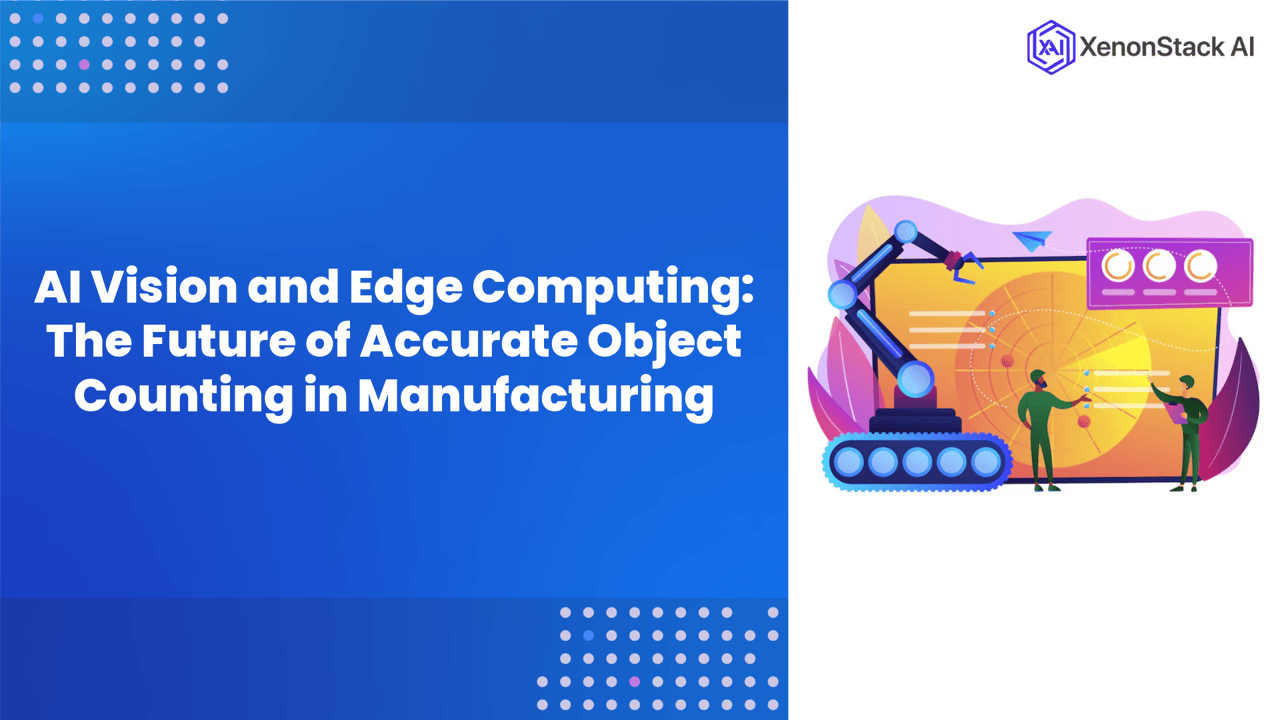
The manufacturing industry is undergoing a significant transformation driven by advancements in artificial intelligence (AI) and edge computing. Among the many processes being revolutionized, object counting stands out as a critical operation that directly impacts efficiency, accuracy, and cost-effectiveness. Whether it's:
-
Counting components on an assembly line
-
Tracking inventory in a warehouse
-
Ensuring the correct number of items in a package
Object counting is a fundamental task that manufacturers must perform with precision.
The Shift Towards Smart Object Counting in Manufacturing
Traditional methods of object counting, such as:
-
Manual counting
-
Mechanical sensors
are increasingly proving inadequate in the face of modern manufacturing demands. These methods are often:
-
Error-prone
-
Time-consuming
-
Unable to keep up with the speed and complexity of today's production lines
Enter AI vision and edge computing—two technologies that are redefining how manufacturers approach object counting.
To truly maximize the benefits of AI vision and edge computing in object counting, exploring the right edge computing platforms is essential. Discover the Top 5 Edge Computing Platforms in 2025 to take your manufacturing efficiency to the next level.
This blog delves deep into how AI vision and edge computing are transforming object counting in manufacturing. We will explore the limitations of traditional methods, the mechanics of AI vision, the role of edge computing, and the tangible benefits these technologies bring to the manufacturing floor. Additionally, we will examine real-world use cases, address implementation challenges, and look ahead to the future of these technologies in the manufacturing sector.
