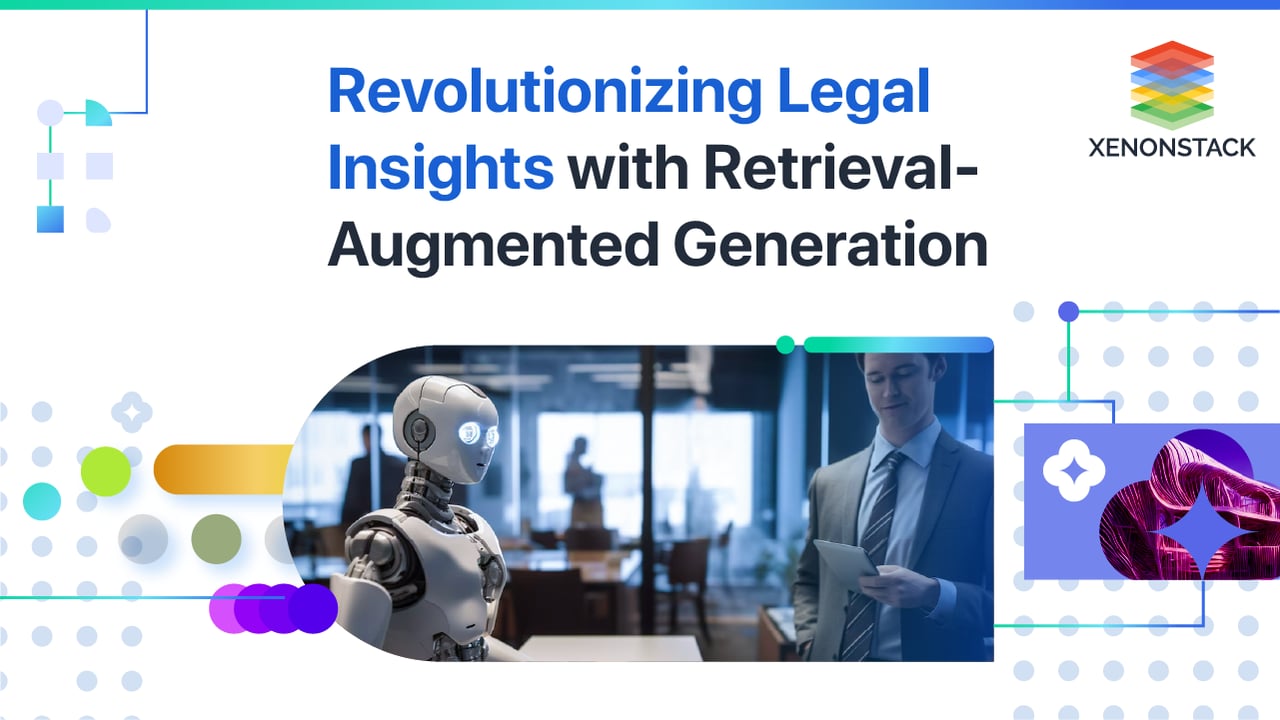
Overview
Legal research is notoriously difficult, involving law libraries and legal experts. It is both time-consuming and complicated, which can frustrate a lawyer or law student, especially if the practitioner does not have access to the required resources or expertise.
Legal work involves a wide range of tasks and challenges. Lawyers and legal professionals are responsible for various activities such as legal research, document review, contract drafting, case management, and client communication. These tasks often require significant time and effort, leading to high costs and potential human errors. Additionally, the legal industry faces issues such as limited access to justice, overwhelming caseloads, and the need for quick and accurate decision-making.
Challenges with the Current Process
-
Volume of Information: Legal research involves sifting through a vast amount of information presented in various formats, including case law, statutes, regulations, and other documents.
-
Variability: Information can vary significantly in its presentation, making it challenging to navigate and interpret effectively.
-
Jurisdictional Limitations: Legal research can be restricted by jurisdiction, business practice, and the size of the jurisdiction, adding complexity to the process.
-
Dynamic Nature of Laws: Laws are subject to frequent changes through new legislation, court decisions, or amendments, necessitating continuous updates and awareness among legal professionals.
-
Risk of Inaccuracies: Failure to consider recent legal changes can result in inaccurate analysis and potentially adverse legal outcomes for clients.
-
Electronic Discovery: Identifying, collecting, and analyzing electronic data is complex and time-consuming, necessitating technical expertise and efficient tools.
-
Client Confidentiality: Maintaining client confidentiality and adhering to ethical guidelines is crucial. Legal professionals must handle sensitive information securely throughout the research and analysis process.
