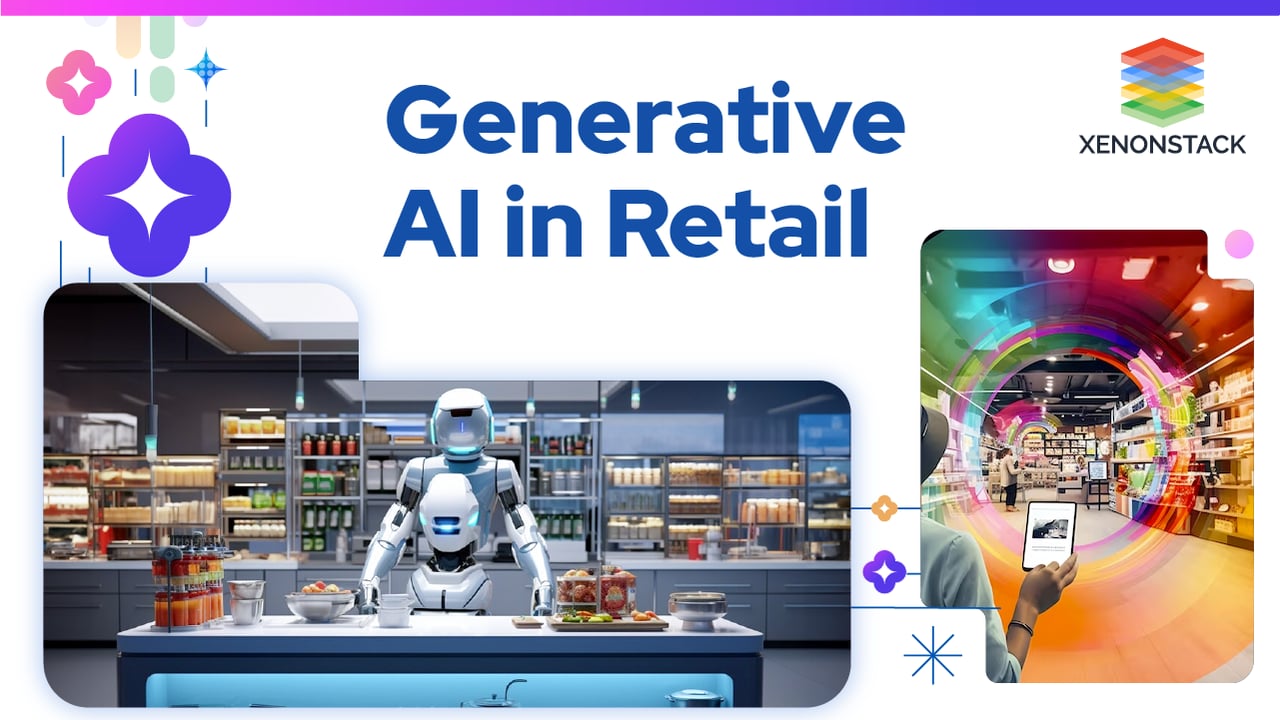
Benefits of Generative AI in Retail
-
Enhance Retail Efficiency with Generative AI - Generative AI helps retailers boost operational efficiency and reduce costs by automating routine tasks like returns, exchanges, and procurement. GenAI-powered chatbots and interactive training videos improve team member productivity and lower turnover.
-
Drive Customer Loyalty with AI-Powered Personalization - GenAI uses data from shopping histories, social media, and reviews to create personalized marketing campaigns at scale. This reduces brand fatigue, enhances content relevance, and strengthens customer retention.
-
Transform Customer Experience with GenAI - GenAI streamlines customer support through chatbots, instant access to customer histories, and team member assistance tools. It ensures faster, more accurate responses, improving customer satisfaction and loyalty.
-
Innovate Products with AI-Driven Insights - GenAI simplifies product development by analyzing reviews, social media posts, and call centre logs to provide actionable insights. Retailers can improve product designs or create new offerings, boosting innovation and quality.
Generative AI Use Cases for the Retail Industry
Top six use cases of how brands, commerce and retailers can leverage generative AI in this transformative landscape:
1. Receive Tailored Product Recommendation
Modern consumers expect personalized and engaging content and deals from their preferred brands. Generative AI leverages customer data to curate personalized product recommendations and exclusive offers, creating tailor-made shopping experiences. By leveraging historical data, retailers can provide individualized discounts, enhancing sales and customer loyalty.
2. Optimize Inventory Management and Supply Chains
In response to recent challenges in retail supply chains, generative AI offers solutions. By analyzing sales data, this technology provides recommendations for inventory management. It assists in forecasting trends by analyzing historical data, consumer sentiment, and competitive information, enabling retailers to make informed decisions for ordering and manufacturing. This optimization aids in streamlining the supply chain and improving delivery efficiency.
