What is Human Pose Estimation?
Human pose estimation, therefore, can be defined simply as the task by which machines try to identify and locate specific points on human bodies from images or videos. This relates to the displacement of key points—head, shoulders, elbows, and knees—in two dimensions (2D) or three dimensions (3D). The consequences of accurately estimating human pose are far-reaching, and hence, it is crucial since machines need to learn the basis of postures and motions.
For instance, the area of interest is healthcare. Real-time accurate pose estimation can help to assess a patient’s mobility and physical state. With this technology, designers can quantify movement in individuals and can recognize any abnormality to normal movement and the fundamental jobs of the body that are likely to be an indication of a health complication such as falls and poor mobility. Likewise, in sports, pose estimation will be helpful for the coaches to analyze how the athlete is moving and how it can be improved or prevented from causing harm.
The Role of Action Recognition
However, when the human poses are accurately estimated, one is left with the action recognition problem. This process includes translating the actions done by particular key points to set certain activities like walking, running, or jumping and even detailed movements like waving or reaching. A major issue here arises from the very fact that human motion is hardly ever consistent in the last degree; minor differences in the way in which a movement is performed may be interpreted in drastically different ways- this is why this field is very engaging yet very complicated at the same time.
As demonstrated before, action recognition has imperative implications for almost every field of study. In the sphere of public safety, it might help improve the surveillance system by indicating that someone is behaving uncomfortably, having a seizure, or starting to act threateningly. In smart homes, hand gestures can also help control various gadgets, making the control system more natural.
Understanding the Problem
Safety Monitoring
-
Accident Detection: Falling or any form of accident can be recognized automatically, leading to immediate alerts. Hence, response to the incidences can also be fast, thus preventing more from passing away.
Behavior Analysis
Performance Enhancement
User Interaction
-
Enhanced Engagement: Bringing increased interactivity in app-generated experiences, including educational, training, or entertainment environments.
Traffic and Pedestrian Safety
Crafting the Solution
A Step-by-Step Approach
1. From Video Frame to 2D Human Keypoints
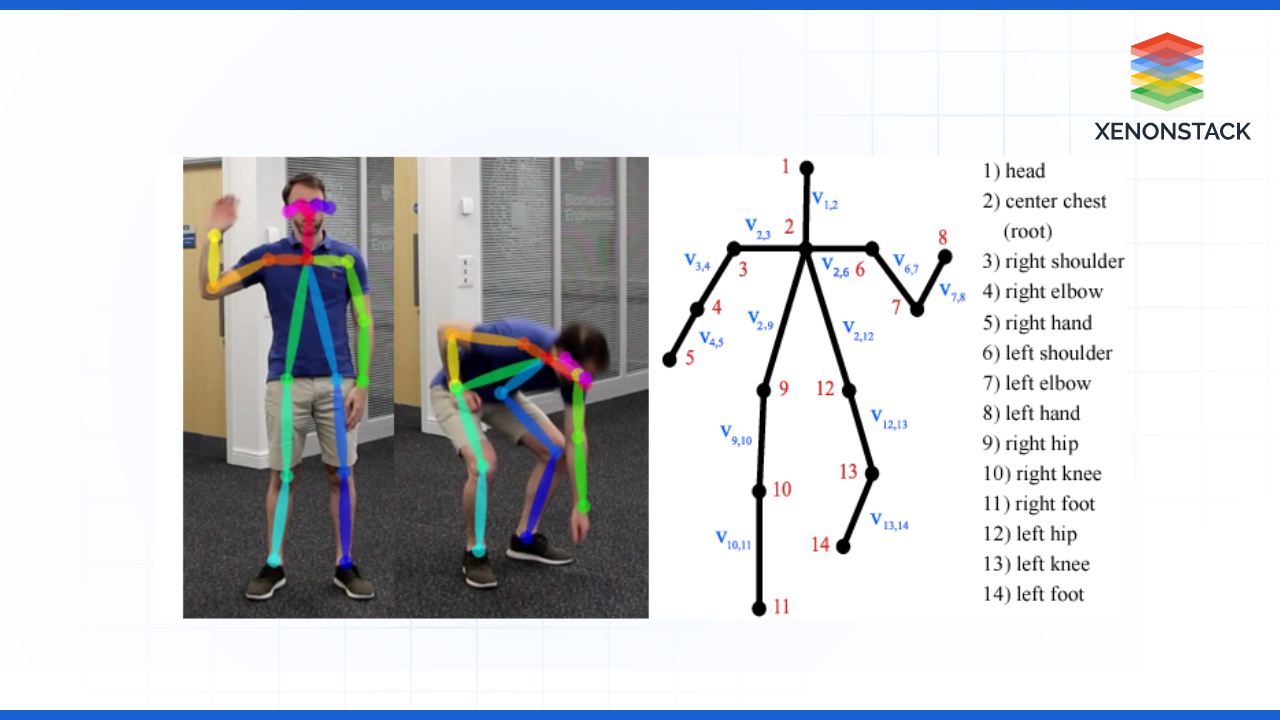
Figure – Mapping of Human Keypoints
Overview: The first phase of human pose estimation includes identifying components of a human body in each frame of the video signaled by bright points organized in correspondence with the skeleton topology. It is a process of converting video space into the spatial coordinates of human posture.