Solution: Innovating Legal Practices
Introducin AI Agent, an advanced solution designed to assist legal professionals in tackling complex challenges. By leveraging Retrieval-Augmented Generation (RAG) and Large Language Models (LLMs), Legal Gen AI provides comprehensive support, enhancing efficiency and accuracy in legal research and decision-making. In addressing the challenge of accessing legal documents and extracting relevant information, a Retrieval-Augmented Generation (RAG) application offers a comprehensive solution. This innovative approach combines the power of large language models (LLMs) with retrieval mechanisms, allowing users to efficiently access and utilize external knowledge sources.
Approach: Strategic Implementation
Retrieval-Augmented Generation (RAG)
-
Optimization process for large language models (LLMs) where they reference external authoritative knowledge bases before generating responses.
-
Enhances LLM capabilities by extending them to specific domains or internal organizational knowledge bases.
-
No need for retraining the model, making it a cost-effective solution.
Identify Relevant Knowledge Sources
-
Determine authoritative legal databases, case law repositories, statutes, regulations, and other sources relevant to the legal domain.
Develop Retrieval Mechanisms
-
Implement algorithms to retrieve information from identified knowledge sources based on user queries or prompts.
-
Ensure retrieval mechanisms are efficient and capable of handling large volumes of legal data.
Integrating with Large Language Models (LLMs):
-
Incorporating the retrieval mechanisms into the RAG framework, enabling LLMs to access and utilize retrieved legal information.
-
Ensuring seamless integration between the retrieval process and LLMs' generation capabilities.
Customize for Legal Domain
- Tailor the RAG application to suit the specific needs and nuances of the legal domain, including jurisdictional variations and legal terminology.
Iterative Improvement and Validation:
-
Continuously refine and enhance the RAG application through iterative testing and validation.
-
Request feedback from legal professionals to identify areas for improvement and optimization.
Comprehensive Architecture for Legal AI
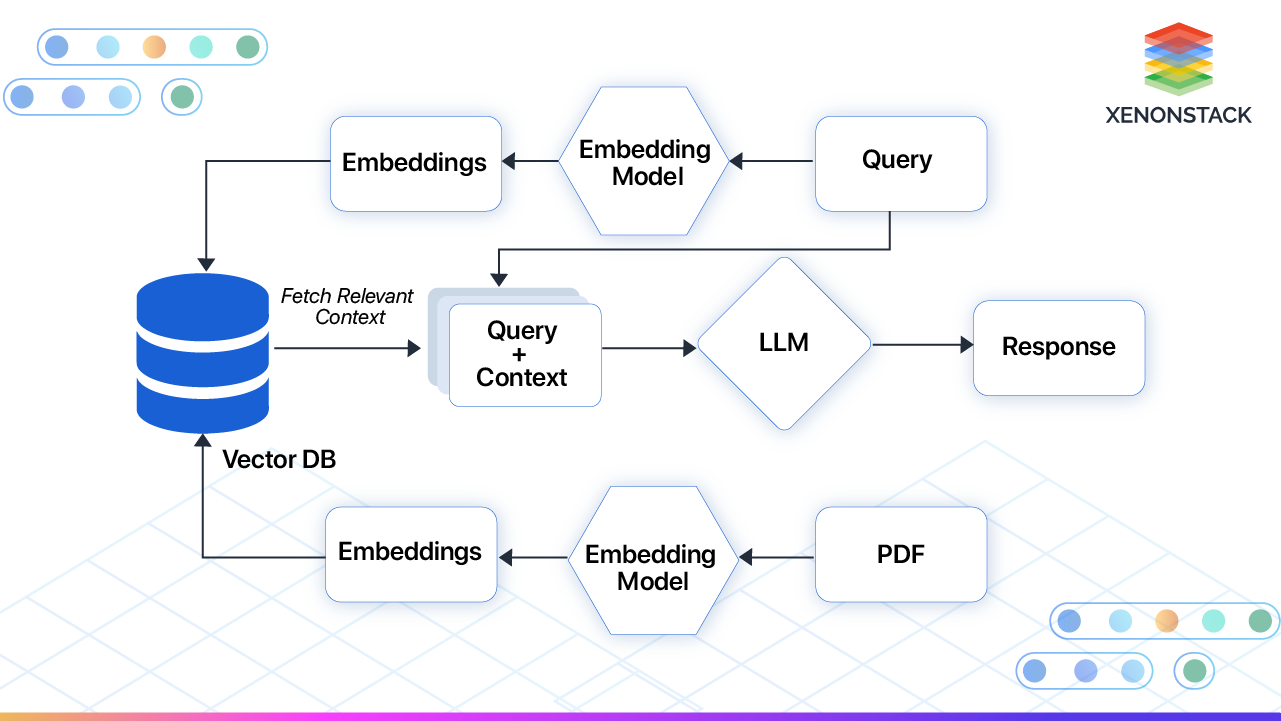
Query Processor (Query)
The Query Processor component receives user queries or prompts from the UI and preprocesses them before passing them to the retrieval and generation modules. This may involve tokenization, parsing, and other linguistic preprocessing tasks.