Why Accurate Object Counting is Crucial for Manufacturing Efficiency
Accurate object counting is a cornerstone of efficient manufacturing operations. It plays a pivotal role in several key areas:
Inventory Management
Accurate counting ensures that manufacturers maintain optimal stock levels. Overproduction can lead to excess inventory, tying up capital and storage space, while underproduction can result in stockouts, delaying orders, and disappointing customers.
Quality Control
In industries like automotive, electronics, and pharmaceuticals, missing or extra components can lead to defective products. Accurate counting ensures that each product meets quality standards, reducing the risk of recalls and reputational damage.
Production Efficiency
Counting objects accurately helps manufacturers identify bottlenecks and optimize workflows. For example, if a particular station on an assembly line is consistently short on components, it can cause delays downstream. Real-time counting allows for immediate corrective action.
Cost Reduction
Miscounts can lead to significant financial losses. For instance, overcounting can result in unnecessary reorders, while undercounting can lead to production halts. Accurate counting minimizes waste and optimizes resource allocation.
Compliance and Reporting
Many industries are subject to strict regulatory requirements. Accurate counting ensures compliance with these regulations, avoiding fines and legal issues. It also facilitates accurate reporting, which is essential for strategic decision-making.
Limitations of Traditional Object Counting and the Need for Change
Before the advent of AI and edge computing, manufacturers relied on several traditional methods for object counting. While these methods served their purpose, they come with significant limitations:
Manual Counting
-
Simple and requires no specialized equipment.
-
Extremely time-consuming, prone to human error, and not scalable for large-scale operations.
Mechanical Sensors
-
Automated and faster than manual counting.
-
Limited in their ability to distinguish between overlapping or irregularly shaped objects. They also require precise alignment and are often inflexible.
Barcode and RFID Scanning
-
Relatively accurate and can track individual items.
-
Requires manual intervention, making it unsuitable for high-speed production lines. Additionally, barcodes can be damaged, and RFID tags can be expensive
Conveyor-Based Counters
-
Automated and can handle high volumes.
-
Limited adaptability to different product shapes and sizes. They also struggle with items that are not uniformly spaced.
These limitations create inefficiencies that can lead to increased labor costs, operational delays, and reduced overall productivity.
AI Vision and Edge Computing: Transforming Object Counting in Manufacturing
The integration of AI vision and edge computing is revolutionizing object counting in manufacturing. These technologies address the shortcomings of traditional methods by offering:
High-Speed, Automated Counting: AI vision systems can process images and videos at incredible speeds, making them ideal for high-speed production lines. Real-Time Processing with Minimal Latency: Edge computing ensures that data is processed locally, reducing latency and enabling real-time decision-making. Improved Accuracy Through Deep Learning: AI models can be trained to recognize and count objects with a high degree of accuracy, even in complex environments. Adaptability to Diverse Manufacturing Environments: AI vision systems can be easily adapted to different product types, shapes, and sizes, making them versatile tools for various industries.
How AI Vision Streamlines Object Counting in Modern Manufacturing
AI vision systems leverage deep learning and computer vision to analyze images or videos and count objects with precision. The process involves several key steps:
Image Acquisition
High-resolution cameras capture images or video footage from the production line. These cameras are strategically placed to ensure optimal coverage of the objects being counted.
Preprocessing
The captured images are preprocessed to enhance their quality. This may involve filtering out noise, adjusting brightness and contrast, and resizing the images to a standard format.
Object Detection
AI models, often based on convolutional neural networks (CNNs), analyze the preprocessed images to detect and classify objects. These models are trained on large datasets to recognize specific objects, even in cluttered or overlapping scenarios.
Counting Algorithm
Once objects are detected, a counting algorithm is applied to tally them. This algorithm can differentiate between objects that are close together or partially obscured, ensuring accurate counts.
Data Integration
The counted data is integrated into the manufacturing system in real-time. This allows for immediate monitoring and decision-making, such as triggering alerts if a count is off or adjusting production parameters.
Enhancing AI Vision with Edge Computing for Real-Time Solutions
Edge computing plays a crucial role in enhancing the capabilities of AI vision systems. By processing data closer to the source, edge computing offers several advantages:
-
Real-Time Processing: Edge devices process data locally, eliminating the need to send data to a centralized cloud server. This reduces latency and enables real-time object detection and counting.
-
Bandwidth Efficiency: By processing data at the edge, manufacturers can reduce the amount of data that needs to be transmitted to the cloud. This not only saves bandwidth but also reduces costs associated with data transfer.
