-
Fraud: Crooks and spammers have identified the essence of this approach by wearing masks or duplicating images of individuals to fake their identities. During the pandemic, over 80,000 attempts were made to fraudulently obtain state unemployment benefits using government systems. Some of the questions that the Wall Street Journal raised between June 2020 and January 2021 showed the weakness in those technologies.
-
Bias: Facial recognition is not created equal. Research shows that a higher false identification rate among black and Asian individuals compared to whites can lead to false arrests. Also, it is likely to confuse an elderly person or a child and a middle-aged person, often shifting investigations and arriving at wrong conclusions. Therefore, cities such as Baltimore and Portland have prohibited using this technology.
-
Inaccuracy: The risks are even bigger in healthcare. Based on the information received, the external signals and data noise are responsible for the mistakes in the diagnosis. For instance, one system provided health predictions based on the type of X-ray machine, providing portable machines for a certain disease because the patients who use them are sicker, as a rule. It also illustrates and importantly demonstrates the use of data analysis gained within organizations.
-
Legal Consent Violations: Facial recognition in the private sector has become a questionable legal practice that has warranted the following consequences. Businesses have harvested personal data without permission, thus infringing on the Illinois Biometric Information Privacy Act and the California Consumer Privacy Act. These have created several class-action lawsuits and cries from the public domain. Even to tackle privacy concerns, Apple postponed releasing CV accompaniment software that is used to identify child sexual abuse material (CSAM) in personal devices.
-
Ethical Consent Violations: The problem of facial recognition impacts ethical issues that go beyond the legal provisions. Scientists accumulate large collections of facial images without adequate permission; they use data collected from the internet to improve the software used for surveillance. This practice apparently has very important ethical implications, especially when personal information is processed for military and commercial ends unknown to the users. For instance, such as work done among the Muslim Uyghur of China, work done among this group of people was met with a lot of criticism, some within the scientific community even demanded the withdrawal of such research.
Ethical principles in CV 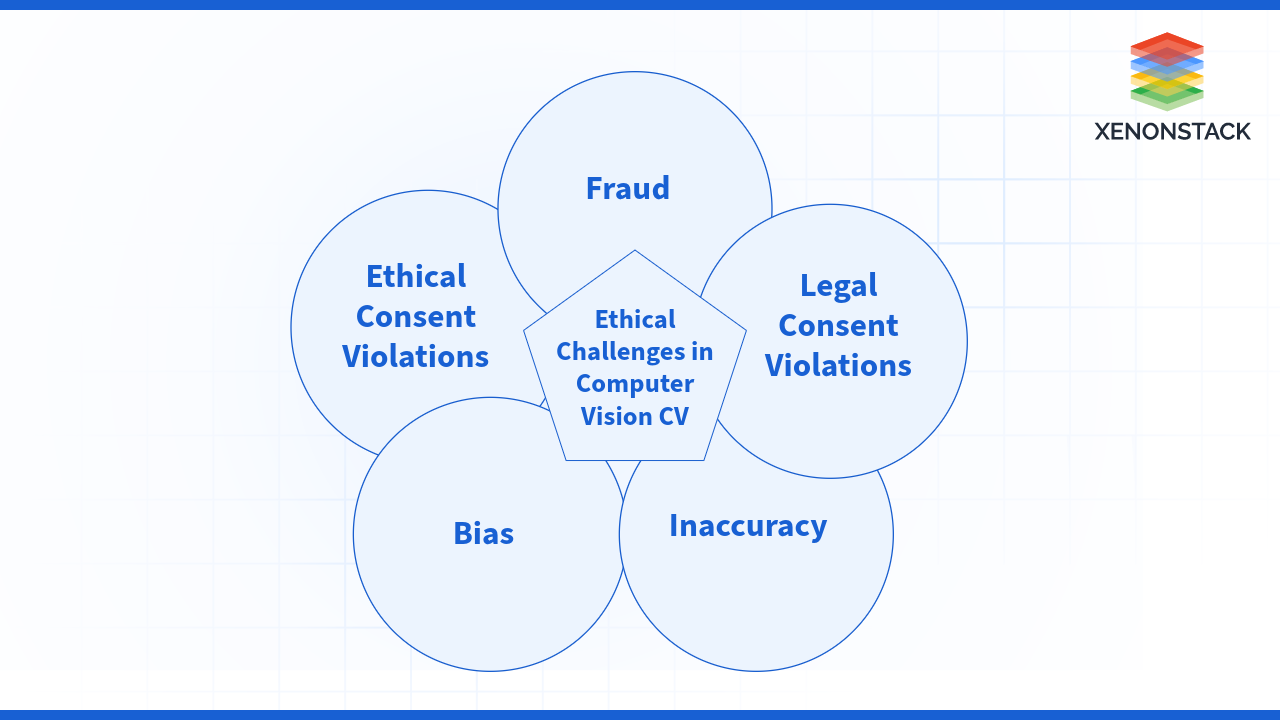
Respect for Human Dignity and Privacy
Computer vision technologies should be created or implemented to address the ethical development of human rights and their privacy privileges. This commitment involves protecting people’s private parts throughout the data life cycle, from the collection of samples to the provision of models. The measures include asking for consent, particularly where it is possible, and always refraining from identifying subjects or using their pictures in any way that may harm them.