Key Steps:
-
Preprocessing: Products are further proposed to perform a standard normalization of the input images to enhance the model’s outcome. As part of data preprocessing, it is possible to scale, transform colors, or apply other standard operations to a dataset to make it more adaptable.
-
Model Selection: Use an optimal model for 2D pose detection, maybe a CNN model enhanced for keypoint detection. To maintain high accuracy for this model, a data set should be developed containing the annotated key points, such as COCO or MPII, etc.
-
Keypoint Detection: Perform a model on each frame to detect and localize 2D key points that signify the human skeleton. Each key point will be represented by the coordinates of its position in the frame, coordinates (x, y).
2. Mapping 2D to 3D Human Keypoints
Overview: As mentioned earlier, 2D key points are fundamental, and riding them into the third dimension improves the perception of human motion in space.
Key Steps:
-
Depth Estimation: One method employs other methods, like triangulation or training machine learning algorithms, which use information produced by 2D key points to approximate depth and devise a 3D map for each.
3. Identifying Human Actions
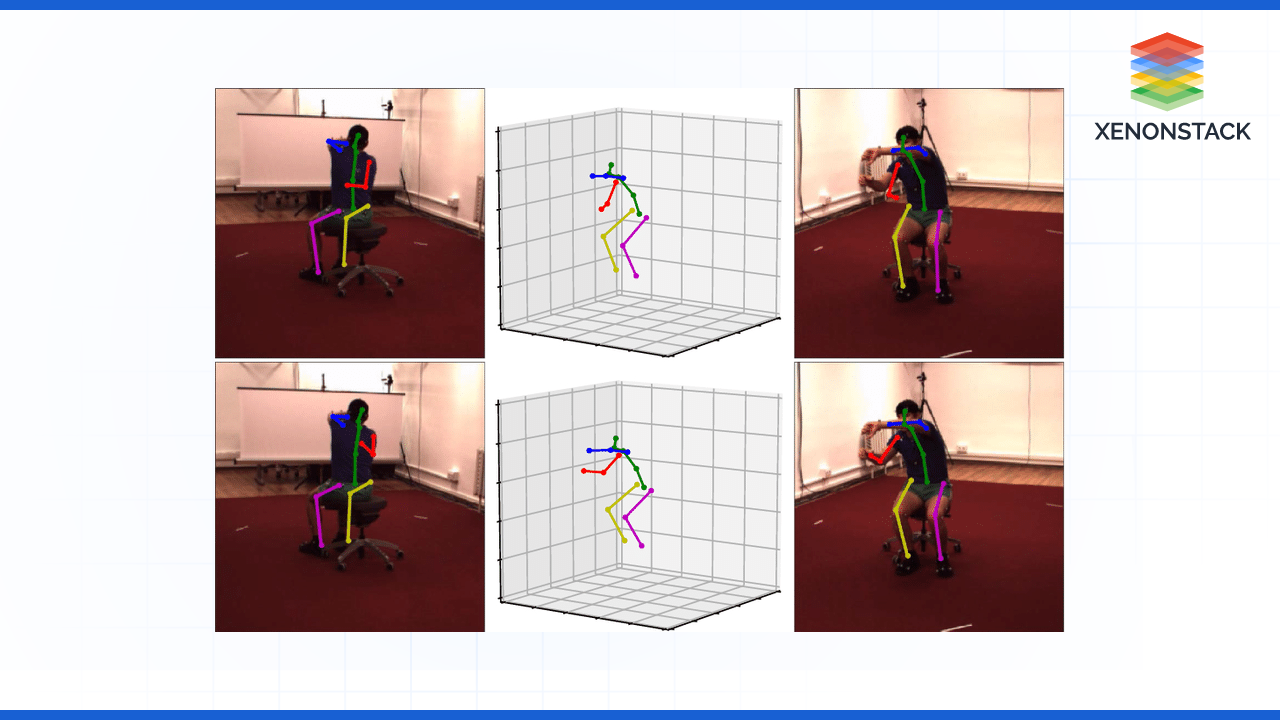
Figure - Mapping 2D to 3D
Overview: When both 2D and 3D key points have been determined, the following step is to study the movements in order to identify an individual's specific action or behavior.
Key Steps:
-
Heuristic Analysis: Describe how simple heuristic methods can be applied to monitor changes in the keypoint position and velocity. For instance, where the height of the head or distance of the limbs is detected, it is possible to deduce if the person is walking, jumping, or falling.
4. Real-Time Implementation
Overview: Practical systems require the system to work in real-time in a fashion where it provides feedback and responses in real-time.
Key Steps:
Testing and Validation
Activity Monitoring: The camera recorded video samples of various situations to note various actions, which included:
-
Riding a Scooter: To assess the model's deeper capacity by making its components and algorithms foresee and recognize not only the very typical and average motions compared to those closely associated with the device, one may sit on a scooter and observe how well the model responds to more dynamic and unique movements.
Performance Evaluation
Feedback and Iteration: The validation information proved quite useful in identifying aspects that were well done and those that required some improvement. The observations from the activity monitoring applied to tuning lead to a higher accuracy of detections in algorithms as well as an enriched user experience.