-
Scalability: Edge computing allows manufacturers to deploy AI vision systems across multiple production facilities without the need for extensive cloud infrastructure. This makes it easier to scale operations as needed.
Key Benefits of AI Vision and Edge Computing in Manufacturing
The adoption of AI vision and edge computing provides manufacturers with several tangible benefits:
Enhanced Accuracy
AI models can count objects with a high degree of accuracy, reducing errors and improving overall product quality.
Increased Speed
Automated counting systems can keep up with high-speed production lines, ensuring that operations run smoothly without delays.
Operational Efficiency
Real-time monitoring allows manufacturers to detect inefficiencies early and take corrective action, improving overall operational efficiency.
Reduced Costs
By minimizing reliance on manual labor and avoiding losses due to miscounts, manufacturers can significantly reduce costs.
Scalability
AI vision systems can be easily deployed across different production facilities, making it easier to scale operations as needed.
Continuous Learning
AI models can be continuously trained and improved, ensuring that they remain effective even as manufacturing conditions change.
Use Cases of AI Vision and Edge Computing for Object Counting
-
Automotive Industry: AI-driven cameras count components on assembly lines, ensuring that each vehicle has the correct number of parts. This reduces the risk of defects and improves overall product quality.
-
Food & Beverage Industry: Edge AI systems detect and count packaged food items, ensuring accuracy before dispatch. This is particularly important in industries where regulatory compliance is critical.
-
Pharmaceutical Industry: AI vision ensures the correct number of tablets in blister packs, reducing the risk of compliance violations and improving patient safety.
-
Logistics & Warehousing: Edge AI systems count goods in transit and storage, reducing losses and optimizing inventory management. This is particularly important in industries where inventory accuracy is critical.
-
Electronics Manufacturing: Real-time component counting helps prevent assembly errors in circuit board production, improving overall product quality and reducing the risk of defects.
Overcoming Implementation Challenges with AI and Edge Computing
Despite its many advantages, implementing AI vision and edge computing comes with several challenges:
-
Initial Investment: The setup costs for AI-enabled cameras and edge devices can be high. However, the long-term benefits often outweigh the initial investment.
-
Model Training & Adaptation: AI models need to be fine-tuned to handle different product shapes, sizes, and lighting conditions. This requires a significant amount of training data and expertise.
-
Integration with Legacy Systems: Ensuring compatibility with existing manufacturing infrastructure can be challenging. Manufacturers may need to invest in additional hardware or software to facilitate integration.
-
Data Privacy & Security: Protecting sensitive manufacturing data processed at the edge is critical. Manufacturers must implement robust security measures to prevent data breaches.
-
Hardware Maintenance: Keeping edge devices and cameras in optimal condition is essential for uninterrupted performance. Regular maintenance and updates are required to ensure long-term reliability
The Future of AI Vision and Edge Computing in Manufacturing
The future of AI vision and edge computing in manufacturing looks promising, with several advancements on the horizon:
Edge AI Optimization
More powerful edge processors will improve the speed and accuracy of AI vision systems, making them even more effective.
Self-Learning AI Models
Adaptive AI models that fine-tune themselves based on new manufacturing conditions will become more common, reducing the need for manual intervention.
5G Integration
The rollout of 5G networks will enable faster data transfer, enhancing real-time object detection and counting capabilities.
Collaborative Robotics (Cobots)
AI-driven robots will work alongside human workers, assisting in object handling and quality checks, further improving efficiency.
Predictive Analytics
AI vision systems will be integrated with predictive maintenance tools, allowing manufacturers to foresee equipment failures and take preventive action.
Driving Productivity and Efficiency with AI Vision and Edge Computing
AI vision and edge computing are changing object counting in manufacturing, offering unparalleled accuracy, speed, and efficiency. By automating the counting process, manufacturers can improve productivity, reduce costs, and maintain high-quality standards. As technology continues to evolve, AI-driven vision systems will become even more sophisticated, further transforming the manufacturing landscape.
Manufacturers looking to stay ahead in the competitive market should embrace AI vision and edge computing for optimized object counting and smarter production processes. The future of manufacturing is here, and AI and edge computing power it.
Next Steps in Implementing AI Vision and Edge Computing
Talk to our experts about implementing AI vision and edge computing systems. How industries and departments use object counting and decision intelligence to become efficiency-centric. Utilize AI to automate and optimize manufacturing operations, improving accuracy and responsiveness.