Informed Consent
Getting and involving the patient’s consent is very central to human dignity. It helps make people aware of how their pictures will be used and something as simple as saying no if that is what they want. However, getting informed consent can be quite a difficult endeavour, especially when working with big data, which may contain images gathered randomly or intrusively from social media or other domains where a subject’s consent cannot be sought. This issue becomes more complicated, especially when the pictures are used outside their original intended purpose, for example, for business purposes or for research satisfying the expectations of the people involved.
Anonymization Techniques
Data anonymization, also known as data masking, is a practice that ensures that individuals' information is not compromised. Anonymization is usually done to eliminate (PII) from the datasets. Anything that involves erasing human faces, removing metadata, and replacing original pictures with fake images can be done in computer vision. But it is important to understand here that there is no complete substitute for anonymity. Specifically, current developments in re-identification raise new threats to individuals’ anonymity, and therefore, frequent update of anonymization techniques, coupled with periodic data checks, is required. The formula for anonymity success:
Transparency in Data Usage
However, to get to that position, transparency is inevitable for Computer Vision Systems. The users need to be informed on how data is being collected, processed and used through reporting by the developers. These include the explanation of various aspects, which include the ethical issues that are coming from the creation of the datasets as well as the actions that have been taken to try to eschew privacy issues that take place while using the data and any negatives that may come from the use of such data.
Bias Prevention and Fairness
It is highlighted that computer vision models need care and precautions to reduce bias and unfairness right at the conceptualization phase. There are numerous ways through which bias can creep into a data set, including sample bias, where the data represents either too many or too few people from one community, biased labelling, or using a skewed training sample from which the general population data is drawn. Regarding model training, the availability of more biased datasets will only deepen or prolong social imparity.
One way to measure bias in classifiers is through the Disparate Impact (DI) ratio:
-
Y Y is the predicted outcome
- A is a binary-sensitive attribute (e.g., gender, race)
- P(Y=1 ∥ A= 1) is the probability of a positive outcome for the advantaged group
- P(Y=1 ∥ A= 0) is the probability of a positive outcome for the disadvantaged group
What Causes Bias?
Bias in Computer Vision Datasets can be derived in many ways. For example, if a data set contains mostly images of people with light skin, the models used to recognize people’s faces may be trained poorly for people with dark skin. Furthermore, certain attributes are applied unequally and, therefore, belong to definite populations and contribute to cultural bias.
-
Bias Identification and Alleviation: In the context of the present best practices, reducing bias is an important aspect since it is oriented toward providing fairness and equality. This process requires searching for deceptive trends within the datasets, applying ways to fix those trends, and evaluating model fairness before its release into the community. Based on the identified techniques, it can be inferred that re-sampling, re-weighting, and adversarial debiasing techniques may be useful in addressing both dataset and model bias problems.
-
Fairness Metrics: Researchers use different metrics to analyze fairness in computer vision models, such as Disparate Impact, Demographic Parity, and Equal Opportunity. These metrics provide a way of measuring how a certain demographic is treated by a model and using such information to build the right systems.