Explore more about Generative AI for Supply Chain Management and its Use Cases
3. Enhance Product Page Descriptions and Images
The digital shelf requires more than good products; it demands optimized product description pages (PDP) for visibility. Generative AI can swiftly create, optimize, and improve product descriptions and copies. It contributes to improved rankings on the digital shelf by adding SEO keywords, optimizing images for PDP, and curating compelling product descriptions tailored to resonate with shoppers.
4. Monitor and Adjust Prices Effectively
Brands must remain vigilant in monitoring and comparing prices to adapt to shoppers' heightened price sensitivity. Generative AI in the Retail Industry enables brands and retailers to optimize costs rapidly by analyzing competitor price movements, demand patterns, and market trends. Real-time adjustments help avoid losing customers to competitors and maintain market competitiveness.
5. Develop Customer Service Chatbots
Generative AI facilitates the creation of chatbots capable of assisting customers with inquiries and troubleshooting. This technology enables retailers to enhance customer service, reduce the workload on human representatives, and improve overall customer satisfaction. Effective customer service contributes to increased brand loyalty.
Get to know more about Generative AI for Customer Service and its Use Case
6. Identify and Prevent Fraudulent Activities
Generative AI algorithms detect and prevent fraudulent activities, such as fake purchases or returns. This technology can also identify counterfeit products and unauthorized sellers using sophisticated algorithms. These capabilities help protect a brand's identity, preserve profit margins, and enhance customer trust.
Examples of GenAI in the Retail Industry
Despite its relatively recent introduction, Generative AI (GenAI) has quickly become a valuable retailer asset, offering numerous innovative applications. Here are some examples:
-
Hypermarket Chain Enhances Customer Experience - A global hypermarket leverages a GenAI-powered chatbot to assist shoppers in purchasing groceries tailored to recipes and dietary needs, using a friendly and conversational tone. On the backend, it utilizes GenAI to draft vendor proposals and analyze bid submissions.
-
Electronics Store Summarizes Customer Reviews - An online electronics retailer uses GenAI to generate concise summaries of customer reviews, enabling shoppers to quickly understand product feedback without reading through each review individually.
-
Apparel Store Simplifies Gift Buying - An online apparel and accessories retailer employs a GenAI chatbot to guide customers in selecting gifts. The bot gathers details like the recipient’s gender, size, hobbies, interests, and budget to recommend suitable options.
-
Superstore Optimizes Vendor Negotiations - A global superstore uses GenAI for small contract negotiations with vendors. Conventional analytics assess the market, while a GenAI chatbot interacts with vendors via online portals, streamlining communication and freeing procurement teams to focus on strategic deals.
-
Online Mall Supports Small Businesses - An online shopping platform that uses AI-driven analytics to help small retailers optimize pricing strategies. GenAI complements this by crafting product descriptions and personalized follow-up messages for interested customers.
Challenges in Leveraging Generative AI
1. Limited Understanding and Expertise
More understanding and expertise are among today's primary hurdles for generative AI. Numerous businesses encounter barriers in acquiring the requisite technical expertise to proficiently deploy and harness AI tools' capabilities, potentially limiting their innovation potential and competitiveness.
2. Data Quality and Bias Issue
Another notable challenge revolves around data quality and bias. Generative AI models rely heavily on extensive top-notch data to generate accurate and impactful outcomes. Should the data be skewed, incomplete, or inaccurate, the models may produce biased or erroneous results, threatening the business's reputation and trustworthiness.
Explore How Generative AI Can Improve Data Quality
3. Model Interpretability and Reliability Problem
The interpretability and reliability of generative AI models present a third challenge. Often complex and opaque, these models create difficulties for businesses in explaining their functioning and the reasons behind specific outputs. This poses a risk for companies needing to justify decisions or actions based on model outputs. Additionally, generative AI models may generate unexpected or inconsistent results, impacting the overall quality and usability of the generated content.
4. Regulation and Ethical Concerns
Generative AI raises ethical and regulatory concerns about privacy, security, authenticity, accountability, and fairness. Businesses must navigate potential risks and responsibilities associated with generative AI use, ensuring compliance with relevant laws and regulations. Alignment with organizational values and principles is crucial to preventing harm or offence to stakeholders.
- Know more about Generative AI for Insurance
- Explore further Generative AI for Energy Sector
- Deep dive into Generative AI for Supply Chain
Key Takeaways: Generative AI in Retail
- Market Growth: Projected 10.4% CAGR (2023-2028).
- Applications: Personalization, virtual stylists, catalog automation, demand forecasting, and loyalty programs.
- Efficiency: Automates tasks, improves catalog management, and optimizes supply chains.
- Personalization: Drives tailored recommendations, marketing, and pricing strategies.
- Customer Experience: Enhances support with AI chatbots and tools for faster resolutions.
- Fraud Prevention: Identifies and mitigates fraudulent activities.
- Real-World Use: Supports review summaries, gifting suggestions, vendor negotiations, and small businesses.
- Challenges: Faces hurdles like data bias, model reliability, and ethical concerns.
- Future Scope: Promises innovation, efficiency, and strategic benefits with responsible use.