Retrieval Module
The Retrieval Module retrieves relevant information from external knowledge sources based on the user's query. It interfaces with databases, APIs, or other data repositories containing legal documents, case law, statutes, regulations, and other relevant sources.
Knowledge Base (PDF)
The Knowledge Base consists of authoritative sources of legal information, including databases, repositories, and libraries containing legal documents and resources. This may include both structured and unstructured data sources.
Embeddings
Embeddings encapsulate the semantic meaning of words and phrases by representing them as points in a high-dimensional vector space. Words with similar meanings are mapped to nearby points in this space, enabling the model to comprehend the context and semantics of textual input.
Retrieval Mechanisms
Retrieval Mechanisms are algorithms and techniques used to search, retrieve, and filter information from the Knowledge Base based on the user query. This may involve keyword-based search, semantic matching, document ranking, and other retrieval strategies.
Large Language Model (LLM)
The large language model (LLM) forms the core of the RAG application and generates responses based on the retrieved information. It utilizes pre-trained language models with billions of parameters to generate text output.
Vector DB
Vector DB stores vector embeddings as key-value pairs, where each key represents an identifier or unique identifier associated with a vector, and the corresponding value represents the vector embedding itself. This enables efficient storage and retrieval of vectors.
Understanding AI's Legal Impact
Combining Retrieval and Generation
-
Hybrid Model: RAG leverages both retrieval-based and generative models to enhance the accuracy and relevance of responses. This hybrid approach ensures that the information is not only relevant but also contextually appropriate.
-
Contextual Relevance: By retrieving relevant documents or excerpts first, the generative model can produce responses that are grounded in actual legal texts, leading to more precise and reliable outputs.
Efficiency in Handling Legal Data
-
Rapid Data Processing: RAG systems can process vast amounts of legal data quickly, including statutes, case law, legal opinions, and regulatory texts.
-
Automated Research: This technology automates the traditionally time-consuming process of legal research, freeing lawyers to focus on more strategic tasks.
Case Law Analysis
-
Extracting and summarizing relevant case laws and precedents that can support legal arguments or inform case strategies.
Regulatory Compliance
-
Ensuring that businesses adhere to relevant regulations by continuously monitoring and interpreting changes in legal requirements.
Advantages of Implementation
1. Efficiency and Time Savings: Automates labor-intensive tasks, allowing legal professionals to focus on higher-value activities.
-
Time Saved: After adopting the RAG application, the average time spent on legal research and document retrieval was reduced by 70%.
-
Increased Productivity: Legal professionals can increase their productivity by up to 50% due to faster access to relevant legal information.
2. Accuracy and Consistency: Reduces the risk of human error and ensures consistent application of legal principles and standards.
-
The RAG application can contribute to a 90% decrease in errors or inaccuracies in legal documents and analyses
3. Enhanced Decision-Making: Provides comprehensive and up-to-date information, enabling more informed legal decisions.
4. Cost Reduction: Reduces the reliance on extensive manual labor, thereby lowering operational costs.
-
The ROI for the RAG application can be up to 3x the decrease in cost usage, which would include manpower, time taken, etc.
5. Tailored Solutions: RAG can be customized to cater to specific legal practices, whether for small law firms or large corporate legal departments.
6. Legacy Data Utilization: This method utilizes historical legal data, ensuring that valuable insights and precedents are not lost.
Concluding the RAG Revolution
The implementation of Retrieval-Augmented Generation (RAG) technology in legal work represents a transformative advancement, significantly enhancing efficiency, accuracy, and overall effectiveness in the legal domain. This project demonstrates that RAG systems drastically reduce the time required for legal research, document review, and case preparation by automating labor-intensive tasks, allowing legal professionals to focus on strategic activities. The hybrid approach of combining retrieval with generation ensures accurate, contextually relevant information, reducing human error and maintaining consistency in legal application.
However, challenges such as ensuring data privacy and security, addressing biases, and achieving seamless integration with existing systems must be considered. Overall, the project underscores the profound potential of RAG in revolutionizing legal work, delivering significant benefits in efficiency, accuracy, cost reduction, and client satisfaction.
Thanks for submitting the form.