-
Ethical Implications: In computer vision, the problem of bias has serious ethical consequences. Traditionally, when there are biased models, there will be bias in various practices, such as in the misrecognition of faces in face recognition systems, which can be very disadvantageous for individuals involved and their societies. Eliminating bias is not simply a matter of solving scientifically and methodologically complex issues; it is a matter of professional conscience Simply treating bias as a methodological and scientific problem and employing ideas from the social sciences, ethics, and law is not enough. From the above ethical principles, we can ensure that computer vision technologies are developed correctly and properly.
Computer vision needs to be made more ethical as technology continues to improve.” Here’s how organizations and individuals can navigate this new landscape responsibly.
How to use computer vision Ethically
Improve Training Data
-
Diversity Matters: Ensure your training data reflects a variety of backgrounds to reduce bias.
-
Rigorous Review: Implement strong verification processes to catch and mitigate discrimination before it affects your models.
Match Technology to Need
-
Right Tool for the Job: Use only the technology necessary for your application. For instance, a simple headcount can be done without facial recognition, with less tech and fewer risks!
Define Clear Purposes
-
Set Boundaries: Clearly outline the intended use of your computer vision model.
-
Documentation is Key: Record how and why the technology is applied to ensure it stays within ethical boundaries.
Strengthen Privacy Protections
-
Compliance is Crucial: Stay updated on local laws to protect personal data and privacy.
-
Data Security: Develop robust data protection programs to guard against misuse.
Prioritize Informed Consent
-
Get Consent: Always seek informed consent before collecting facial images or personal data. This is not only ethical but often a legal requirement.
-
Panel Representation: In large studies, a representative panel is considered to voice the consent of wider populations.
Remember: Computer vision ethics is not an afterthought; it is a lifelong approach to the future of technology. In this way, it will be possible to implement the given approaches to effectively using computer vision without violating human rights or being unprotected from inequitable treatment.
Best Practices for Handling Sensitive Data in CV
Modern business is highly dependent on information and technology, so such industries as IT can use the most advanced practices to protect data. Here’s how:
-
Homomorphic Encryption: Think about processing data while keeping it secure! This is made achievable through homomorphic encryption, which allows one to compute encrypted data and yield secure results. These results can only be decoded by the users, who possess the necessary key, making it easier to secure sensitive information while transacting.
-
Secure Federated Learning: Now, you can know how to harness the value of decentralized data processing with Secure Federated Learning. It is innovative in that such independent nodes as devices and servers are used to control subgroups of information while not exchanging any raw data. This data is then integrated into a single ensemble machine learning model, further ensuring that each data set remains secure. This technology, developed and refined by Google, underlies features like the Gboard text prediction and the TensorFlow machine learning, showcasing that privacy and performance really are not mutually exclusive!
-
Secure Multiparty Computation: Join forces without compromising privacy through Secure Multiparty Computation! This method allows multiple parties to jointly compute inputs while keeping their individual data confidential. Eliminating the need for a trusted third-party server ensures that each participant’s information remains protected, fostering collaboration without risk.
The identified best practices not only increase data protection but also contribute to more trust in requested services among clients. Because of these developments, it becomes essential for tech-savvy companies to move to higher levels of enterprise privacy solution strategies to retain a competitive advantage and guard confidential data.
It’s time to put privacy first and make the world of tomorrow safer!
Final Thoughts
The advancement in Computer Vision Technology is progressing at a very high speed, revealing exciting new opportunities; however, ethics in this discipline imposes emerging questions that cannot be overlooked. The greater the extent of decision-making facilitated by automated systems, the more important privacy, decision-making responsibility, openness, risk of the presence of bias, and consent become. This paper concludes that without a framework for responsible self-governance to mediate between the potential for innovation and acceptability, rhetoric can quickly deteriorate into socially untenable solutions.
Thus, there is no one-to-one correspondence between roles and the system's complexity, but everyone must play his part. Technologists cannot, will not, and should not develop technologies irresponsibly; commercial providers must protect rights; governments must make policies and then govern them correctly to serve both innovation and public needs.
Solving these problems requires an effective approach, which this paper will try to offer. Computer vision can be achieved at its process-optimized capacity with its risks managed by intentionally creating voluntary standards, freely reacting to those pressures, developing agreed-upon practices, and regularly implementing legislative supervision.
Let’s seize this moment! By aligning advancements in computer vision with our shared values and ethical standards, we can ensure a brighter, more equitable future for all.
Read more about History of Computer Vision Explore the Workflow of Computer Vision